LEAN-LIFE: A Label-Efficient Annotation Framework Towards Learning from Explanation
meeting of the association for computational linguistics(2020)
摘要
Successfully training a deep neural network demands a huge corpus of labeled data. However, each label only provides limited information to learn from and collecting the requisite number of labels involves massive human effort. In this work, we introduce LEAN-LIFE, a web-based, Label-Efficient AnnotatioN framework for sequence labeling and classification tasks, with an easy-to-use UI that not only allows an annotator to provide the needed labels for a task, but also enables LearnIng From Explanations for each labeling decision. Such explanations enable us to generate useful additional labeled data from unlabeled instances, bolstering the pool of available training data. On three popular NLP tasks (named entity recognition, relation extraction, sentiment analysis), we find that using this enhanced supervision allows our models to surpass competitive baseline F1 scores by more than 5-10 percentage points, while using 2X times fewer labeled instances. Our framework is the first to utilize this enhanced supervision technique and does so for three important tasks -- thus providing improved annotation recommendations to users and an ability to build datasets of (data, label, explanation) triples instead of the regular (data, label) pair.
更多查看译文
关键词
learning,lean-life,label-efficient
AI 理解论文
溯源树
样例
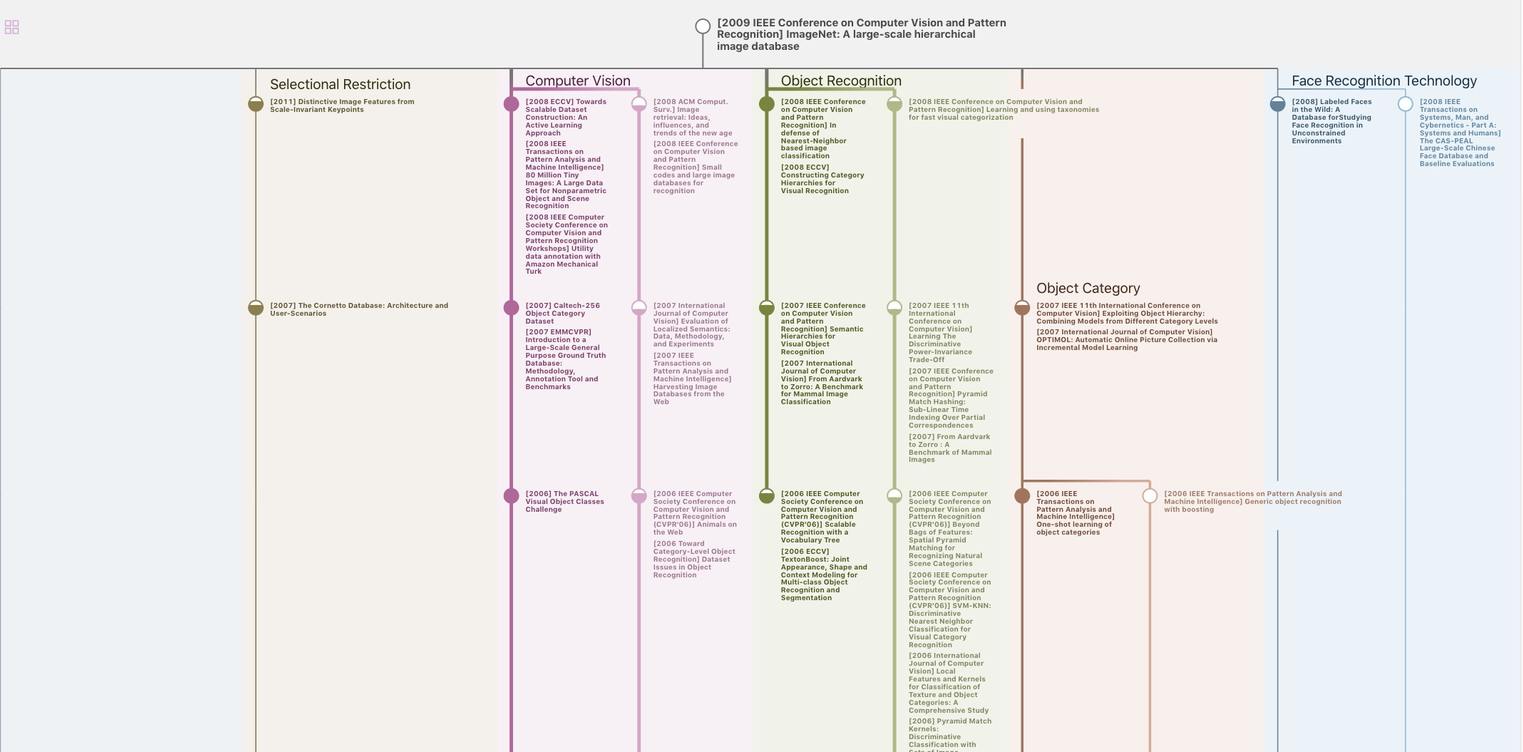
生成溯源树,研究论文发展脉络
Chat Paper
正在生成论文摘要