EasyAug: An Automatic Textual Data Augmentation Platform for Classification Tasks
WWW '20: The Web Conference 2020 Taipei Taiwan April, 2020(2020)
Abstract
Imbalanced data is a perennial problem that impedes the learning abilities of current machine learning-based classification models. One approach to address it is to leverage data augmentation to expand the training set. For image data, there are a number of suitable augmentation techniques that have proven effective in previous work. For textual data, however, due to the discrete units inherent in natural language, techniques that randomly perturb the signal may be ineffective. Additionally, due to the substantial discrepancy between different textual datasets (e.g., different domains), an augmentation approach that facilitates the classification on one dataset may be detrimental on another dataset. For practitioners, comparing different data augmentation techniques is non-trivial, as the corresponding methods might need to be incorporated into different system architectures, and the implementation of some approaches, such as generative models, is laborious. To address these challenges, we develop EasyAug, a data augmentation platform that provides several augmentation approaches. Users can conveniently compare the classification results and can easily choose the most suitable one for their own dataset. In addition, the system is extensible and can incorporate further augmentation approaches, such that with minimal effort a new method can comprehensively be compared with the baselines.
MoreTranslated text
Key words
imbalanced data, data augmentation, text generation, model fusion, text classification
AI Read Science
Must-Reading Tree
Example
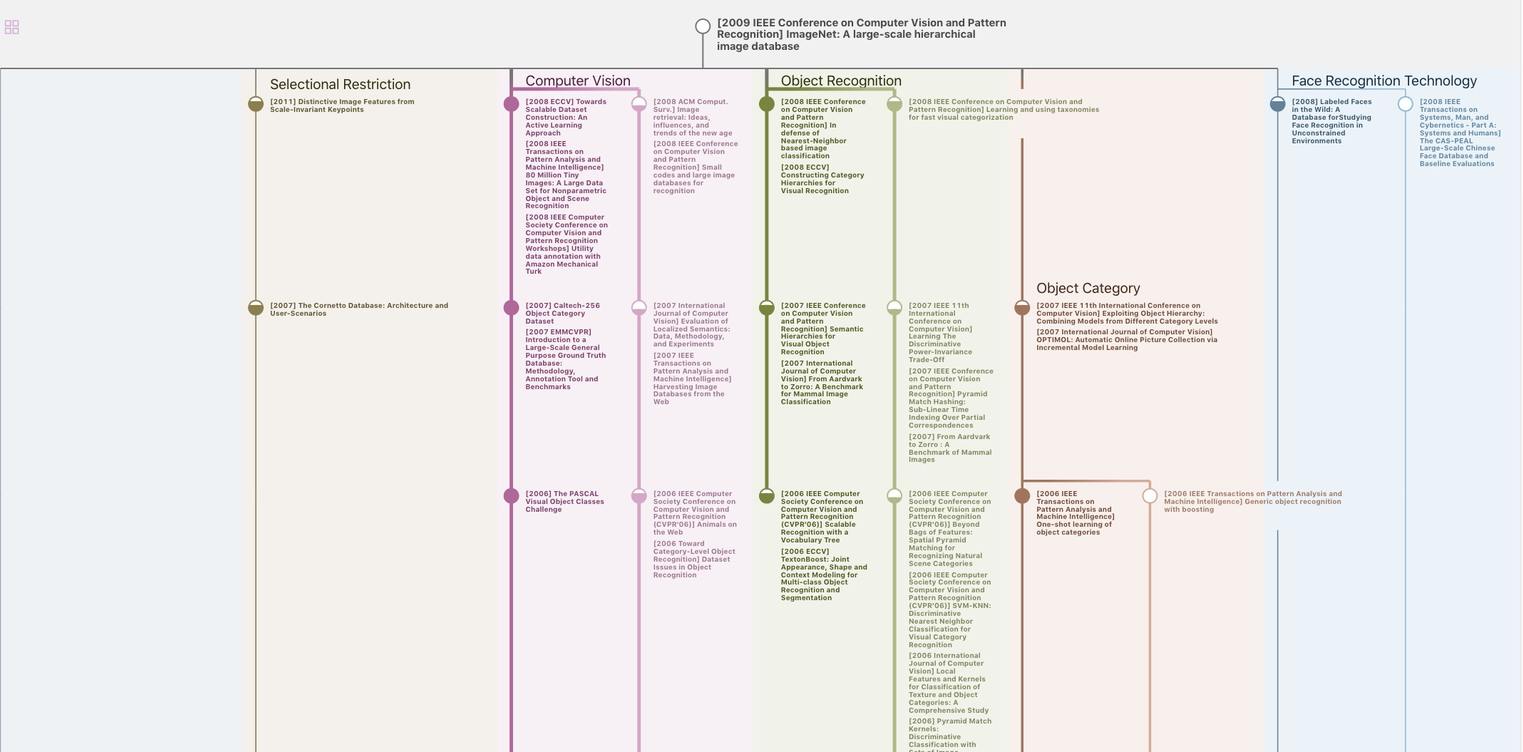
Generate MRT to find the research sequence of this paper
Chat Paper
Summary is being generated by the instructions you defined