Exploring nonnegative and low-rank correlation for noise-resistant spectral clustering
World Wide Web(2020)
摘要
Clustering has been extensively explored in pattern recognition and data mining in order to facilitate various applications. Due to the presence of data noise, traditional clustering approaches may become vulnerable and unreliable, thereby degrading clustering performance. In this paper, we propose a robust spectral clustering approach, termed Non-negative Low-rank Self-reconstruction (NLS), which simultaneously a) explores the nonnegative low-rank properties of data correlation as well as b) adaptively models the structural sparsity of data noise. Specifically, in order to discover the intrinsic correlation among data, we devise a self-reconstruction approach to jointly consider the nonnegativity and low-rank property of data correlation matrix. Meanwhile, we propose to model data noise via a structural norm, i.e., ℓ p ,2 -norm, which not only naturally conforms to genuine patterns of data noise in real-world situations, but also provides more adaptivity and flexibility to different noise levels. Extensive experiments on various real-world datasets illustrate the advantage of the proposed robust spectral clustering approach compared to existing clustering methods.
更多查看译文
关键词
Non-negative, Low-rank, Structural sparsity
AI 理解论文
溯源树
样例
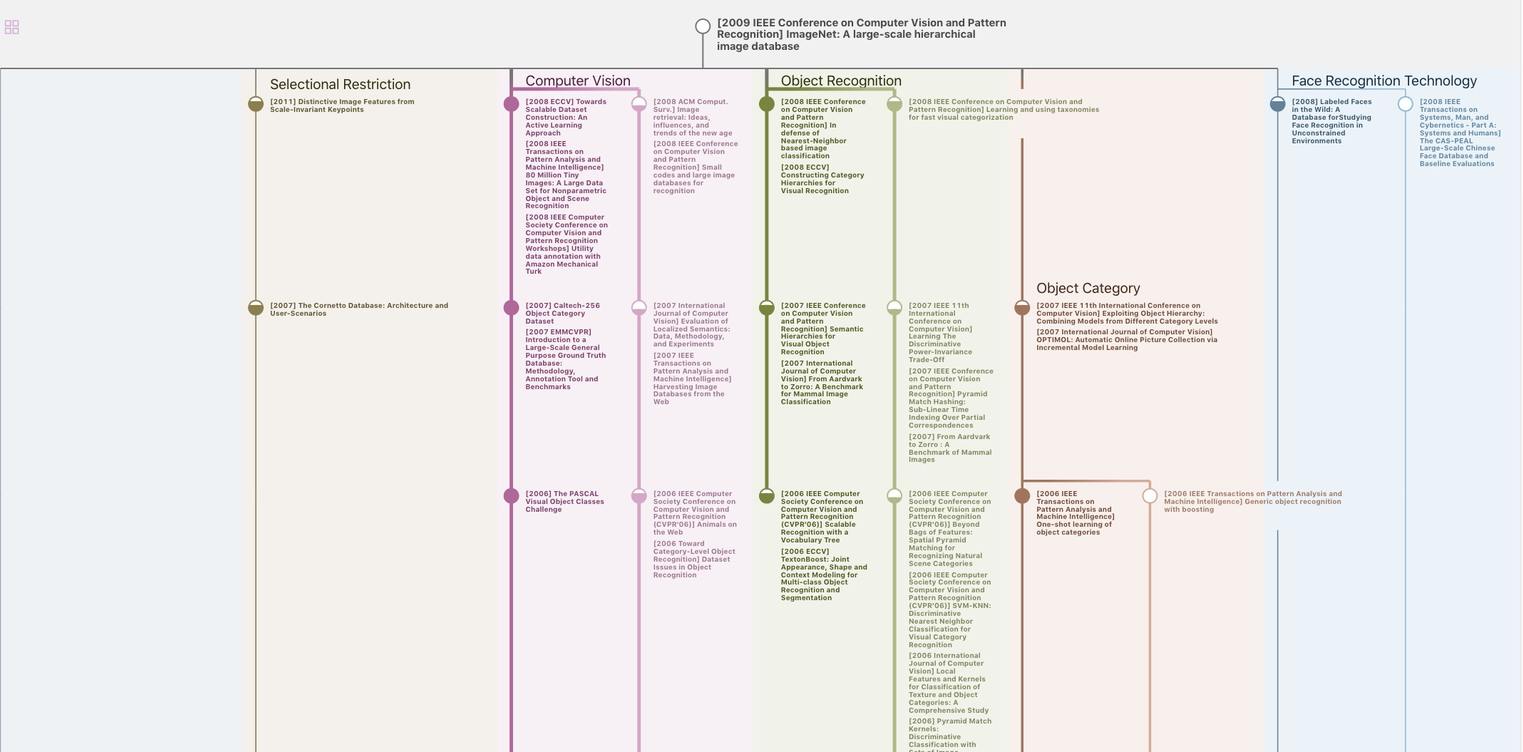
生成溯源树,研究论文发展脉络
Chat Paper
正在生成论文摘要