Code-Aligned Autoencoders for Unsupervised Change Detection in Multimodal Remote Sensing Images
IEEE TRANSACTIONS ON NEURAL NETWORKS AND LEARNING SYSTEMS(2024)
摘要
Image translation with convolutional autoencoders has recently been used as an approach to multimodal change detection (CD) in bitemporal satellite images. A main challenge is the alignment of the code spaces by reducing the contribution of change pixels to the learning of the translation function. Many existing approaches train the networks by exploiting supervised information of the change areas, which, however, is not always available. We propose to extract relational pixel information captured by domain-specific affinity matrices at the input and use this to enforce alignment of the code spaces and reduce the impact of change pixels on the learning objective. A change prior is derived in an unsupervised fashion from pixel pair affinities that are comparable across domains. To achieve code space alignment, we enforce pixels with similar affinity relations in the input domains to be correlated also in code space. We demonstrate the utility of this procedure in combination with cycle consistency. The proposed approach is compared with the state-of-the-art machine learning and deep learning algorithms. Experiments conducted on four real and representative datasets show the effectiveness of our methodology.
更多查看译文
关键词
Codes,Training,Synthetic aperture radar,Deep learning,Decoding,Tensors,Remote sensing,Affinity matrix,aligned autoencoders,deep learning,heterogeneous data,image regression,multimodal image analysis,unsupervised change detection (CD)
AI 理解论文
溯源树
样例
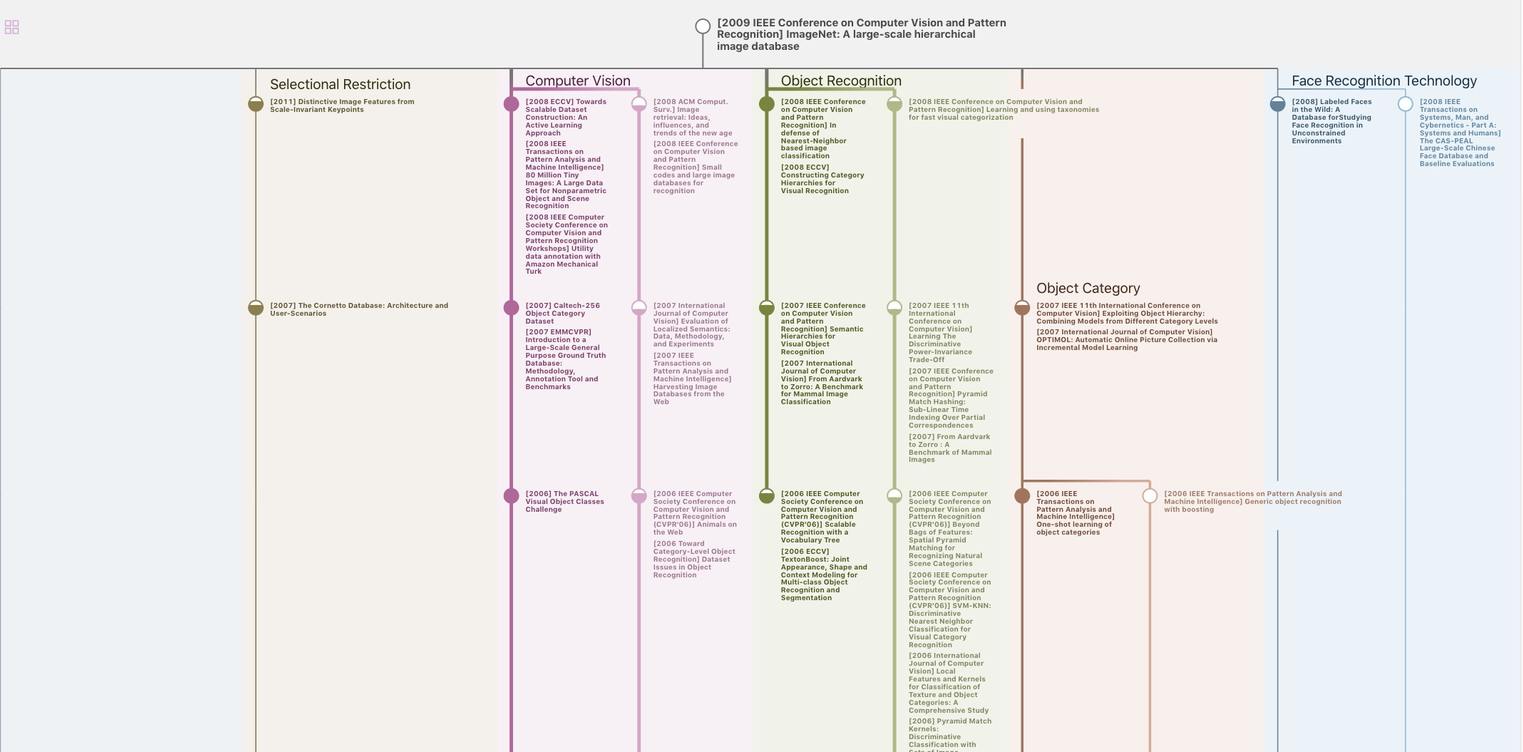
生成溯源树,研究论文发展脉络
Chat Paper
正在生成论文摘要