Real-Time Vehicle Detection Framework Based on the Fusion of LiDAR and Camera
ELECTRONICS(2020)
摘要
Vehicle detection is essential for driverless systems. However, the current single sensor detection mode is no longer sufficient in complex and changing traffic environments. Therefore, this paper combines camera and light detection and ranging (LiDAR) to build a vehicle-detection framework that has the characteristics of multi adaptability, high real-time capacity, and robustness. First, a multi-adaptive high-precision depth-completion method was proposed to convert the 2D LiDAR sparse depth map into a dense depth map, so that the two sensors are aligned with each other at the data level. Then, the You Only Look Once Version 3 (YOLOv3) real-time object detection model was used to detect the color image and the dense depth map. Finally, a decision-level fusion method based on bounding box fusion and improved Dempster-Shafer (D-S) evidence theory was proposed to merge the two results of the previous step and obtain the final vehicle position and distance information, which not only improves the detection accuracy but also improves the robustness of the whole framework. We evaluated our method using the KITTI dataset and the Waymo Open Dataset, and the results show the effectiveness of the proposed depth completion method and multi-sensor fusion strategy.
更多查看译文
关键词
autonomous vehicle,vehicle detection,depth completion,decision-level fusion,D-S evidence theory
AI 理解论文
溯源树
样例
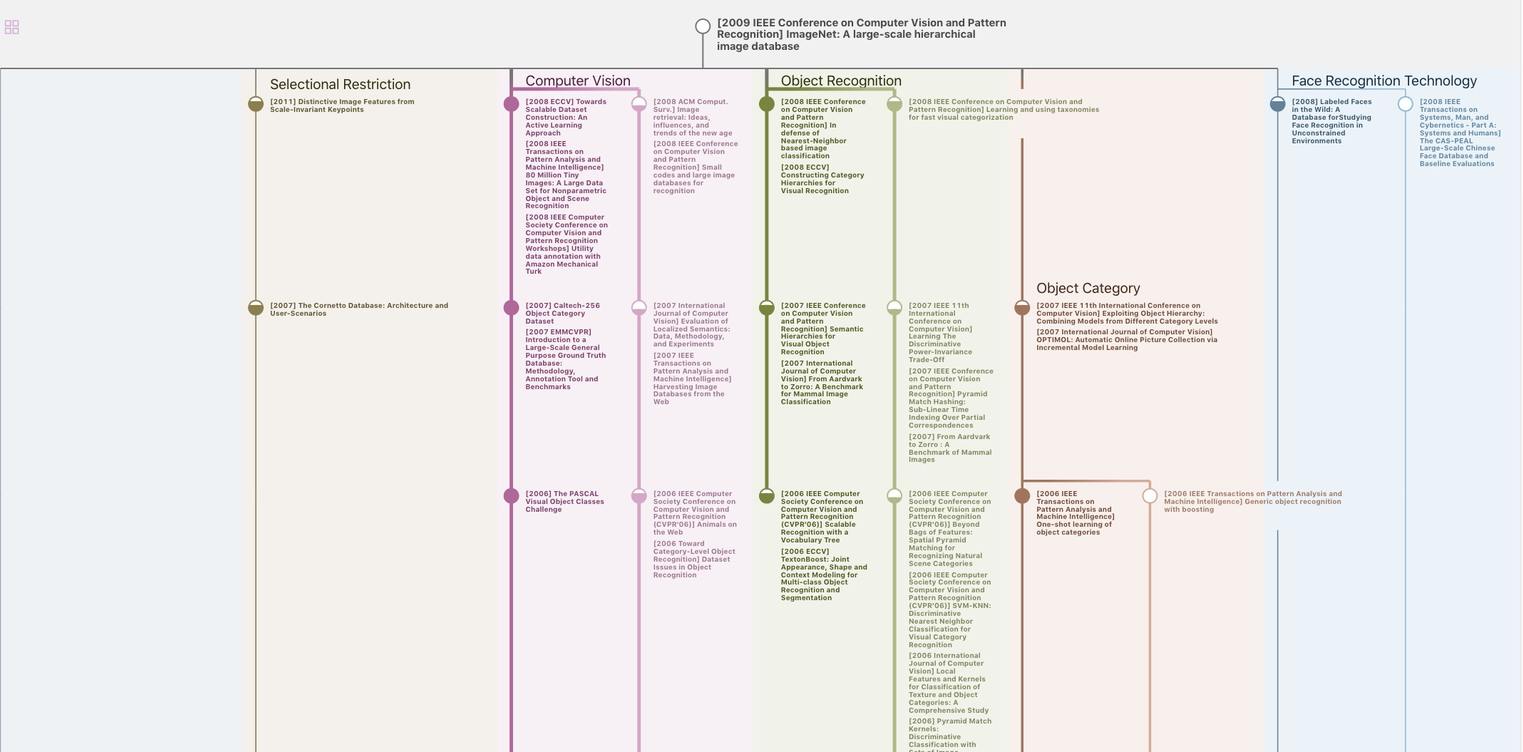
生成溯源树,研究论文发展脉络
Chat Paper
正在生成论文摘要