Cross-View Feature Learning via Structures Unlocking Based on Robust Low-Rank Constraint
IEEE ACCESS(2020)
摘要
The cross-view multimedia are widely existed and attract many attentions in recent years. Nevertheless, it is noted that the phenomenon, that data in different classes from same view are more similar than that in same class from different views, is usually presented for cross-view multimedia data. The intrinsic imperfection leads disappointing performance for cross-view multimedia recognition or classification. To solve this problem, in this paper, we propose a novel discriminative learning framework with low-rank constraint, which can be applied for view-invariant low-dimensional subspace learning. The advantages of our framework include three aspects. Firstly, to unlock the latent class structure and view structure, a self-expressed model by dual low-rank constraints are presented, which can separate the two manifold structures in the learned subspace. Secondly, two effective discriminative graphs are constructed to guide the affinity relationship of data in the above two low-dimensional projected subspaces respectively. Finally, the joint semantic consensus constraint is designed to be integrated into the learning framework, which can explore the shared and view-specific information for enforcing the view-invariant character in semantic space. Experimental results on several public cross-view multimedia datasets demonstrate that our proposed method outperforms existing excellent subspace learning approaches.
更多查看译文
关键词
Multimedia analysis,multi-view discriminative analysis,cross-view feature learning,low-rank representation
AI 理解论文
溯源树
样例
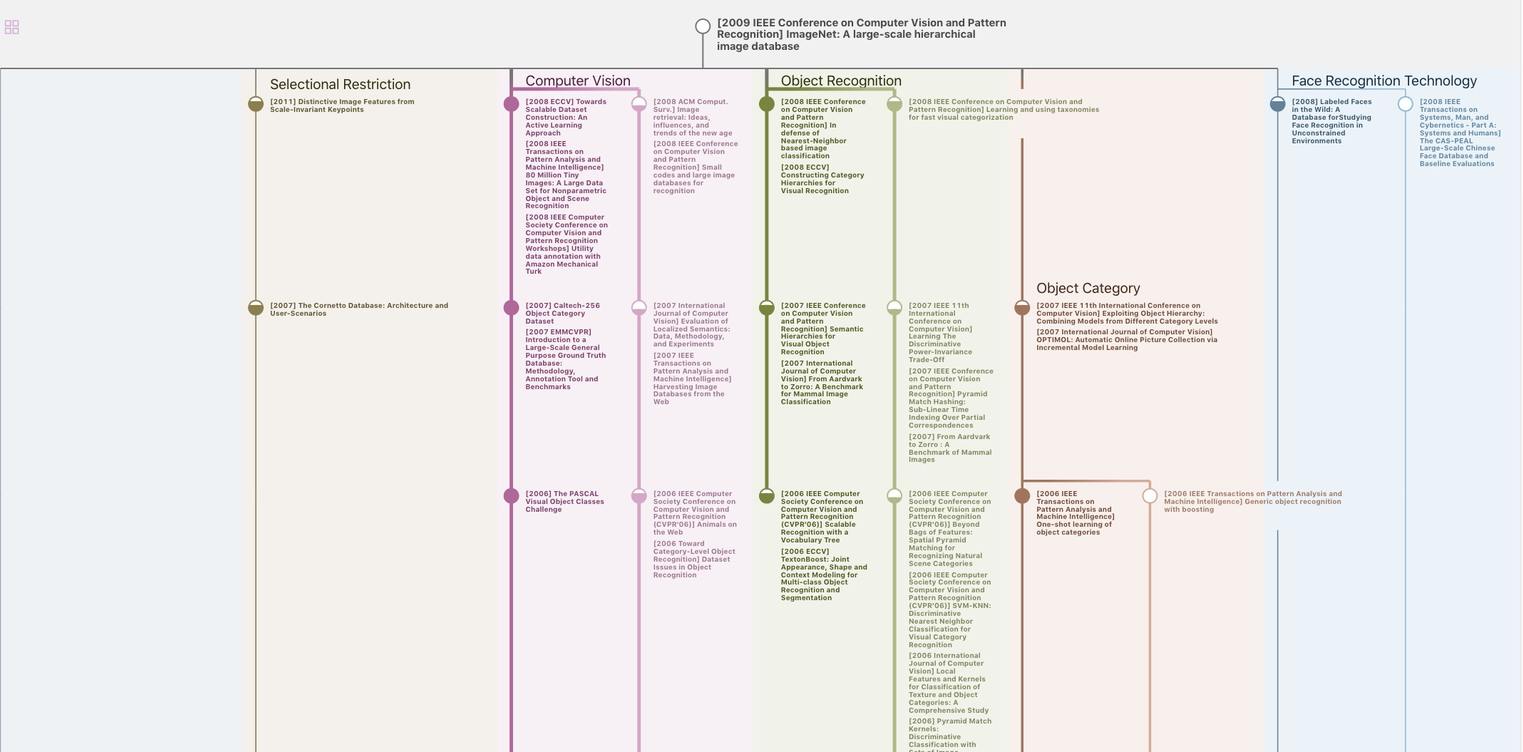
生成溯源树,研究论文发展脉络
Chat Paper
正在生成论文摘要