MA 3 : Model Agnostic Adversarial Augmentation for Few Shot learning
arxiv(2020)
摘要
Despite the recent developments in vision-related problems using deep neural networks, there still remains a wide scope in the improvement of generalizing these models to unseen examples. In this paper, we explore the domain of few-shot learning with a novel augmentation technique. In contrast to other generative augmentation techniques, where the distribution over input images are learnt, we propose to learn the probability distribution over the image transformation parameters which are easier and quicker to learn. Our technique is fully differentiable which enables its extension to versatile data-sets and base models. We evaluate our proposed method on multiple base-networks and 2 data-sets to establish the robustness and efficiency of this method. We obtain an improvement of nearly 4% by adding our augmentation module without making any change in network architectures. We also make the code readily available for usage by the community.
更多查看译文
关键词
model agnostic adversarial augmentation,vision-related problems,deep neural networks,unseen examples,few-shot learning,augmentation technique,generative augmentation techniques,input images,probability distribution,image transformation parameters,versatile data-sets,base models,multiple base-networks,augmentation module,network architectures
AI 理解论文
溯源树
样例
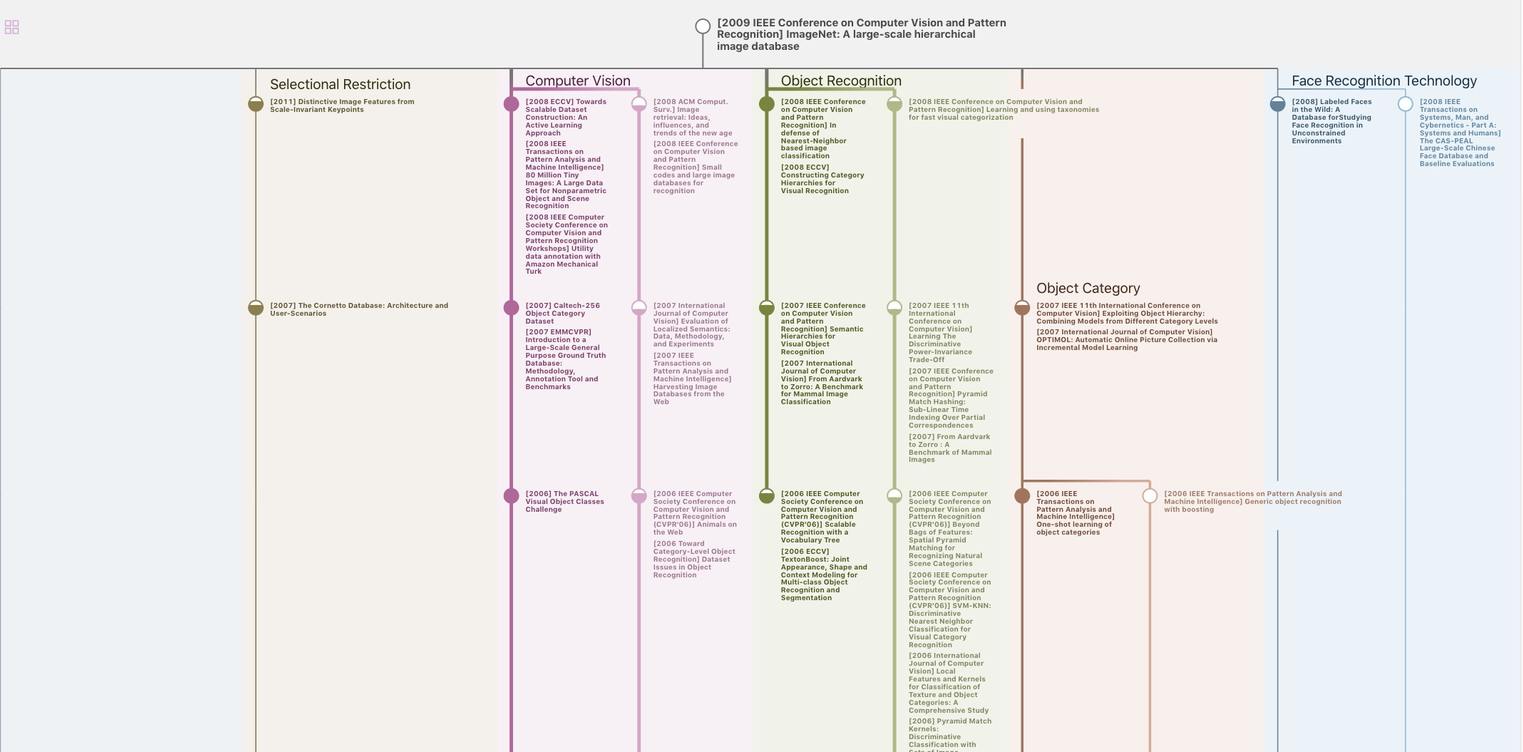
生成溯源树,研究论文发展脉络
Chat Paper
正在生成论文摘要