Analysis of The Ratio of $\ell_1$ and $\ell_2$ Norms in Compressed Sensing
arxiv(2020)
摘要
We first propose a novel criterion that guarantees that an $s$-sparse signal is the local minimizer of the $\ell_1/\ell_2$ objective; our criterion is interpretable and useful in practice. We also give the first uniform recovery condition using a geometric characterization of the null space of the measurement matrix, and show that this condition is easily satisfied for a class of random matrices. We also present analysis on the stability of the procedure when noise pollutes data. Numerical experiments are provided that compare $\ell_1/\ell_2$ with some other popular non-convex methods in compressed sensing. Finally, we propose a novel initialization approach to accelerate the numerical optimization procedure. We call this initialization approach \emph{support selection}, and we demonstrate that it empirically improves the performance of existing $\ell_1/\ell_2$ algorithms.
更多查看译文
关键词
compressed
AI 理解论文
溯源树
样例
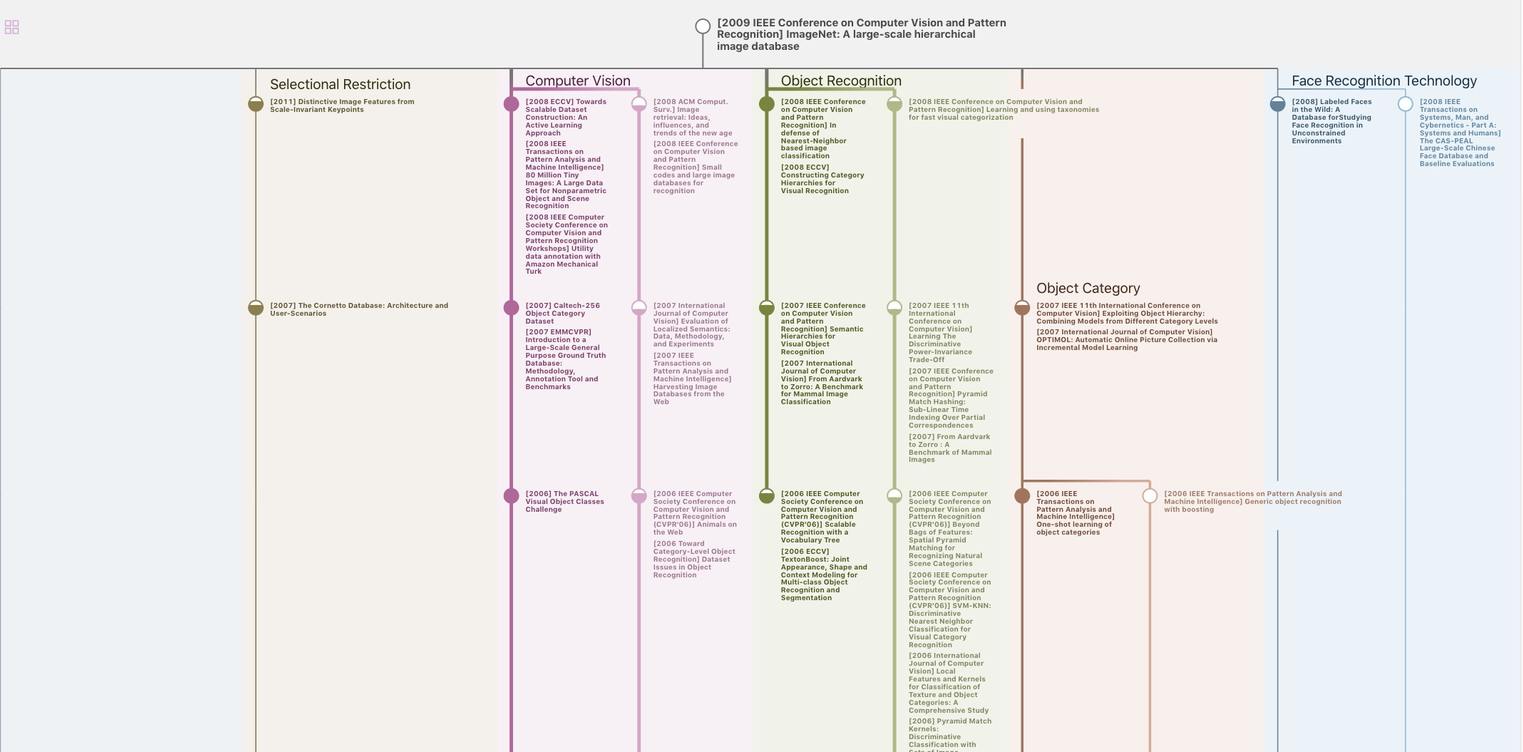
生成溯源树,研究论文发展脉络
Chat Paper
正在生成论文摘要