On the Language Neutrality of Pre-trained Multilingual Representations
EMNLP(2020)
摘要
Multilingual contextual embeddings, such as multilingual BERT (mBERT) and XLM-RoBERTa, have proved useful for many multi-lingual tasks. Previous work probed the cross-linguality of the representations indirectly using zero-shot transfer learning on morphological and syntactic tasks. We instead focus on the language-neutrality of mBERT with respect to lexical semantics. Our results show that contextual embeddings are more language-neutral and in general more informative than aligned static word-type embeddings which are explicitly trained for language neutrality. Contextual embeddings are still by default only moderately language-neutral, however, we show two simple methods for achieving stronger language neutrality: first, by unsupervised centering of the representation for languages, and second by fitting an explicit projection on small parallel data. In addition, we show how to reach state-of-the-art accuracy on language identification and word alignment in parallel sentences.
更多查看译文
关键词
language neutrality,representations,pre-trained
AI 理解论文
溯源树
样例
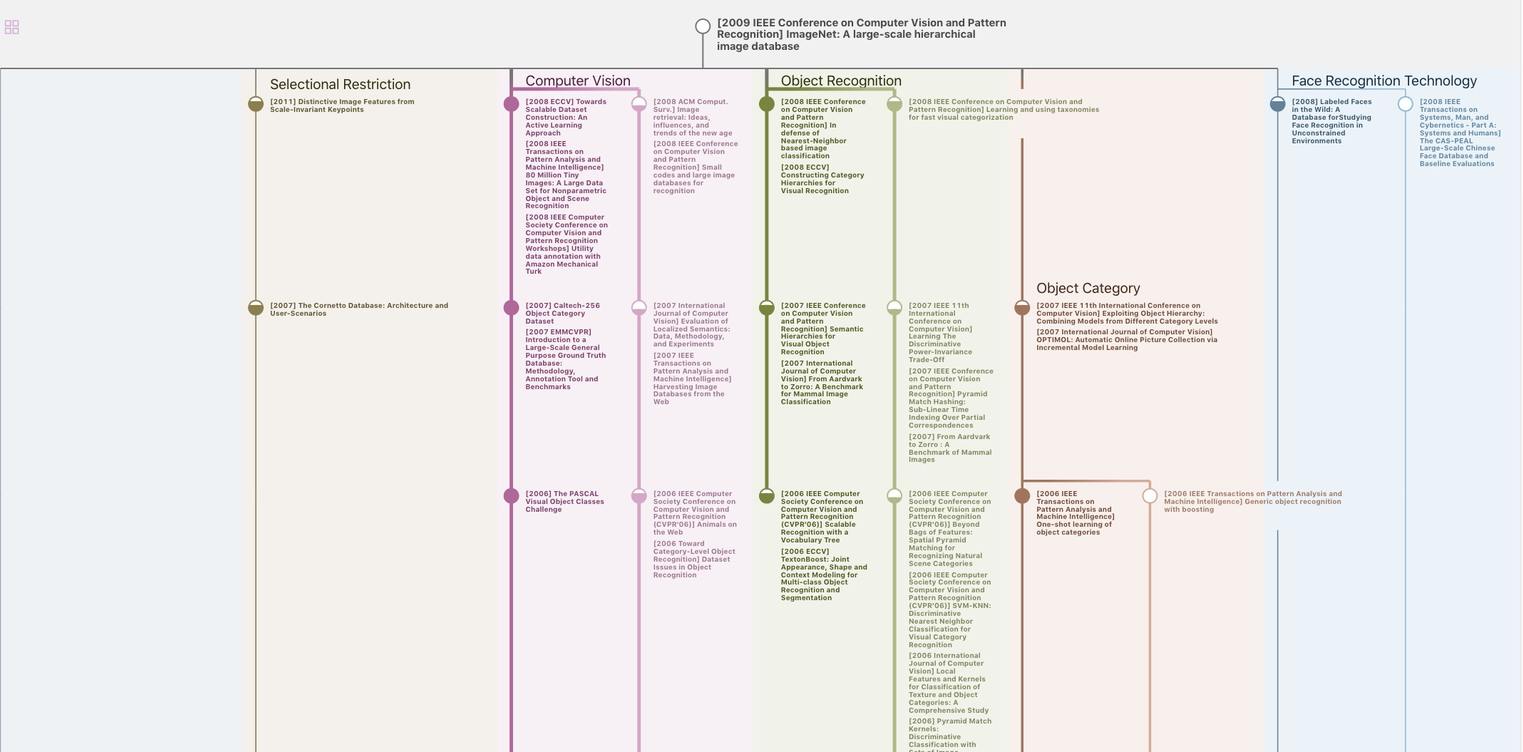
生成溯源树,研究论文发展脉络
Chat Paper
正在生成论文摘要