MaldomDetector: A system for detecting algorithmically generated domain names with machine learning
Computers & Security(2020)
摘要
One of the leading problems in cyber security at present is the unceasing emergence of sophisticated attacks, such as botnets and ransomware, that rely heavily on Command and Control (C&C) channels to conduct their malicious activities remotely. To avoid channel detection, attackers constantly try to create different covert communication techniques. One such technique is Domain Generation Algorithm (DGA), which allows malware to generate numerous domain names until it finds its corresponding C&C server. It is highly resilient to detection systems and reverse engineering, while allowing the C&C server to have several redundant domain names. This paper presents a malicious domain name detection system, MaldomDetector, which is based on machine learning. It is capable of detecting DGA-based communications and circumventing the attack before it makes any successful connection with the C&C server, using only domain name's characters. MaldomDetector uses a set of easy-to-compute and language-independent features in addition to a deterministic algorithm to detect malicious domains. The experimental results demonstrate that MaldomDetector can operate efficiently as a first alarm to detect DGA-based domains of malware families while maintaining high detection accuracy.
更多查看译文
关键词
Network security,Intrusion detection,Machine learning,Command and control,Domain Generation Algorithm (DGA),DNS,Domain name
AI 理解论文
溯源树
样例
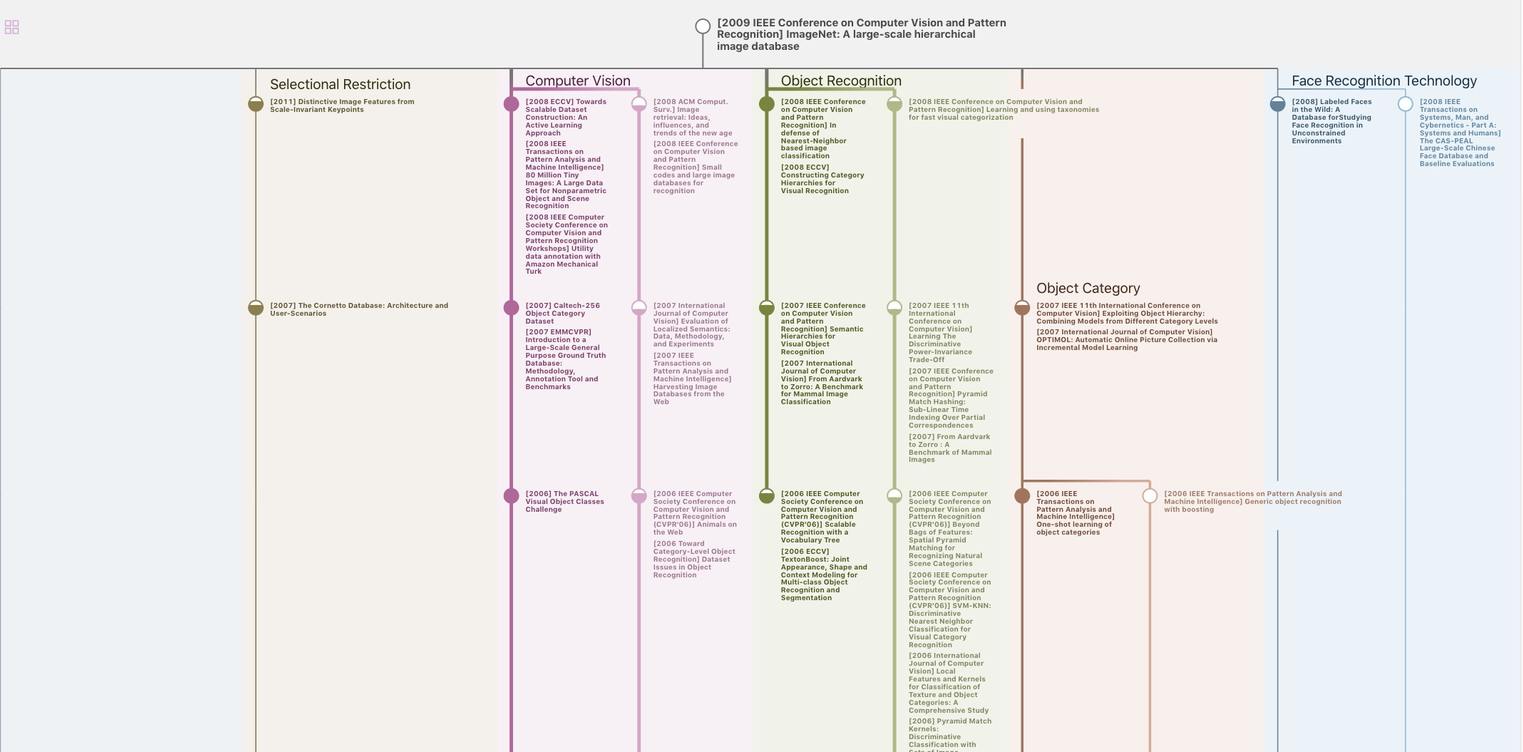
生成溯源树,研究论文发展脉络
Chat Paper
正在生成论文摘要