Robust Bregman Clustering
ANNALS OF STATISTICS(2021)
Abstract
Clustering with Bregman divergences encompasses a wide family of clustering procedures that are well suited to mixtures of distributions from exponential families (J. Mach. Learn. Res. 6 (2005) 1705-1749). However, these techniques are highly sensitive to noise. To address the issue of clustering data with possibly adversarial noise, we introduce a robustified version of Bregman clustering based on a trimming approach. We investigate its theoretical properties, showing for instance that our estimator converges at a sub-Gaussian rate 1/root n, where n denotes the sample size, under mild tail assumptions. We also show that it is robust to a certain amount of noise, stated in terms of breakdown point. We also derive a Lloyd-type algorithm with a trimming parameter, along with a heuristic to select this parameter and the number of clusters from sample. Some numerical experiments assess the performance of our method on simulated and real datasets.
MoreTranslated text
Key words
Clustering, Bregman divergence, robustness
AI Read Science
Must-Reading Tree
Example
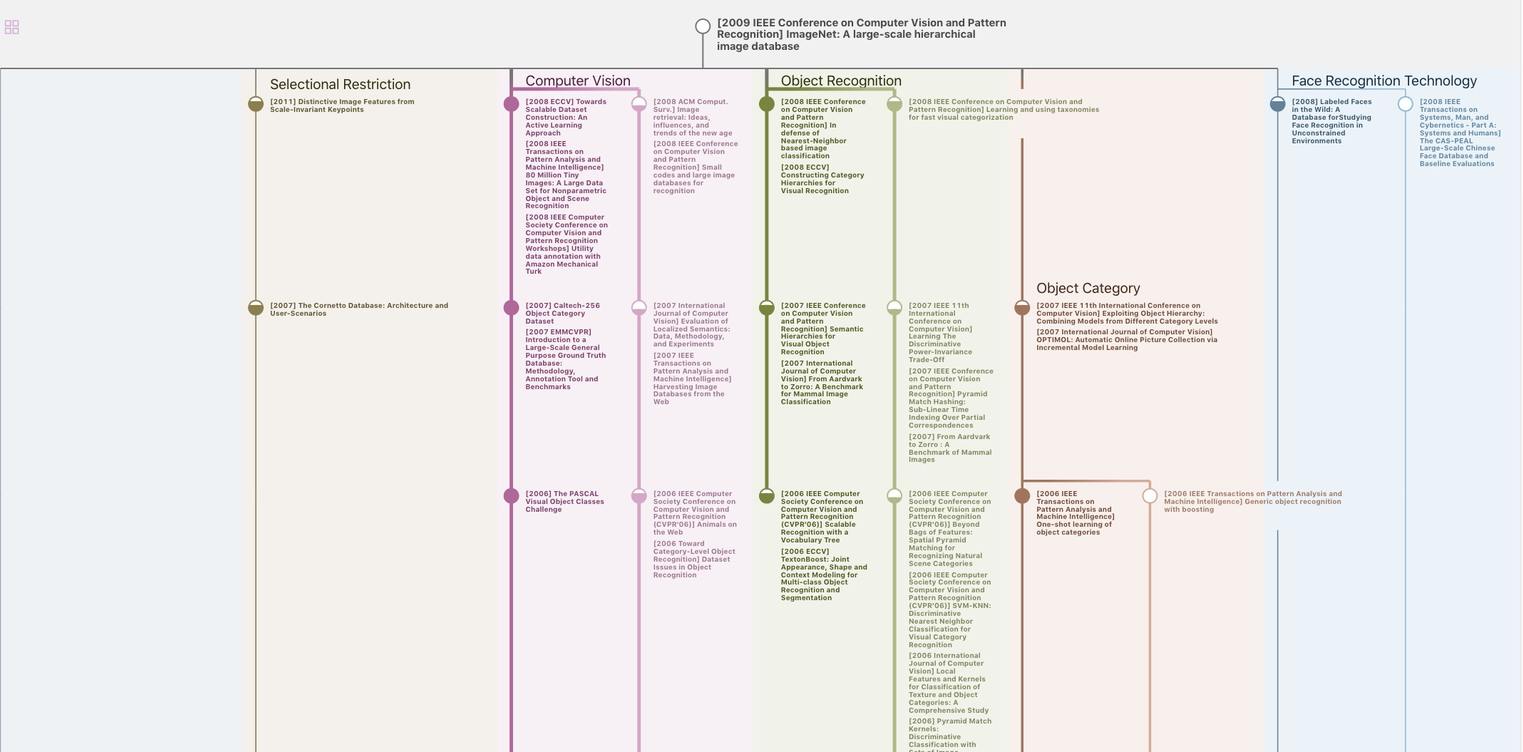
Generate MRT to find the research sequence of this paper
Chat Paper
Summary is being generated by the instructions you defined