Hierarchical Long Short-Term Memory Network for Cyberattack Detection
IEEE ACCESS(2020)
摘要
With the continuous development of network technology, cyberattack detection mechanisms play a vital role in ensuring the security of computers and network systems. However, with the rapid growth of network traffic, traditional intrusion detection systems (IDSs) are far from being able to quickly and accurately identify complex and diverse network attacks, especially those related to low-frequency attacks. To enhance the overall security of the Internet, an IDS based on hierarchical long short-term memory (HLSTM) networks is proposed. With the introduction of HLSTM, the network can learn across multiple levels of temporal hierarchy over complex network traffic sequences. The system is evaluated on the well-known benchmark data set NSL-KDD for comparison with other existing methods. The experimental results demonstrate that compared with existing start-of-the-art methods, our system has better detection performance for different types of cyberattacks. In addition, the low-frequency network attack types have higher classification accuracy and a lower false detection rate.
更多查看译文
关键词
Intrusion detection,Cyberattack,Training,Deep learning,Data models,Data preprocessing,HLSTM,cyberattack detection,NSL-KDD data set,intrusion detection system
AI 理解论文
溯源树
样例
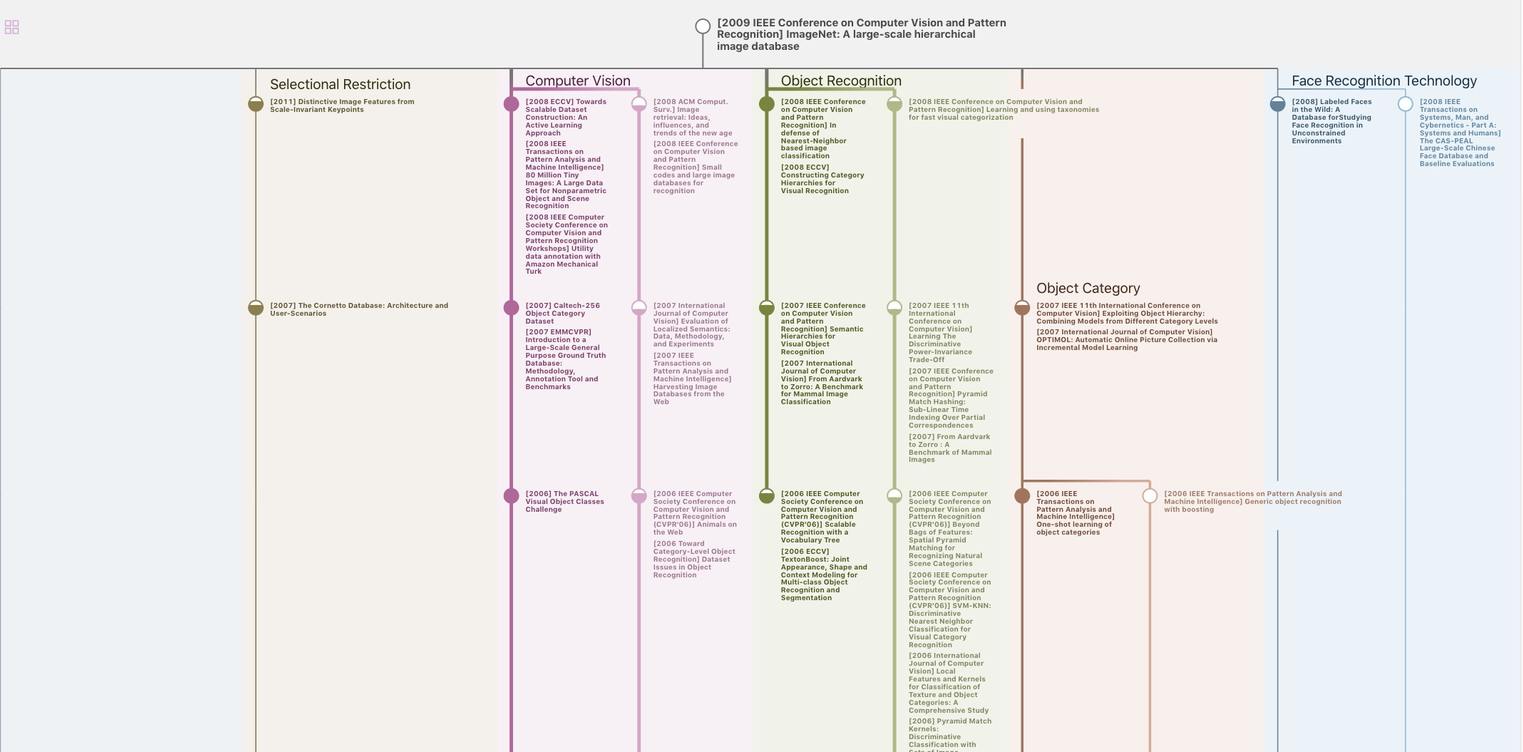
生成溯源树,研究论文发展脉络
Chat Paper
正在生成论文摘要