Prediction Of Tumour Pathological Subtype From Genomic Profile Using Sparse Logistic Regression With Random Effects
JOURNAL OF APPLIED STATISTICS(2021)
摘要
The purpose of this study is to highlight the application of sparse logistic regression models in dealing with prediction of tumour pathological subtypes based on lung cancer patients' genomic information. We consider sparse logistic regression models to deal with the high dimensionality and correlation between genomic regions. In a hierarchical likelihood (HL) method, it is assumed that the random effects follow a normal distribution and its variance is assumed to follow a gamma distribution. This formulation considers ridge and lasso penalties as special cases. We extend the HL penalty to include a ridge penalty (called 'HLnet') in a similar principle of the elastic net penalty, which is constructed from lasso penalty. The results indicate that the HL penalty creates more sparse estimates than lasso penalty with comparable prediction performance, while HLnet and elastic net penalties have the best prediction performance in real data. We illustrate the methods in a lung cancer study.
更多查看译文
关键词
Tumour, lung cancer, pathological subtype, logistic regression, sparse solution, hierarchical likelihood
AI 理解论文
溯源树
样例
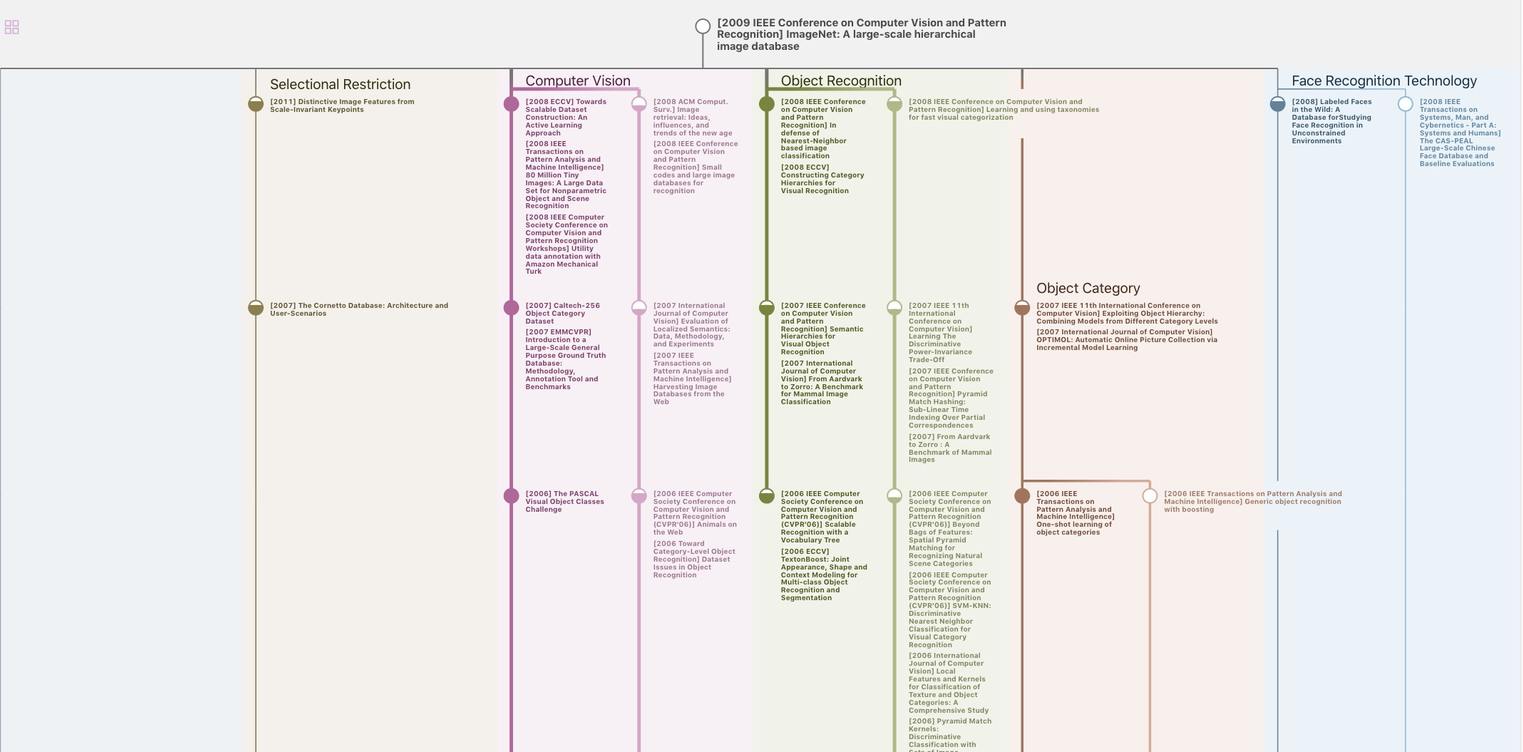
生成溯源树,研究论文发展脉络
Chat Paper
正在生成论文摘要