Extrapolation in Gridworld Markov-Decision Processes
arxiv(2020)
摘要
Extrapolation in reinforcement learning is the ability to generalize at test time given states that could never have occurred at training time. Here we consider four factors that lead to improved extrapolation in a simple Gridworld environment: (a) avoiding maximum Q-value (or other deterministic methods) for action choice at test time, (b) ego-centric representation of the Gridworld, (c) building rotational and mirror symmetry into the learning mechanism using rotational and mirror invariant convolution (rather than standard translation-invariant convolution), and (d) adding a maximum entropy term to the loss function to encourage equally good actions to be chosen equally often.
更多查看译文
关键词
extrapolation,markov-decision
AI 理解论文
溯源树
样例
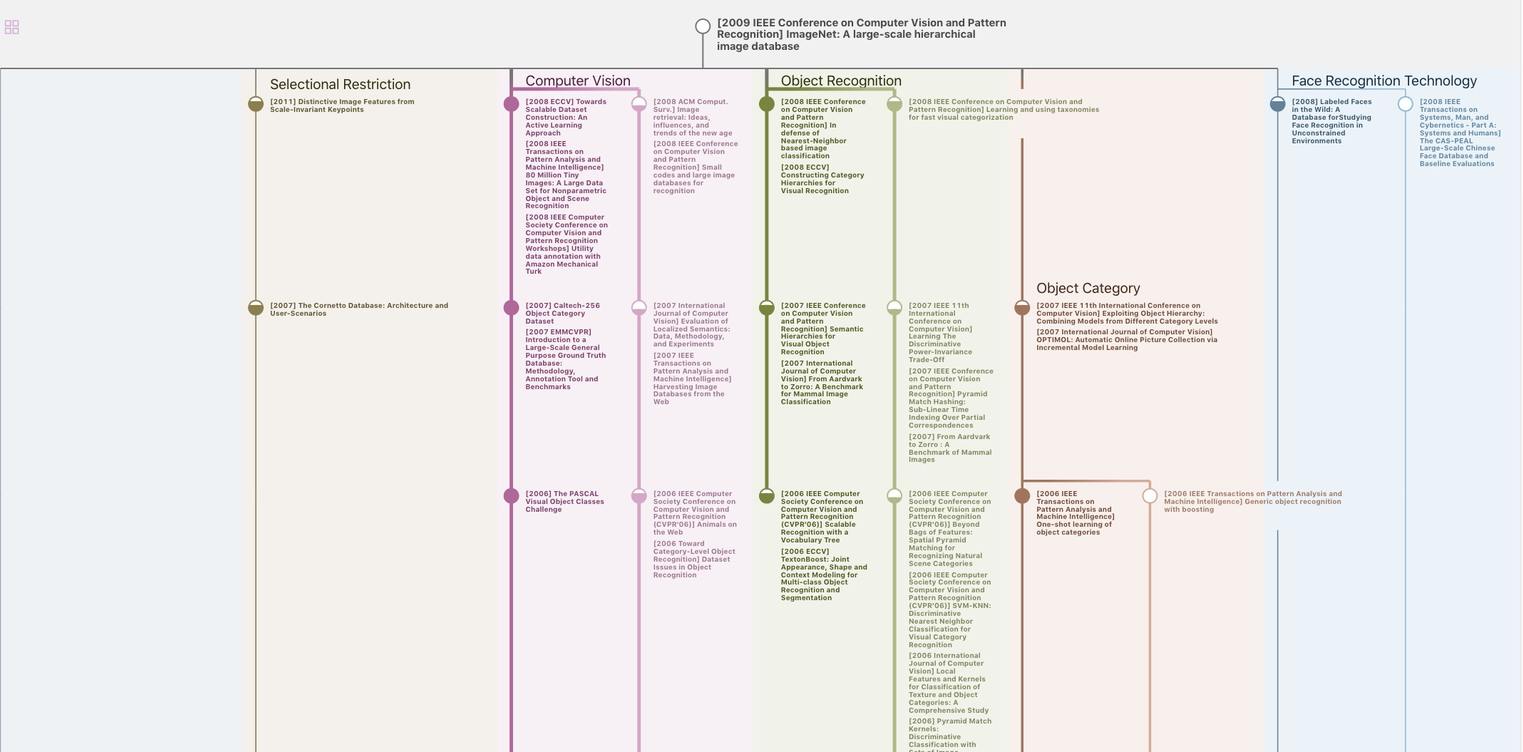
生成溯源树,研究论文发展脉络
Chat Paper
正在生成论文摘要