33.1 A 74 TMACS/W CMOS-RRAM Neurosynaptic Core with Dynamically Reconfigurable Dataflow and In-situ Transposable Weights for Probabilistic Graphical Models.
ISSCC(2020)
摘要
Many powerful neural network (NN) models such as probabilistic graphical models (PGMs) and recurrent neural networks (RNNs) require flexibility in dataflow and weight access patterns as shown in Fig. 33.1.1 Typically, Compute-In-Memory (CIM) designs do not implement such dataflows or do so by replicating circuits at the memory periphery such as ADCs/neurons along both the rows and columns of the memory array, leading to an overhead in operation. This paper describes a CIM architecture implemented in a 130nm CMOS/RRAM process, that delivers the highest reported computational energy-efficiency of 74 tera-multiply-accumulates per second per watt (TMACS/W) for RRAM-based CIM architectures while simultaneously offering dataflow reconfigurability to address the limitations of previous designs. This is made possible through two key features: 1) a runtime reconfigurable dataflow with in-situ access to RRAM array and its transpose for efficient access to NN weights and 2) a voltage sensing stochastic integrate-and-fire analog neuron (Iu0026F) that is reused for correlated double sampling (CDS), stochastic voltage integration, and threshold comparison.
更多查看译文
关键词
dynamically reconfigurable dataflow,tmacs/w,cmos-rram,in-situ
AI 理解论文
溯源树
样例
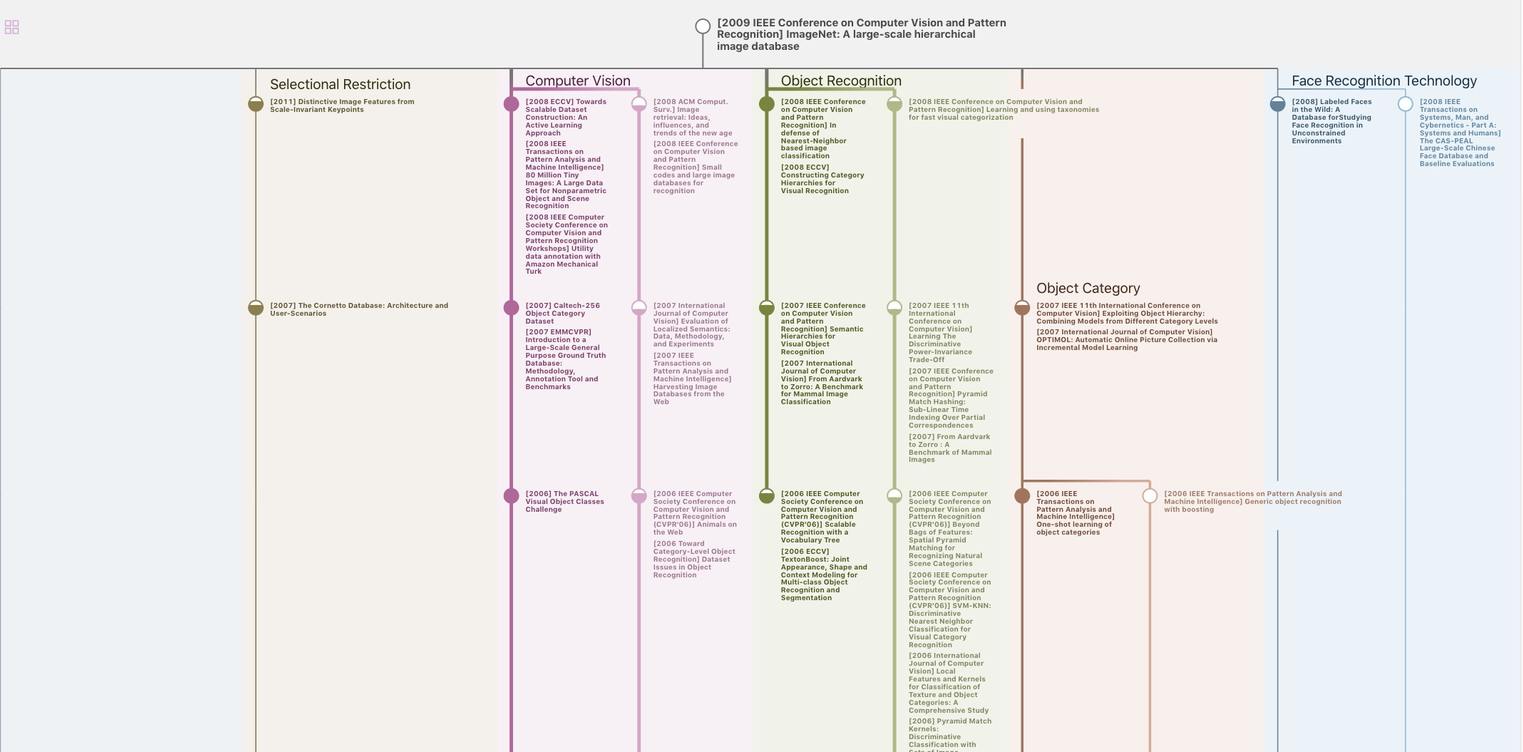
生成溯源树,研究论文发展脉络
Chat Paper
正在生成论文摘要