Machine Learning Accelerated Recovery of the Cubic Structure in Mixed-Cation Perovskite Thin Films
CHEMISTRY OF MATERIALS(2020)
摘要
Data-driven approaches for materials design and selection have accelerated materials discovery along with the upsurge of machine learning applications. We report here a prediction-to-lab-scale synthesis of cubic phase triple-cation lead halide perovskites guided by a machine learning perovskite stability predictor. The starting double-cation perovskite resulting from the incorporation of 15% dimethylammonium (DMA) in methyl-ammonium lead triiodide suffers from significant deviation from the perovskite structure. By analyzing the X-ray diffraction and scanning electron microscopy, we confirmed that it is possible to recover the perovskite structure with the cubic phase at room temperature (RT) while minimizing the iterations of trial-and-error by adding <10 mol % of cesium cation additives, as guided by the machine learning predictor. Our conclusions highly support the cubic-phase stabilization at RT by controlling the stoichiometric ratio of various sized cations. This prediction-to-lab-scale synthesis approach also enables us to identify room for improvements of the current machine learning predictor to take into consideration the cubic phase stability as well as phase segregation.
更多查看译文
关键词
perovskite,cubic structure,thin films,mixed-cation
AI 理解论文
溯源树
样例
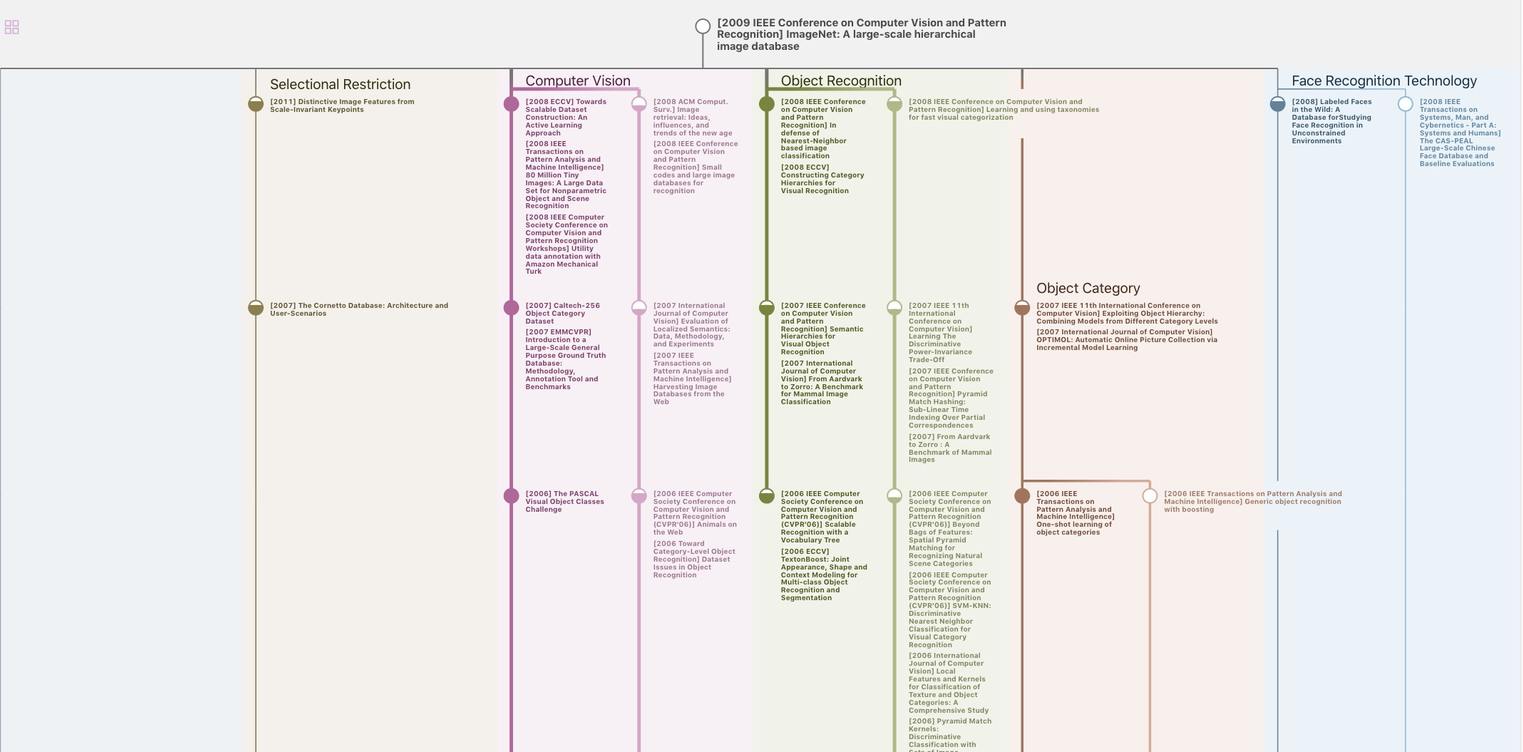
生成溯源树,研究论文发展脉络
Chat Paper
正在生成论文摘要