A transformation-free linear regression for compositional outcomes and predictors
BIOMETRICS(2022)
Abstract
Compositional data are common in many fields, both as outcomes and predictor variables. The inventory of models for the case when both the outcome and predictor variables are compositional is limited, and the existing models are often difficult to interpret in the compositional space, due to their use of complex log-ratio transformations. We develop a transformation-free linear regression model where the expected value of the compositional outcome is expressed as a single Markov transition from the compositional predictor. Our approach is based on estimating equations thereby not requiring complete specification of data likelihood and is robust to different data-generating mechanisms. Our model is simple to interpret, allows for 0s and 1s in both the compositional outcome and covariates, and subsumes several interesting subcases of interest. We also develop permutation tests for linear independence and equality of effect sizes of two components of the predictor. Finally, we show that despite its simplicity, our model accurately captures the relationship between compositional data using two datasets from education and medical research.
MoreTranslated text
Key words
compositional data,expectation‐,maximization algorithm,estimating equation,Kullback–,Leibler distance loss function,transformation‐,free
AI Read Science
Must-Reading Tree
Example
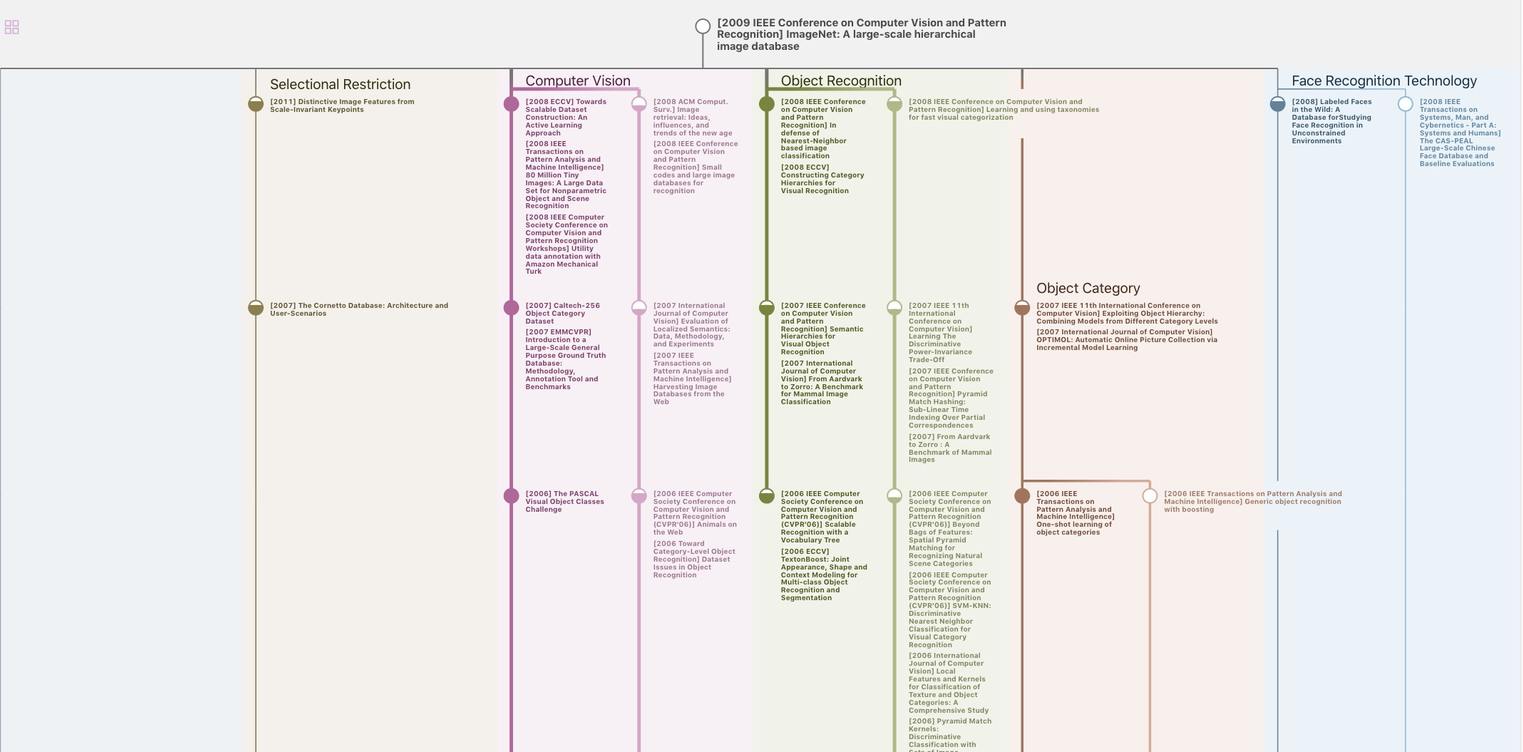
Generate MRT to find the research sequence of this paper
Chat Paper
Summary is being generated by the instructions you defined