Modeling the short-term dynamics of in vivo excitatory spike transmission.
JOURNAL OF NEUROSCIENCE(2020)
摘要
Information transmission in neural networks is influenced by both short-term synaptic plasticity (STP) as well as nonsynaptic factors, such as after-hyperpolarization currents and changes in excitability. Although these effects have been widely characterized in vitro using intracellular recordings, how they interact in vivo is unclear. Here, we develop a statistical model of the short-term dynamics of spike transmission that aims to disentangle the contributions of synaptic and nonsynaptic effects based only on observed presynaptic and postsynaptic spiking. The model includes a dynamic functional connection with short-term plasticity as well as effects due to the recent history of postsynaptic spiking and slow changes in postsynaptic excitability. Using paired spike recordings, we find that the model accurately describes the short-term dynamics of in vivo spike transmission at a diverse set of identified and putative excitatory synapses, including a pair of connected neurons within thalamus in mouse, a thalamocortical connection in a female rabbit, and an auditory brainstem synapse in a female gerbil. We illustrate the utility of this modeling approach by showing how the spike transmission patterns captured by the model may be sufficient to account for stimulus-dependent differences in spike transmission in the auditory brainstem (end-bulb of Held). Finally, we apply this model to large-scale multielectrode recordings to illustrate how such an approach has the potential to reveal cell type-specific differences in spike transmission in vivo. Although STP parameters estimated from ongoing presynaptic and postsynaptic spiking are highly uncertain, our results are partially consistent with previous intracellular observations in these synapses.
更多查看译文
关键词
functional connectivity,generalized linear models,short-term synaptic plasticity,spiking neurons
AI 理解论文
溯源树
样例
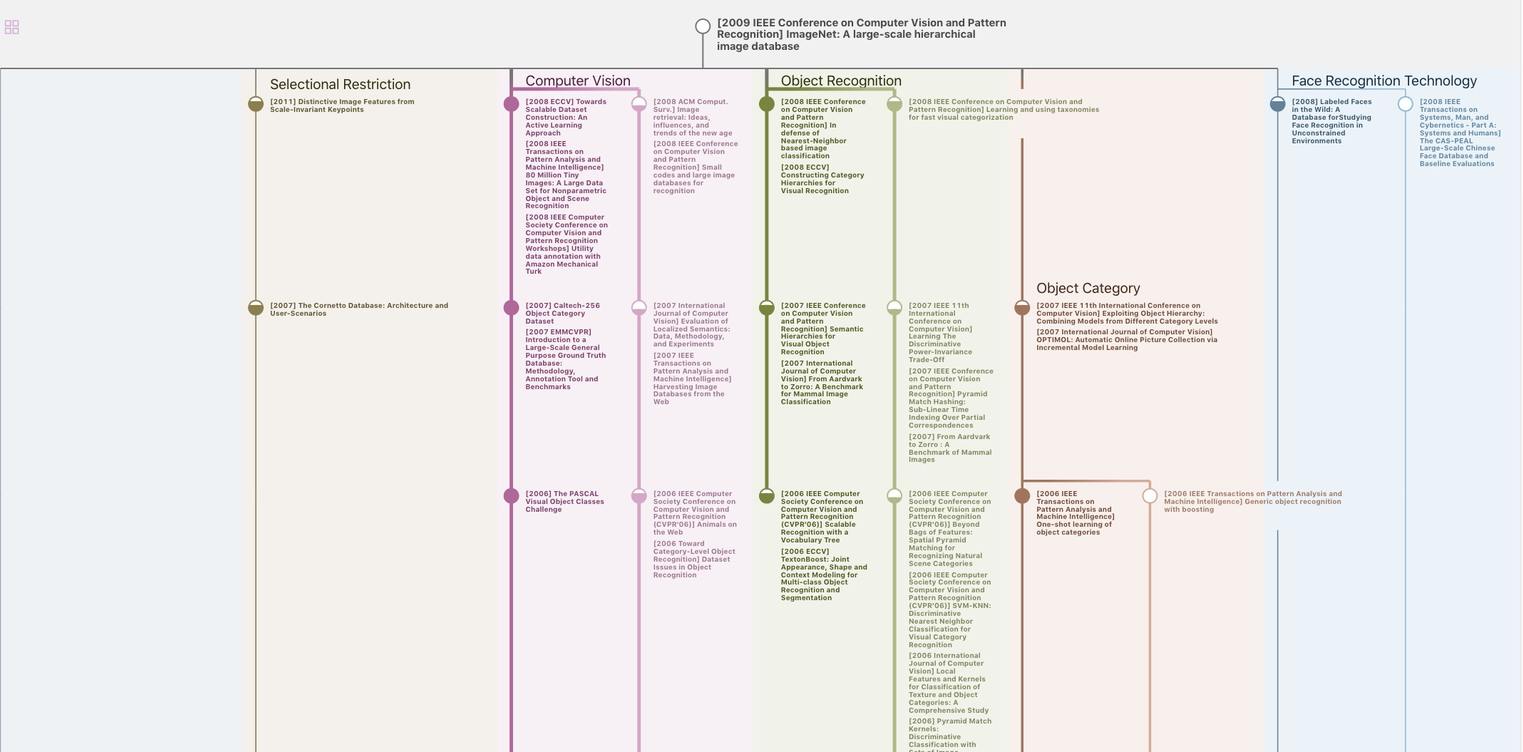
生成溯源树,研究论文发展脉络
Chat Paper
正在生成论文摘要