Structure Fusion Based on Graph Convolutional Networks for Node Classification in Citation Networks
ELECTRONICS(2020)
摘要
Suffering from the multi-view data diversity and complexity, most of the existing graph convolutional networks focus on the networks' architecture construction or the salient graph structure preservation for node classification in citation networks and usually ignore capturing the complete graph structure of nodes for enhancing classification performance. To mine the more complete distribution structure from multi-graph structures of multi-view data with the consideration of their specificity and the commonality, we propose structure fusion based on graph convolutional networks (SF-GCN) for improving the performance of node classification in a semi-supervised way. SF-GCN can not only exploit the special characteristic of each view datum by spectral embedding preserving multi-graph structures, but also explore the common style of multi-view data by the distance metric between multi-graph structures. Suppose the linear relationship between multi-graph structures; we can construct the optimization function of the structure fusion model by balancing the specificity loss and the commonality loss. By solving this function, we can simultaneously obtain the fusion spectral embedding from the multi-view data and the fusion structure as the adjacent matrix to input graph convolutional networks for node classification in a semi-supervised way. Furthermore, we generalize the structure fusion to structure diffusion propagation and present structure propagation fusion based on graph convolutional networks (SPF-GCN) for utilizing these structure interactions. Experiments demonstrate that the performance of SPF-GCN outperforms that of the state-of-the-art methods on three challenging datasets, which are Cora, Citeseer, and Pubmed in citation networks.
更多查看译文
关键词
structure fusion,graph convolutional networks,node classification,citation networks
AI 理解论文
溯源树
样例
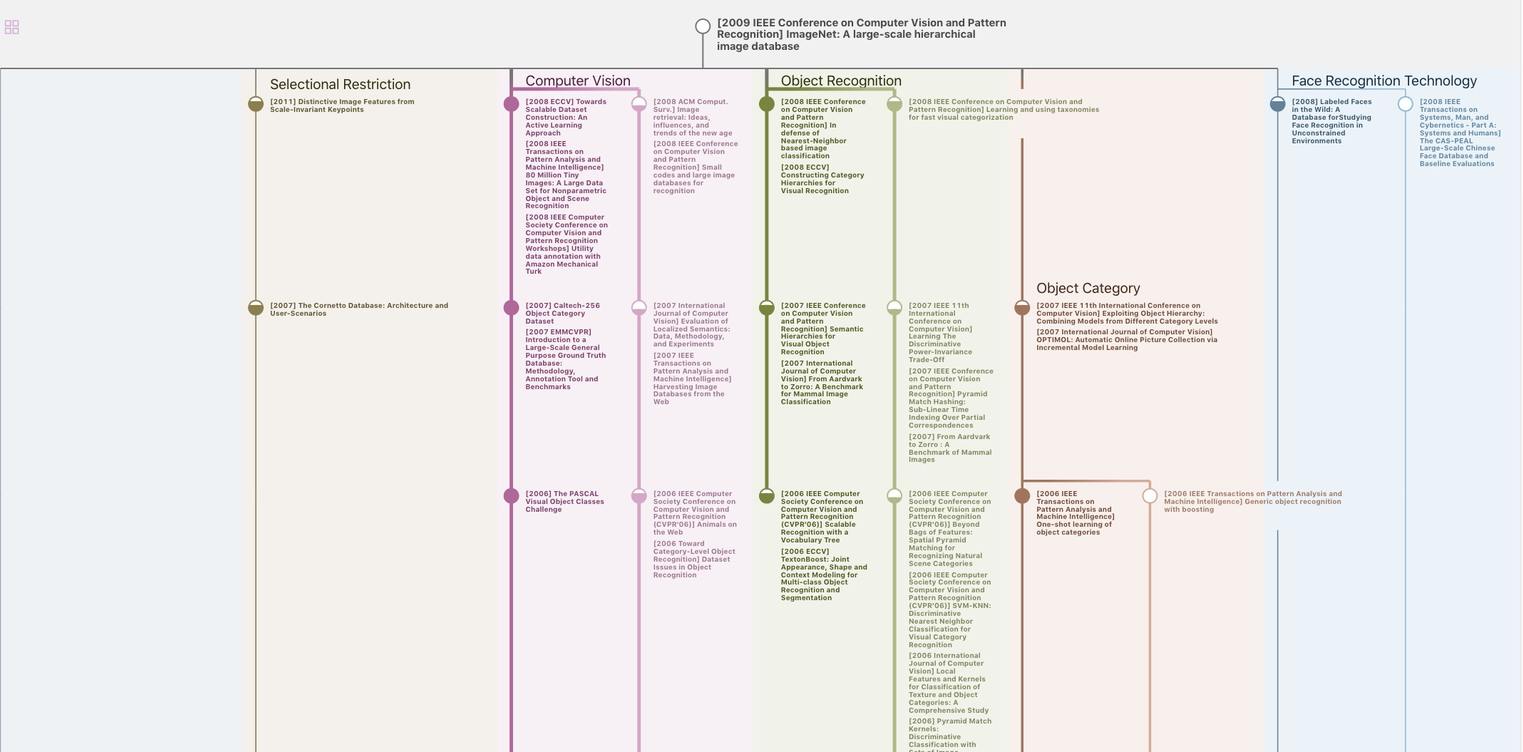
生成溯源树,研究论文发展脉络
Chat Paper
正在生成论文摘要