Scalable Multilingual Frontend for TTS
2020 IEEE INTERNATIONAL CONFERENCE ON ACOUSTICS, SPEECH, AND SIGNAL PROCESSING(2020)
摘要
This paper describes progress towards making a Neural Text-to-Speech (TTS) Frontend that works for many languages and can be easily extended to new languages. We take a Machine Translation (MT) inspired approach to constructing the frontend, and model both text normalization and pronunciation on a sentence level by building and using sequence-to-sequence (S2S) models. We experimented with training normalization and pronunciation as separate S2S models and with training a single S2S model combining both functions. For our language-independent approach to pronunciation we do not use a lexicon. Instead all pronunciations, including context-based pronunciations, are captured in the S2S model. We also present a language-independent chunking and splicing technique that allows us to process arbitrary-length sentences. Models for 18 languages were trained and evaluated. Many of the accuracy measurements are above 99%. We also evaluated the models in the context of end-to-end synthesis against our current production system.
更多查看译文
关键词
speech synthesis, machine learning
AI 理解论文
溯源树
样例
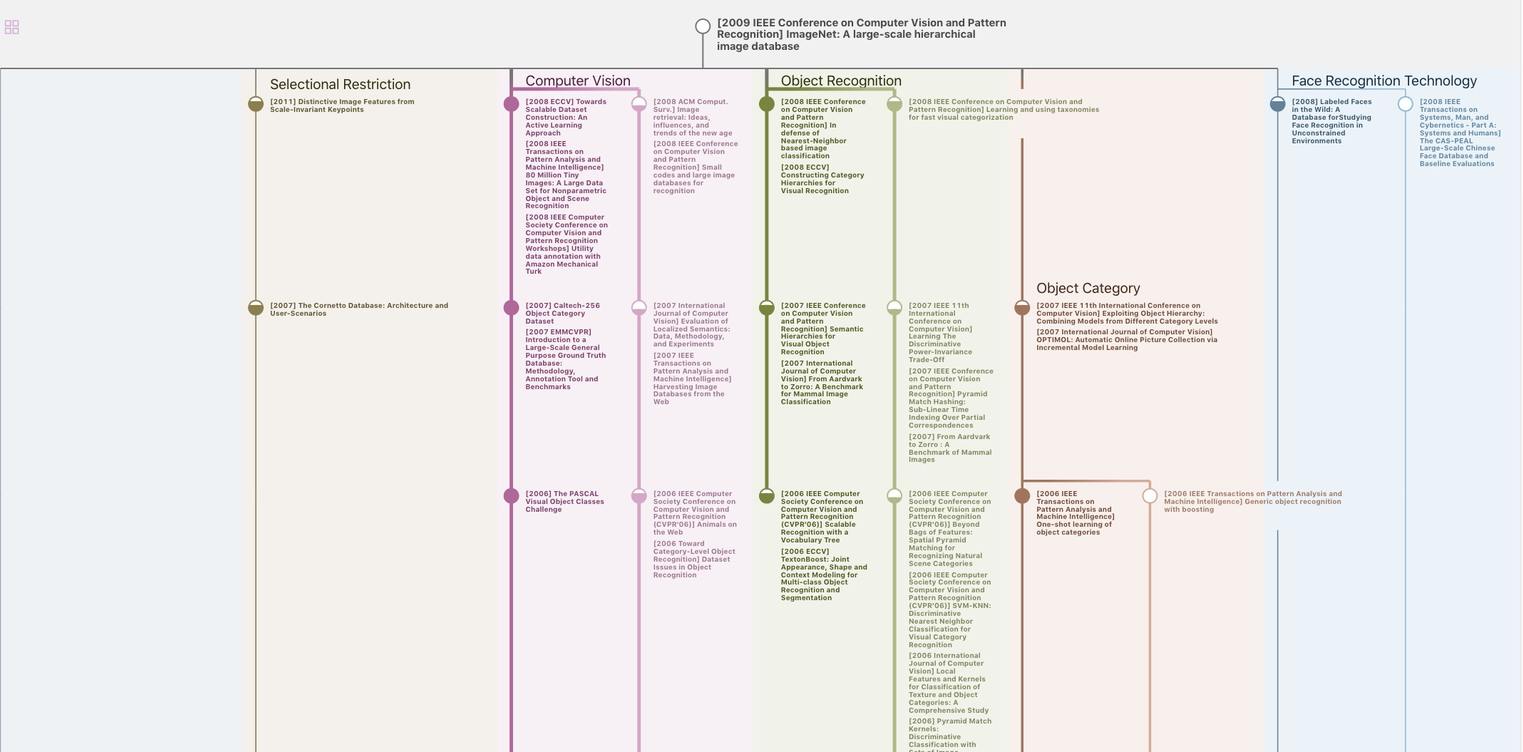
生成溯源树,研究论文发展脉络
Chat Paper
正在生成论文摘要