Cancer-Related Gene Signature Selection Based On Boosted Regression For Multilayer Perceptron
IEEE ACCESS(2020)
摘要
Gene expression profiling is a useful technique for analyzing cellular function, and gene expression profiles are widely studied in human cancer research. Gene expression data usually consist of a very large number of features and a relatively small number of samples, and extracting a small number of important features from these data is a major challenge of gene expression-based analysis in cancer research. In this paper, we propose an embedded feature selection algorithm using boosted linear regression-based feature selection. The boosting technique is applied to derive the ensemble feature selector and improve the performance of linear regression-based feature selection. The proposed feature selection algorithm, called boosted regression-based feature selection for the multilayer perceptron (BREG-MLP), repeats the boosted feature selection process to extract the smallest feature subset while maintaining good classification performance. We apply the proposed BREG-MLP to some human cancer-related gene expression data sets for the purpose of extracting important features, and we confirm that BREG-MLP offers improved performance compared to single regression-based feature selection methods.
更多查看译文
关键词
Boosting, feature selection, linear regression, gene expression profile
AI 理解论文
溯源树
样例
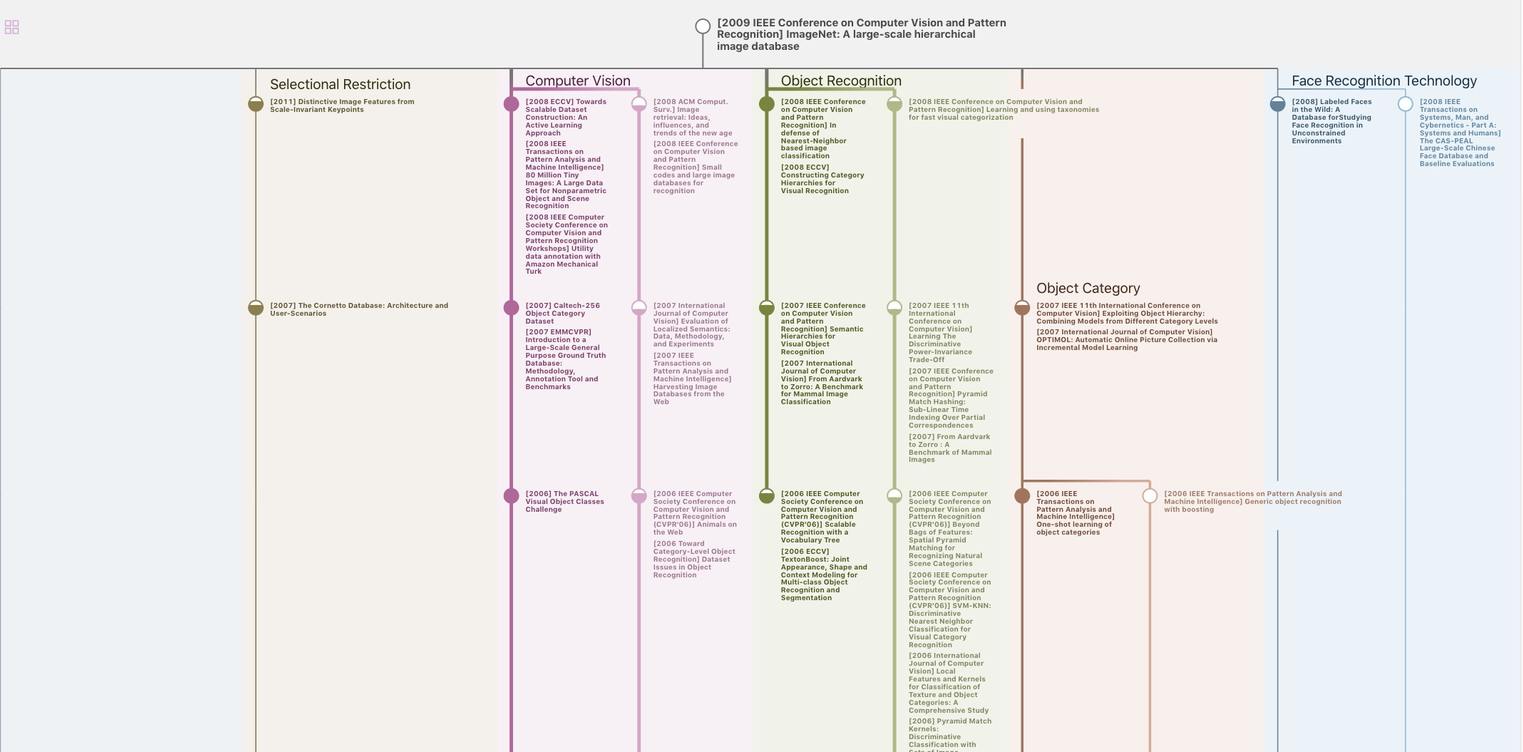
生成溯源树,研究论文发展脉络
Chat Paper
正在生成论文摘要