Near infrared broadband and visible upconversion emissions of erbium ions in oxyfluoride glasses for optical amplifier applications
OPTICS AND LASER TECHNOLOGY(2020)
摘要
Optical and visible upconversion properties of erbium (Er3+)-doped oxyfluoro-titania-phosphate glasses (PCfBffiEr) with the chemical composition of P2O5-CaF2-BaF2-TiO2-Er2O3 have been explored. An intense emission at 1.53 mu m of Er3+-doped PCfBfTiEr1.0 glass was obtained upon excitation of 980 nm diode laser. In addition, green and red visible upconversion emissions were obtained upon the optical excitation of Er3+ ions doped PCfBffiEr glasses at 980 nm diode laser. Upconversion emission intensities and population densities of respective levels were tuned with the variation of Er3+ ion concentration. Fluorescence decay curves of the I-4(13/2) level of PCfBfFiEr glasses were obtained upon 980 nm laser excitation in the pulsed mode and revealed a monoexponential behavior. The stimulated emission cross-section (a em ), full width at half maximum (FWHM) and gain bandwidth product (sigma(em) x FWHM) were found to be 9.3 x 10(-21) cm(2), 95.61 nm and 889.2 cm(-2) nm for PCfBfFiEr2.0 glass, respectively. These results recommend that the Er3+ ions doped PCfBfFiEr glasses may possibly be worthy for the laser and optical amplification applications at 1.53 mu m.
更多查看译文
关键词
Oxyfluoro-phosphate glasses,Erbium ions,Optical band gap,Optical gain bandwidth,Decay curves,Lasers and amplifiers
AI 理解论文
溯源树
样例
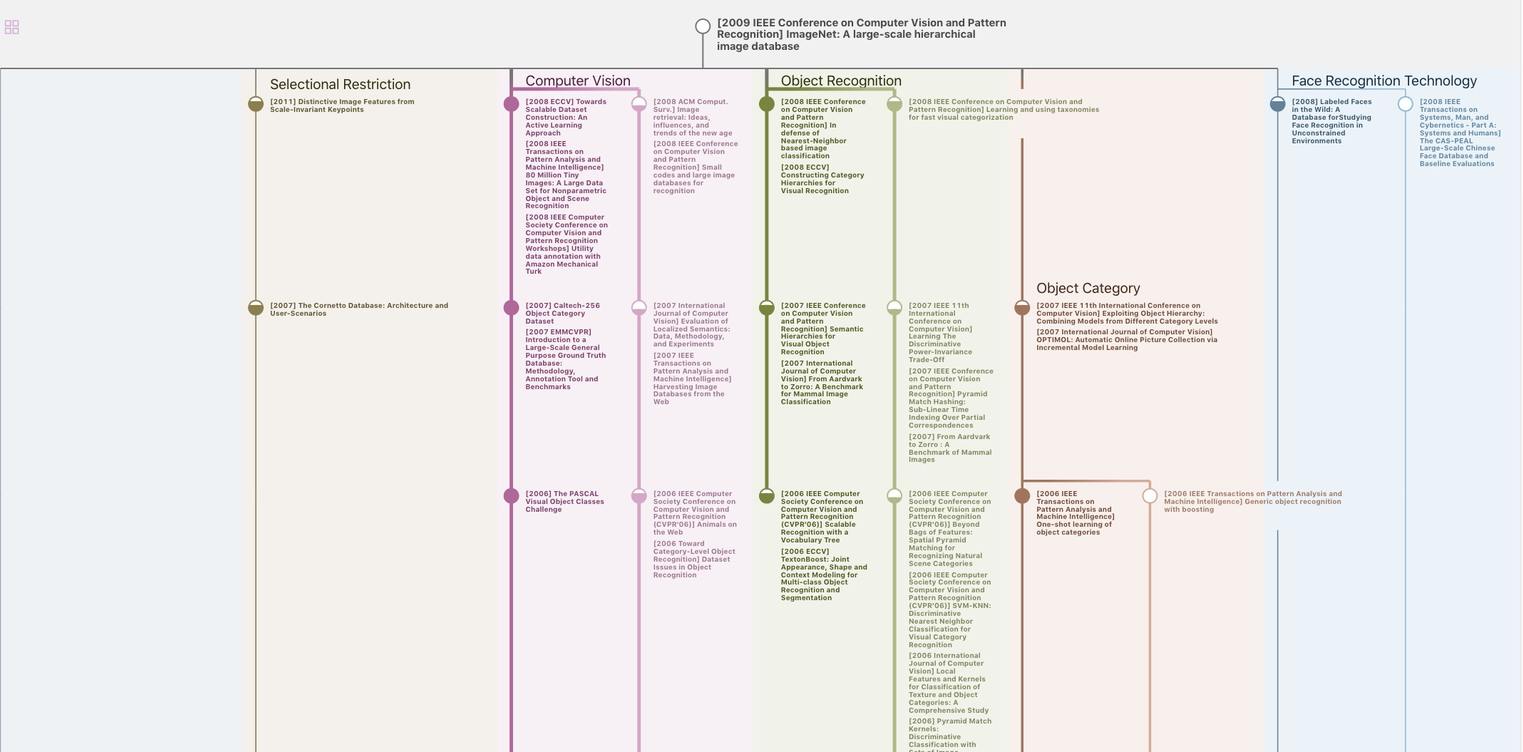
生成溯源树,研究论文发展脉络
Chat Paper
正在生成论文摘要