Classification-Friendly Sparse Encoder And Classifier Learning
IEEE ACCESS(2020)
Abstract
Sparse representation (SR) and dictionary learning (DL) have been extensively used for feature encoding, aiming to extract the latent classification-friendly feature of observed data. Existing methods use sparsity penalty and learned dictionary to enhance discriminative capability of sparse codes. However, training dictionary for SR is time consuming and the resulted discriminative capability is limited. Rather than learning dictionary, we propose to employ the dictionary at hand, e.g., the training set as the class-specific synthesis dictionary to pursue an ideal discriminative property of SR of the training samples: each data can be represented only by data-in-class. In addition to the discriminative property, we also introduce a smoothing term to enforce the representation vectors to be uniform within class. The discriminative property helps to separate the data from different classes while the smoothing term tends to group the data from the same class and further strengthen the separation. The SRs are used as new features to train a sparse encoder and a classifier. Once the sparse encoder and the classifier are learnt, the test stage is very simple and highly efficient. Specifically, the label of a test sample can be easily computed by multiplying the test sample with the sparse encoder and the classifier. We call our method Classification-Friendly Sparse Encoder and Classifier Learning (CF-SECL). Extensive experiments show that our method outperforms some state-of the-art model-based methods.
MoreTranslated text
Key words
Sparse representation, discriminative sparse encoder, pattern classification
AI Read Science
Must-Reading Tree
Example
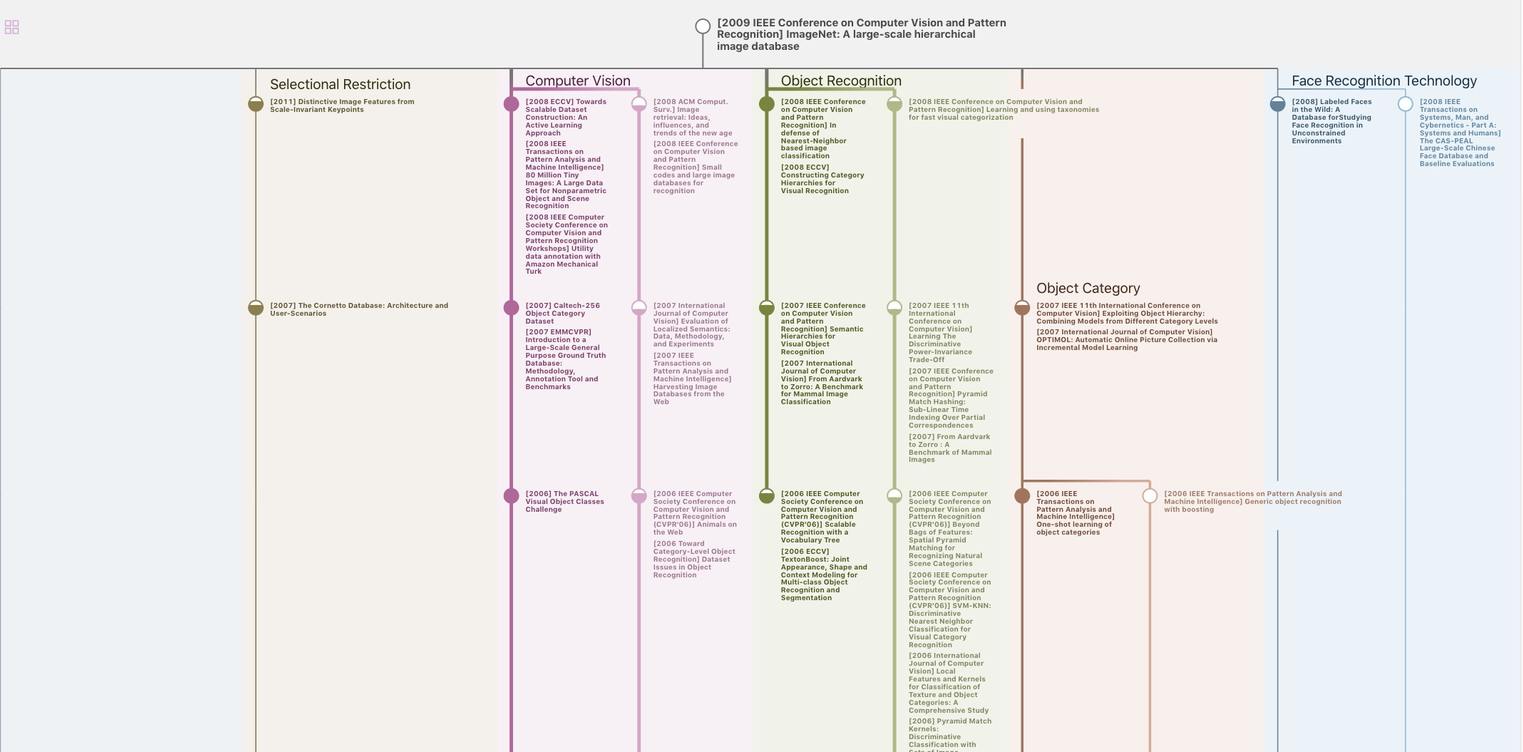
Generate MRT to find the research sequence of this paper
Chat Paper
Summary is being generated by the instructions you defined