Deep Learning for Heart Rate Estimation from Reflectance Photoplethysmography with Acceleration Power Spectrum and Acceleration Intensity
IEEE ACCESS(2020)
摘要
Objective: A wearable reflectance-type photoplethysmography (PPG) sensor can be incorporated in a watch or band to provide instantaneous heart rates (HRs) with minimum inconvenience to users. However, the sensor is sensitive to motion artifacts (MAs), which results in inaccurate HR estimation. To address this problem, we propose a new neural network for deep learning to ensure accurate HR estimation even during intensive exercise. Methods: We propose a new deep neural network based on multiclass and non-uniform multilabel classification for HR estimation. It comprises of two convolutional layers, two long short-term memory (LSTM) layers, one concatenation layer, and three fully connected layers including a softmax. The proposed model feeds the power spectra from the PPG and acceleration signals along with the acceleration intensity to the input layer. We also present a new scheme to evaluate the loss value by modifying the true HR value into a Gaussian distribution. Results: We used 48 training datasets and evaluated 23 isolated testing datasets. The proposed model exhibited average absolute error of less than 1.5 bpm for all the training and test datasets & x2014;1.09 bpm for the training dataset and 1.46 bpm for the test dataset. Conclusion: The proposed model outperforms the state-of-the-art methods for accurate estimation of HR. Significance: It precisely estimates the HRs with robustness even during intensive physical exercise, as evidenced by the accuracy when PPG signals are severely corrupted by MAs.
更多查看译文
关键词
Reflectance-type photoplethysmography,instantaneous heart rate,deep learning,convolutional layer,long short-term memory (LSTM) layer
AI 理解论文
溯源树
样例
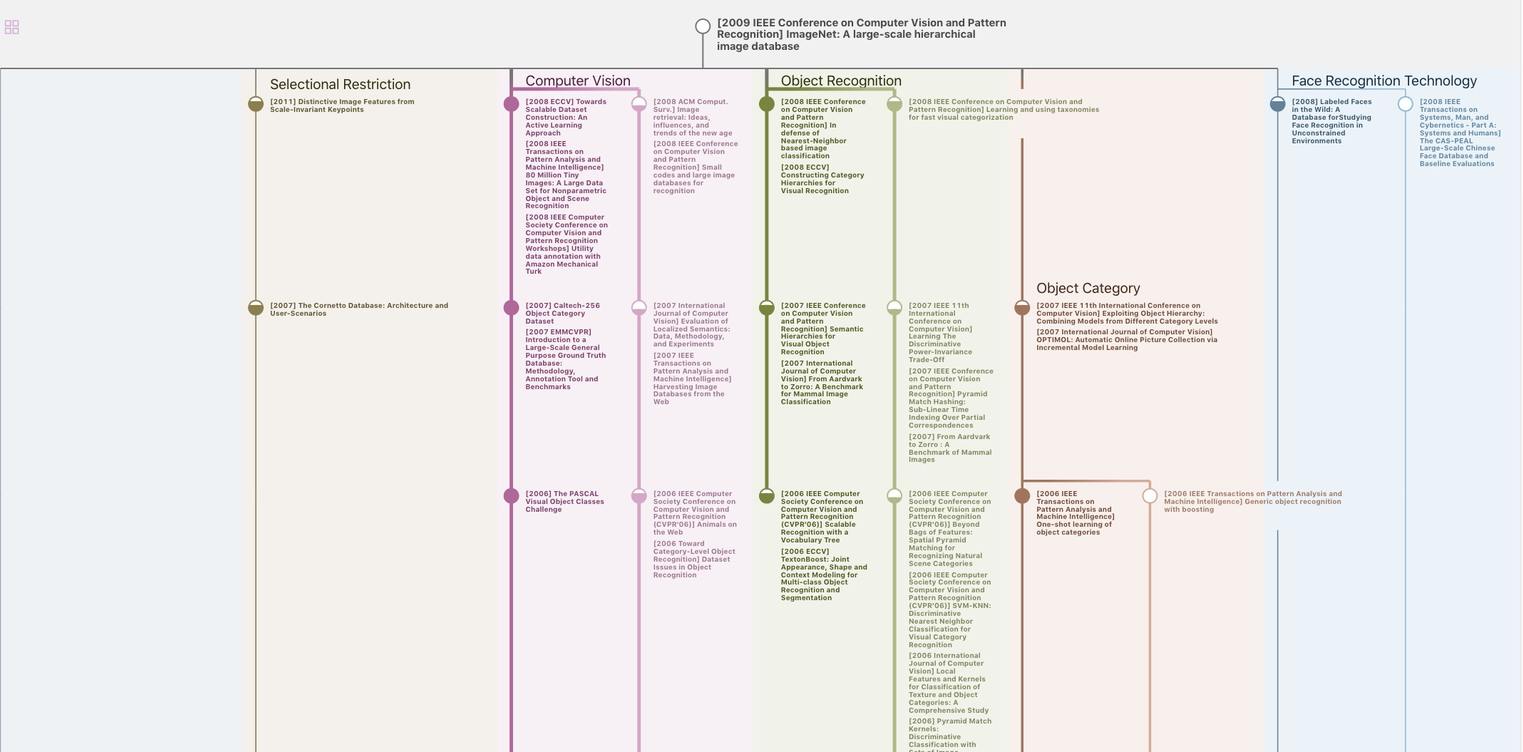
生成溯源树,研究论文发展脉络
Chat Paper
正在生成论文摘要