Multiple-Instance Learning Approach Via Bayesian Extreme Learning Machine
IEEE ACCESS(2020)
摘要
Multiple-instance learning (MIL) can solve supervised learning tasks, where only a bag of multiple instances is labeled, instead of a single instance. It is considerably important to develop effective and efficient MIL algorithms, because real-world datasets usually contain large instances. Known for its good generalization performance, MIL based on extreme learning machines (ELMx2013;MIL) has proven to be more efficient than several typical MIL classification methods. ELMx2013;MIL selects the most qualified instances from each bag through a single hidden layer feedforward network (SLFN) and trains modified ELM models to update the output weights. This learning approach often performs susceptible to the number of hidden nodes and can easily suffer from over-fitting problem. Using Bayesian inferences, this study introduces a Bayesian ELM (BELM)-based MIL algorithm (BELMx2013;MIL) to address MIL classification problems. First, weight self-learning method based on a Bayesian network is applied to determine the weights of instance features. The most qualified instances are then selected from each bag to represent the bag. Second, BELM can improve the classification model via regularization of automatic estimations to reduce possible over-fitting during the calibration process. Experiments and comparisons are conducted with several competing algorithms on Musk datasets, images datasets, and inductive logic programming datasets. Superior classification accuracy and performance are demonstrated by BELMx2013;MIL.
更多查看译文
关键词
Multiple-instance learning, Bayesian extreme learning machine, instance selection, classification
AI 理解论文
溯源树
样例
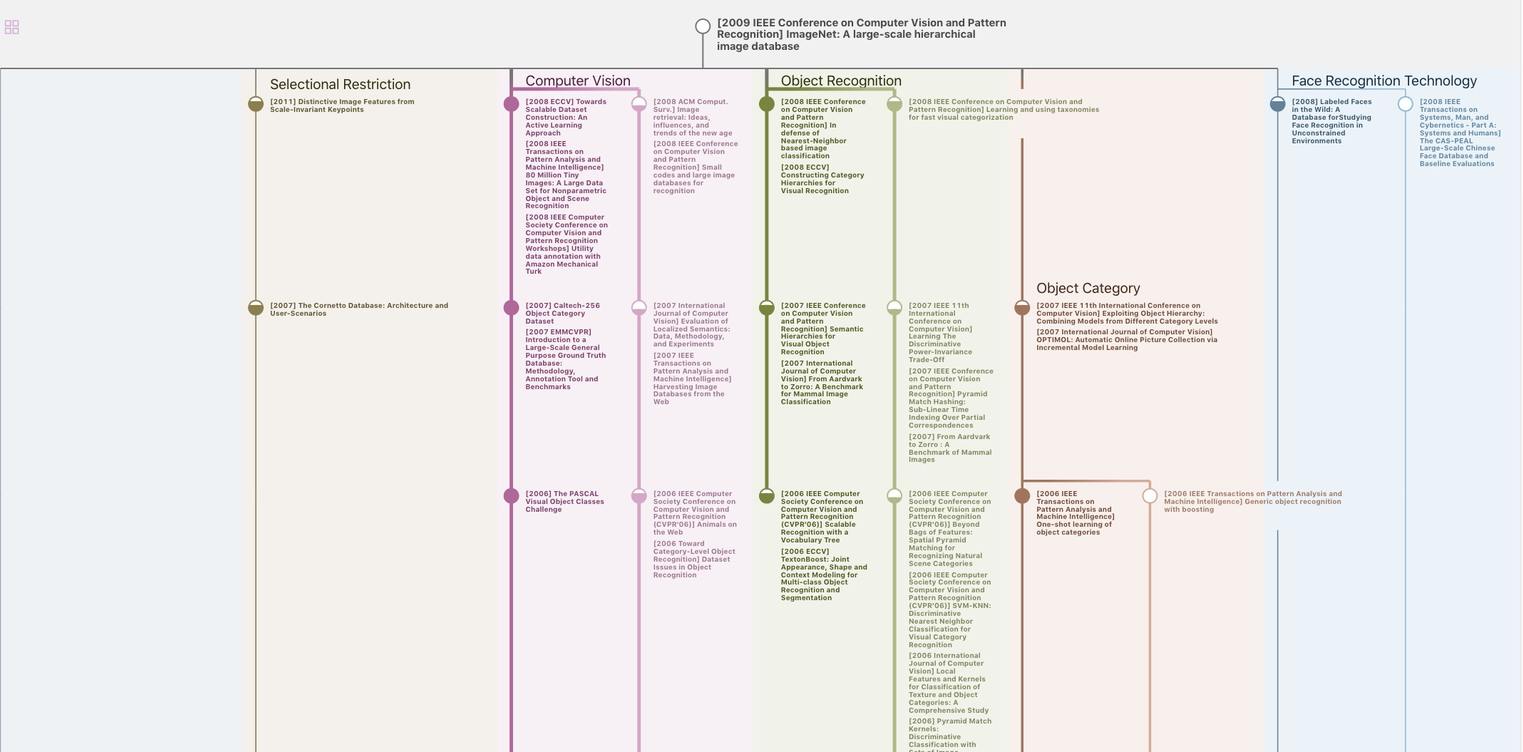
生成溯源树,研究论文发展脉络
Chat Paper
正在生成论文摘要