Learning to Measure the Static Friction Coefficient in Cloth Contact
CVPR(2020)
摘要
Measuring friction coefficients between cloth and an external body is a longstanding issue in mechanical engineering , never yet addressed with a pure vision-based system. The latter offers the prospect of simpler, less invasive friction measurement protocols compared to traditional ones, and can vastly benefit from recent deep learning advances. Such a novel measurement strategy however proves challenging , as no large labelled dataset for cloth contact exists, and creating one would require thousands of physics work-bench measurements with broad coverage of cloth-material pairs. Using synthetic data instead is only possible assuming the availability of a soft-body mechanical simulator with true-to-life friction physics accuracy, yet to be verified. We propose a first vision-based measurement network for friction between cloth and a substrate, using a simple and repeatable video acquisition protocol. We train our network on purely synthetic data generated by a state-of-the-art fric-tional contact simulator, which we carefully calibrate and validate against real experiments under controlled conditions. We show promising results on a large set of contact pairs between real cloth samples and various kinds of sub-strates, with 93.6% of all measurements predicted within 0.1 range of standard physics bench measurements.
更多查看译文
关键词
friction measurement protocols,standard physics bench measurements,cloth samples,contact pairs,state-of-the-art frictional contact simulator,synthetic data,repeatable video acquisition protocol,vision-based measurement network,true-to-life friction physics accuracy,soft-body mechanical simulator,cloth-material pairs,broad coverage,physics workbench measurements,deep learning,invasive friction measurement protocols,vision-based system,mechanical engineering,longstanding issue,external body,friction coefficients,cloth contact,static friction coefficient
AI 理解论文
溯源树
样例
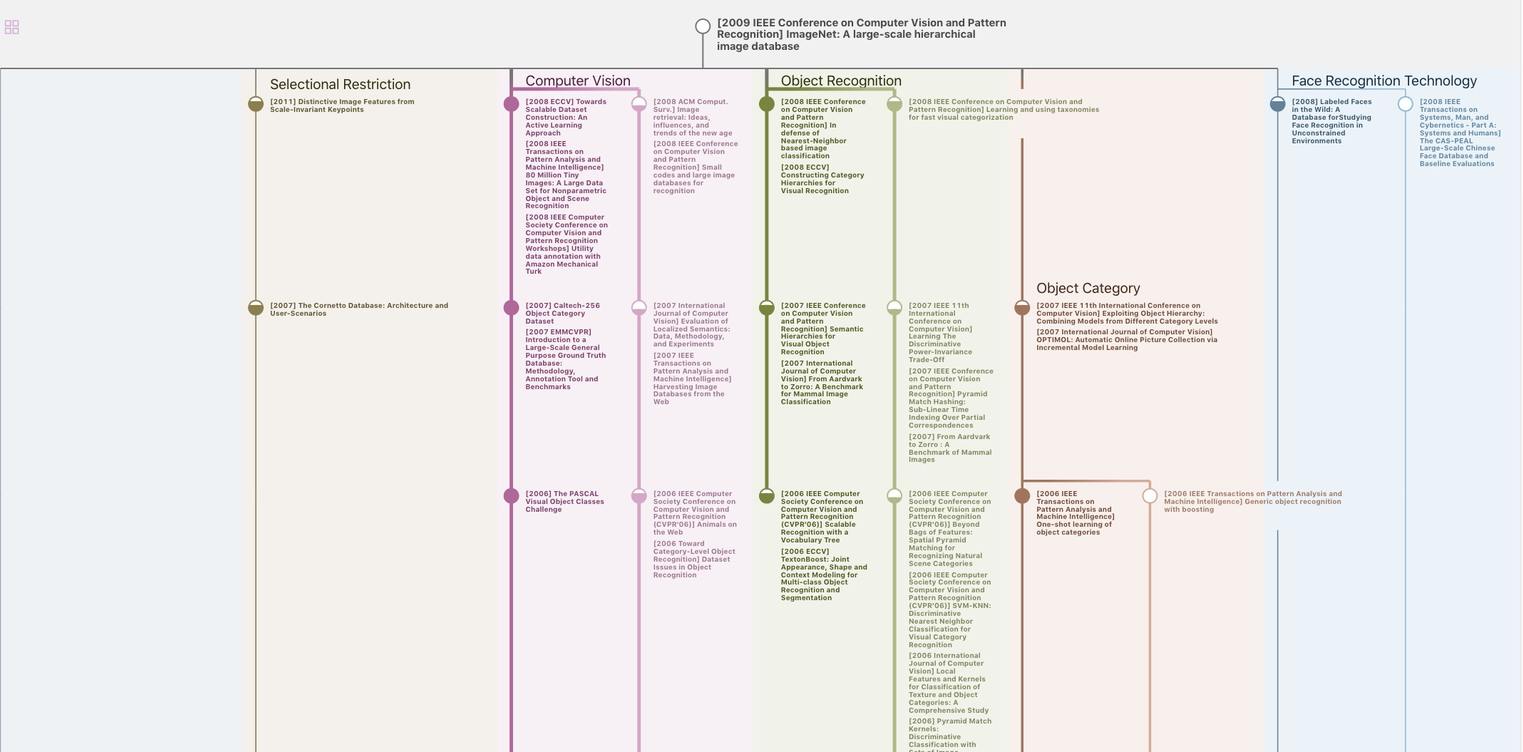
生成溯源树,研究论文发展脉络
Chat Paper
正在生成论文摘要