Multimodal Post Attentive Profiling for Influencer Marketing
WWW '20: The Web Conference 2020 Taipei Taiwan April, 2020(2020)
摘要
Influencer marketing has become a key marketing method for brands in recent years. Hence, brands have been increasingly utilizing influencers’ social networks to reach niche markets, and researchers have been studying various aspects of influencer marketing. However, brands have often suffered from searching and hiring the right influencers with specific interests/topics for their marketing due to a lack of available influencer data and/or limited capacity of marketing agencies. This paper proposes a multimodal deep learning model that uses text and image information from social media posts (i) to classify influencers into specific interests/topics (e.g., fashion, beauty) and (ii) to classify their posts into certain categories. We use the attention mechanism to select the posts that are more relevant to the topics of influencers, thereby generating useful influencer representations. We conduct experiments on the dataset crawled from Instagram, which is the most popular social media for influencer marketing. The experimental results show that our proposed model significantly outperforms existing user profiling methods by achieving 98% and 96% accuracy in classifying influencers and their posts, respectively. We release our influencer dataset of 33,935 influencers labeled with specific topics based on 10,180,500 posts to facilitate future research.
更多查看译文
关键词
Influencer marketing, Multimodal neural network, Multi-task learning, Influencer profiling, Social media, User profiling
AI 理解论文
溯源树
样例
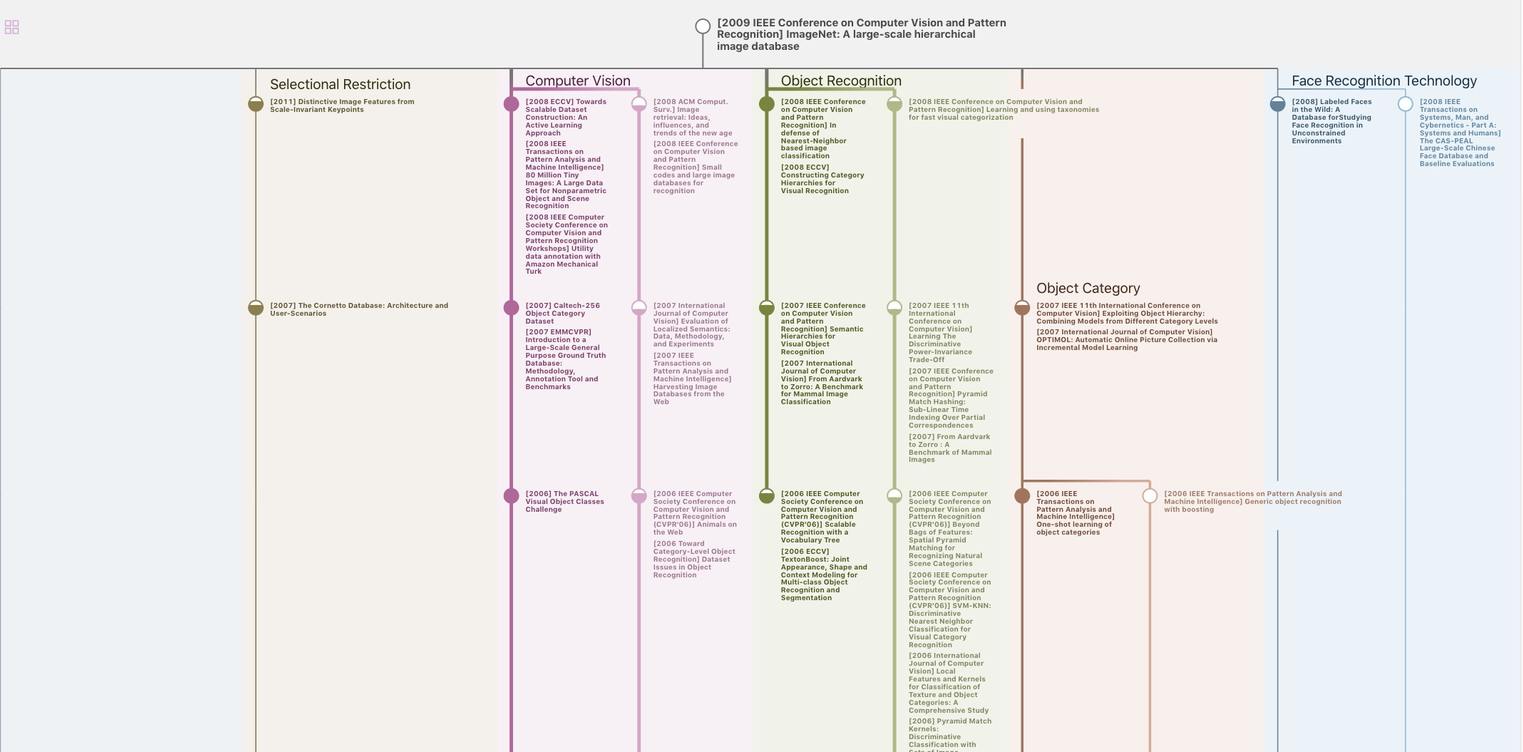
生成溯源树,研究论文发展脉络
Chat Paper
正在生成论文摘要