Wheelchair free hands navigation using robust DWT_AR features extraction method with Muscle Brain signals
IEEE ACCESS(2020)
Abstract
Researchers try to help disabled people by introducing some innovative applications to support and assess their life. The Brain-Computer Interface (BCI) application that covers both hardware and software models, is considered in this work. BCI is implemented based on brain signals to be converted to commands. To increase the number of commands, non-brain source signals are used, such as eye-blinking, teeth clenching, jaw squeezing, and other movements. This paper introduced a low dimensions robust method to detect the eye-blinks and jaw squeezing; so that the method can be applied to drive a wheelchair by using five commands. Our approach is used Discrete Wavelet Transform with Autoregressive to extract the signal & x2019;s features. These features are classified by using a linear Support Vector Machine (SVM) classifier. The present method detects every testing sample using a small training set to test and drive a powered wheelchair. The proposed method is fully implemented practically based on binary-coded commands.
MoreTranslated text
Key words
Autoregressive,brain-computer interface,coded commands,discrete wavelet transform,driving a wheelchair,electroencephalography,eye-blinks,jaw squeeze,muscle brain signals,robust features extraction method,signals detection
AI Read Science
Must-Reading Tree
Example
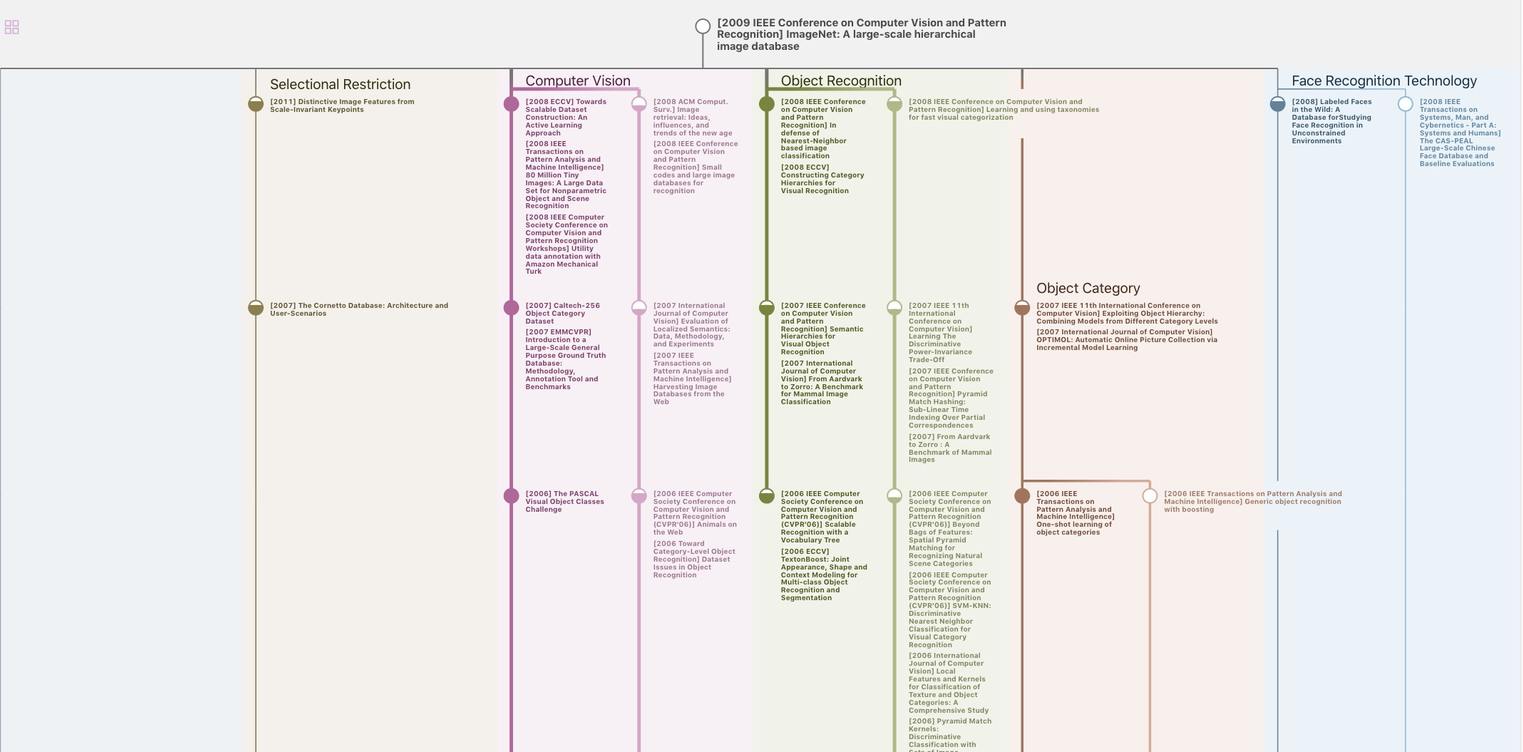
Generate MRT to find the research sequence of this paper
Chat Paper
Summary is being generated by the instructions you defined