Rram-Based In-Memory Computing For Embedded Deep Neural Networks
CONFERENCE RECORD OF THE 2019 FIFTY-THIRD ASILOMAR CONFERENCE ON SIGNALS, SYSTEMS & COMPUTERS(2019)
摘要
Deploying state-of-the-art deep neural networks (DNNs) on embedded devices has created a major implementation challenge, largely due to the energy cost of memory access. RRAM-based in-memory processing units (IPUs) enable fully layerwise-pipelined architectures, minimizing the required SRAM memory capacity for storing feature maps and amortizing its access over hundreds to thousands of arithmetic operations. This paper presents an RRAM-based IPU featuring dynamic voltage-mode multiply-accumulate and a single-slope A/D readout scheme with RRAM-embedded ramp generator, which together eliminate power-hungry current-mode circuitry without sacrificing linearity. SPICE simulations suggest that this RRAM-based IPU architecture can achieve an array-level energy efficiency up to 1.2 2b-POps/s/W and an area efficiency exceeding 45 2b-TOps/s/mm(2).
更多查看译文
关键词
RRAM-based in-memory processing units,single-slope A/D readout scheme,RRAM-based IPU architecture,embedded devices,embedded deep neural networks,RRAM-based In-Memory Computing,power-hungry current-mode circuitry,RRAM-embedded ramp generator,dynamic voltage-mode multiply-accumulate,feature maps,required SRAM memory capacity,memory access,energy cost
AI 理解论文
溯源树
样例
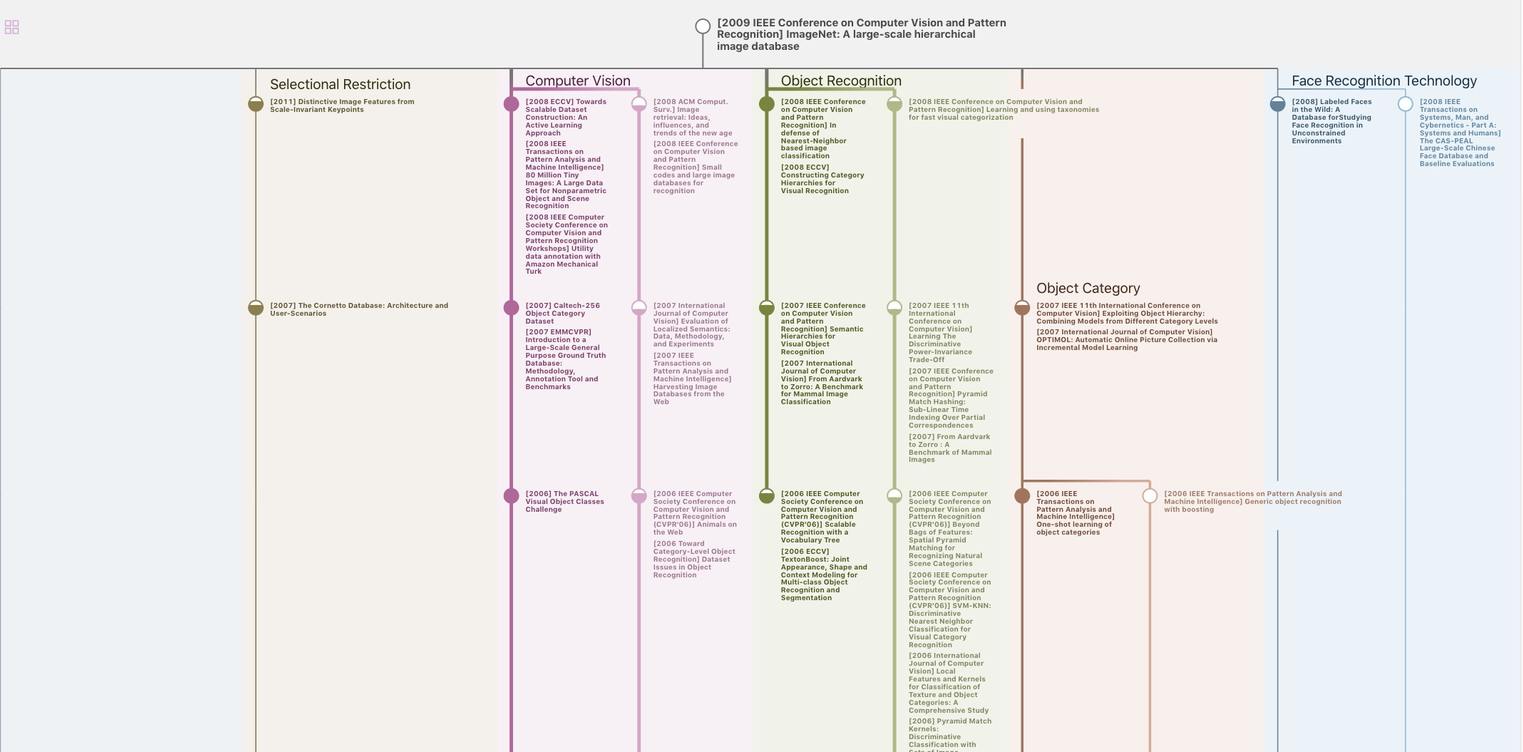
生成溯源树,研究论文发展脉络
Chat Paper
正在生成论文摘要