Machine Learning Aided Diagnosis of Diseases without Clinical Gold Standard: A New Score for Laryngopharyngeal Reflux Disease based on pH Monitoring
IEEE ACCESS(2020)
Abstract
Objective: Laryngopharyngeal Reflux Disease (LPRD) is prevalent and has a range of symptoms. However, diagnosing LPRD is difficult because of the lack of specific symptoms or clinical gold standard. An objective and reliable test, which does not rely on a perfect clinical gold standard, is required in clinical practice. Methods: 60 normal volunteers and 74 confirmed Laryngopharyngeal Reflux (LPR) patients were labelled based on the combined consideration of the reflux symptom index, reflux finding score, 24h oropharyngeal pH monitoring and results of anti-reflux treatment. 72 candidate features were extracted from pH recordings and the most efficient feature combination was detected using a stepwise wrapper method. The labelled data were combined with 1552 unlabelled data for feature selection and model training using semi-supervised learning. A latent class model method was used to assess the proposed model based on 64 additional validation data and an imperfect clinical reference test. Results: A new score (named W score), which significantly improved the sensitivity of the LPRD test (82.67 & x0025; vs. 24.09 & x0025;) and has a relatively high specificity (80.19 & x0025;), was proposed. W score concurs with the complicated clinical test. Conclusion: W score which significantly improves LPRD diagnostic efficiency, can aid the clinical diagnosis of LPRD. W score provides an objective, efficient, and reliable indicator for the application of anti-reflux treatment in clinical practice. Significance: More LPRD patients can benefit from anti-reflux treatment in clinical practice and fewer patients may suffer from, for example, the side effects of unnecessary long-term Proton Pump Inhibitors (PPI) treatments.
MoreTranslated text
Key words
Computer aided diagnosis,LPRD,pH monitoring,Ryan score,machine learning
AI Read Science
Must-Reading Tree
Example
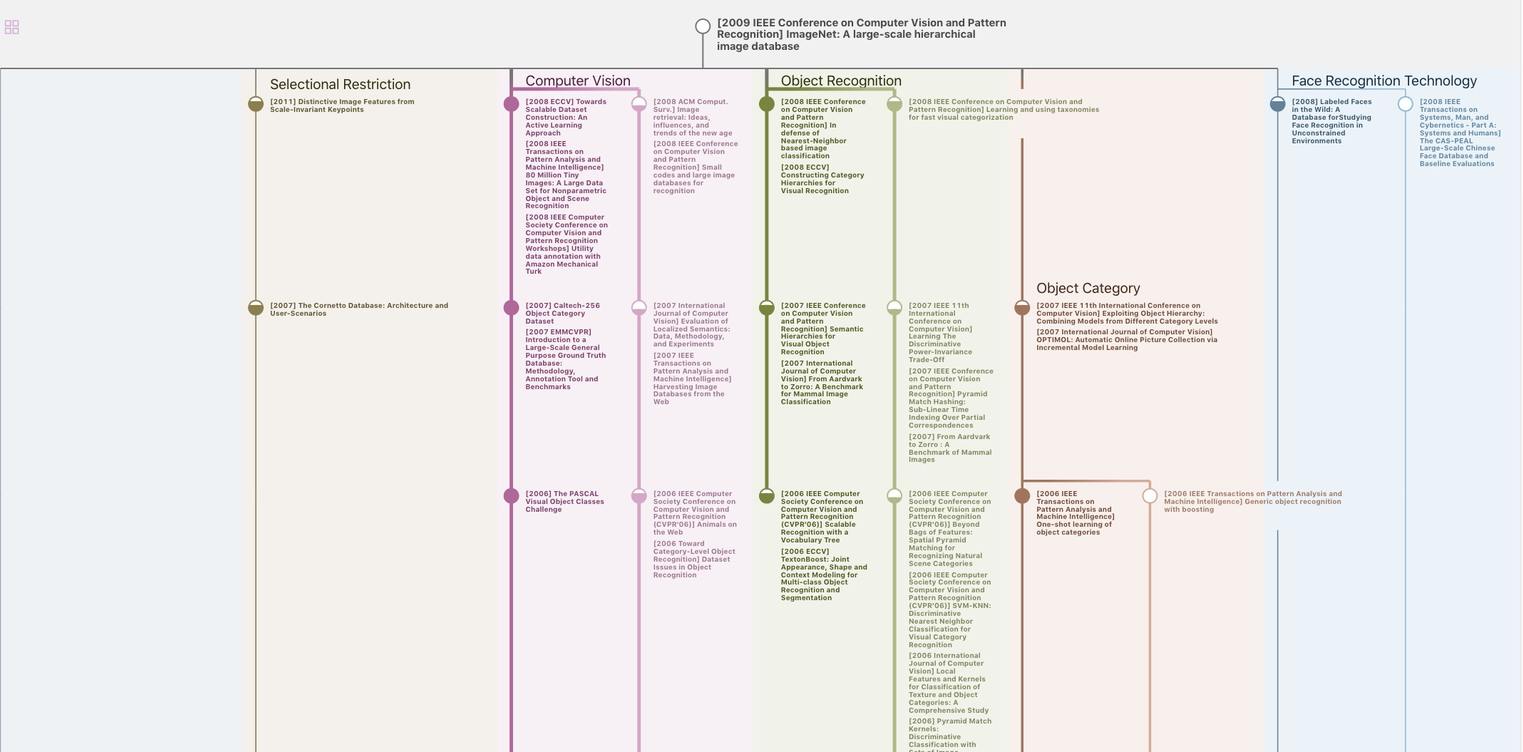
Generate MRT to find the research sequence of this paper
Chat Paper
Summary is being generated by the instructions you defined