A Competitive Co-Evolutionary Approach For The Multi-Objective Evolutionary Algorithms
IEEE ACCESS(2020)
摘要
In multi-objective evolutionary algorithms (MOEAs), convergence and diversity are two basic issues and keeping a balance between them plays a vital role. There are several studies that have attempted to address this problem, but this is still an open challenge. It is thus the purpose of this research to develop a dual-population competitive co-evolutionary approach to improving the balance between convergence and diversity. We utilize two populations to solve separate tasks. The first population uses Pareto-based ranking scheme to achieve better convergence, and the second one tries to guarantee population diversity via the use of a decomposition-based method. Next, by operating a competitive mechanism to combine the two populations, we create a new one with a view to having both characteristics (i.e. convergence and diversity). The proposed method & x2019;s performance is measured by the renowned benchmarks of multi-objective optimization problems (MOPs) using the hypervolume (HV) and the inverted generational distance (IGD) metrics. Experimental results show that the proposed method outperforms cutting-edge co-evolutionary algorithms with a robust performance.
更多查看译文
关键词
Dual-population, convergence, diversity, co-evolution, competitive
AI 理解论文
溯源树
样例
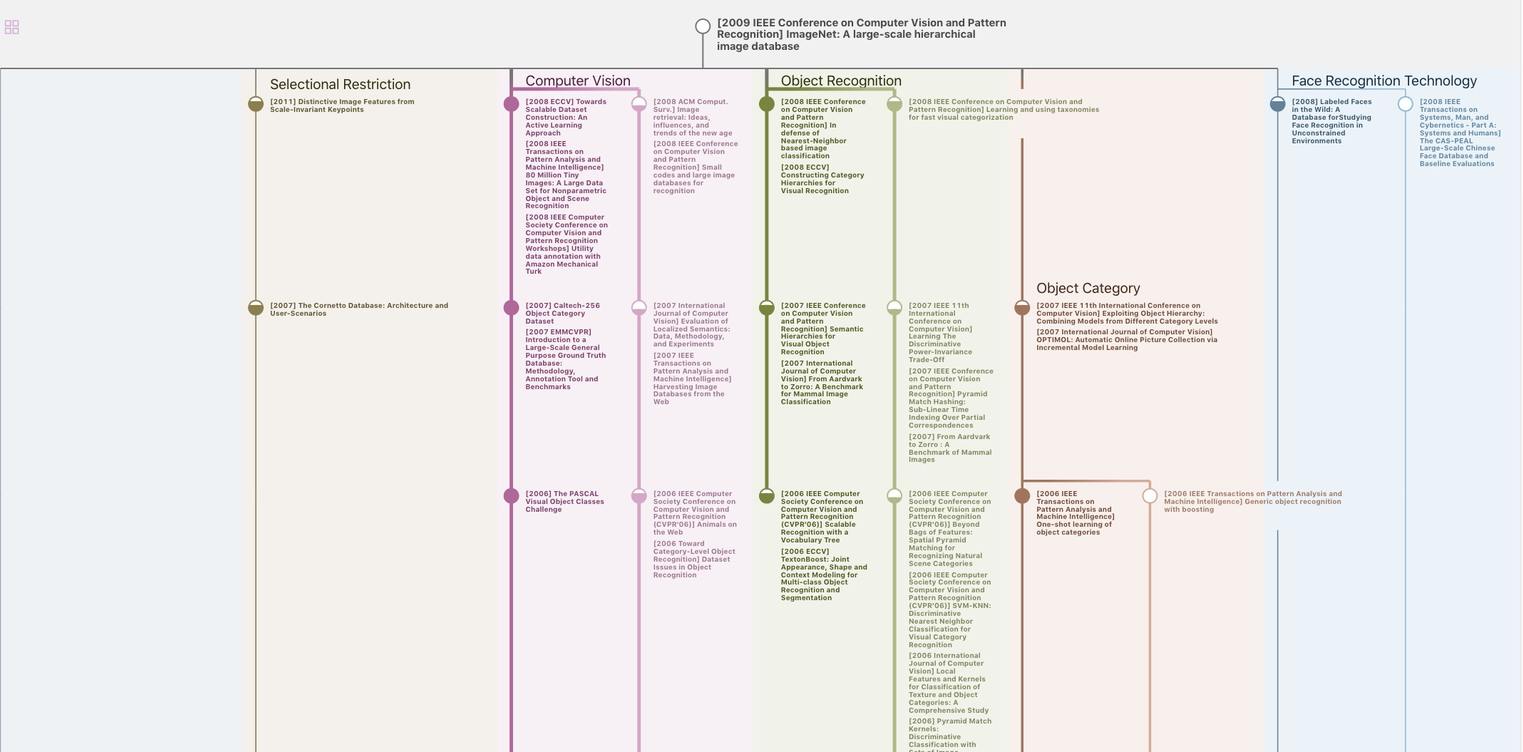
生成溯源树,研究论文发展脉络
Chat Paper
正在生成论文摘要