Poisoning Attacks on Algorithmic Fairness
MACHINE LEARNING AND KNOWLEDGE DISCOVERY IN DATABASES, ECML PKDD 2020, PT I(2021)
Abstract
Research in adversarial machine learning has shown how the performance of machine learning models can be seriously compromised by injecting even a small fraction of poisoning points into the training data. While the effects on model accuracy of such poisoning attacks have been widely studied, their potential effects on other model performance metrics remain to be evaluated. In this work, we introduce an optimization framework for poisoning attacks against algorithmic fairness, and develop a gradient-based poisoning attack aimed at introducing classification disparities among different groups in the data. We empirically show that our attack is effective not only in the white-box setting, in which the attacker has full access to the target model, but also in a more challenging black-box scenario in which the attacks are optimized against a substitute model and then transferred to the target model. We believe that our findings pave the way towards the definition of an entirely novel set of adversarial attacks targeting algorithmic fairness in different scenarios, and that investigating such vulnerabilities will help design more robust algorithms and countermeasures in the future.
MoreTranslated text
Key words
Algorithmic discrimination, Algorithmic fairness, Poisoning attacks, Adversarial machine learning, Machine learning security
AI Read Science
Must-Reading Tree
Example
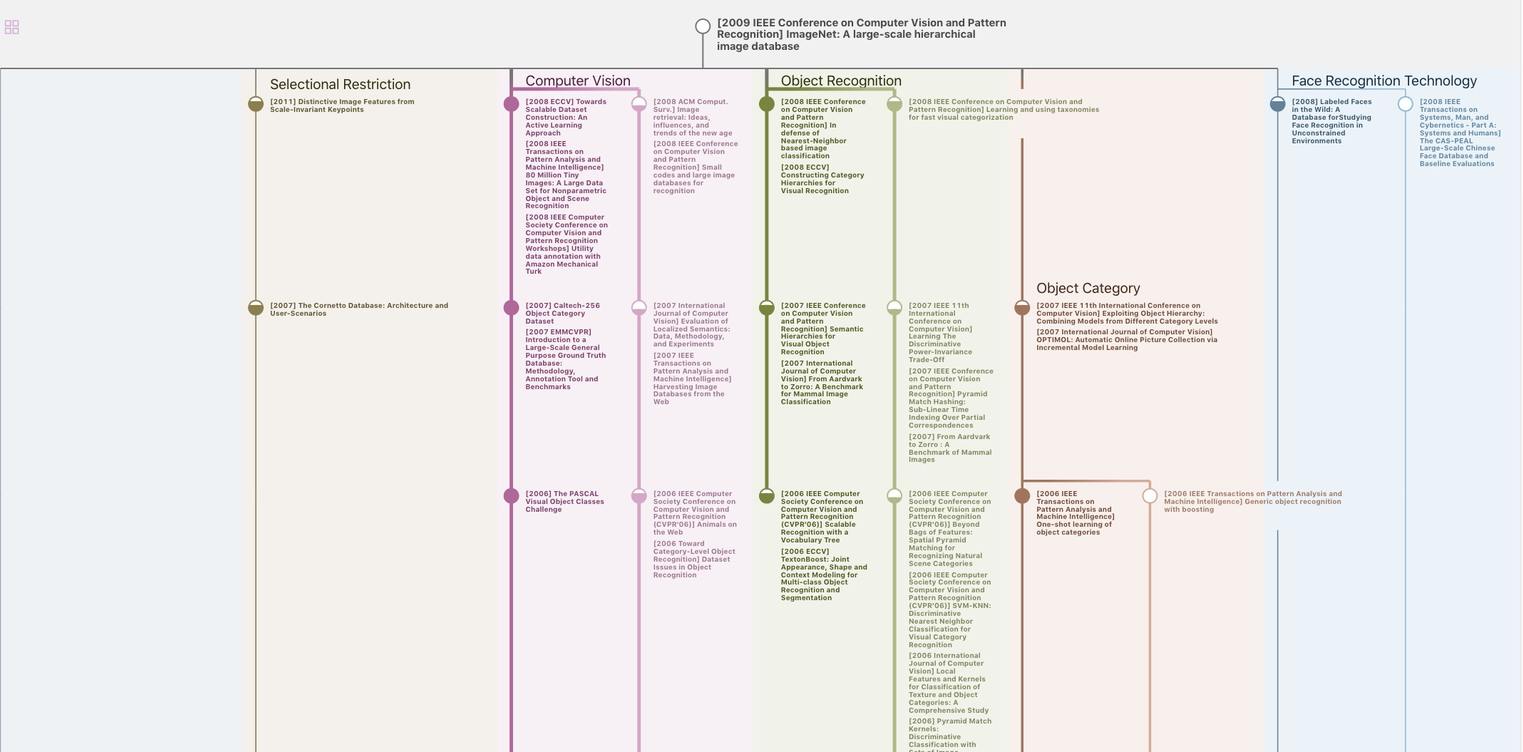
Generate MRT to find the research sequence of this paper
Chat Paper
Summary is being generated by the instructions you defined