Learning Cognitive Map Representations for Navigation by Sensory-Motor Integration.
IEEE Transactions on Cybernetics(2022)
摘要
How to transform a mixed flow of sensory and motor information into memory state of self-location and to build map representations of the environment are central questions in the navigation research. Studies in neuroscience have shown that place cells in the hippocampus of the rodent brains form dynamic cognitive representations of locations in the environment. We propose a neural-network model called sensory-motor integration network model (SeMINet) to learn cognitive map representations by integrating sensory and motor information while an agent is exploring a virtual environment. This biologically inspired model consists of a deep neural network representing visual features of the environment, a recurrent network of place units encoding spatial information by sensorimotor integration, and a secondary network to decode the locations of the agent from spatial representations. The recurrent connections between the place units sustain an activity bump in the network without the need of sensory inputs, and the asymmetry in the connections propagates the activity bump in the network, forming a dynamic memory state which matches the motion of the agent. A competitive learning process establishes the association between the sensory representations and the memory state of the place units, and is able to correct the cumulative path-integration errors. The simulation results demonstrate that the network forms neural codes that convey location information of the agent independent of its head direction. The decoding network reliably predicts the location even when the movement is subject to noise. The proposed SeMINet thus provides a brain-inspired neural-network model for cognitive map updated by both self-motion cues and visual cues.
更多查看译文
关键词
Visualization,Navigation,Robot sensing systems,Brain modeling,Hippocampus,Biological system modeling
AI 理解论文
溯源树
样例
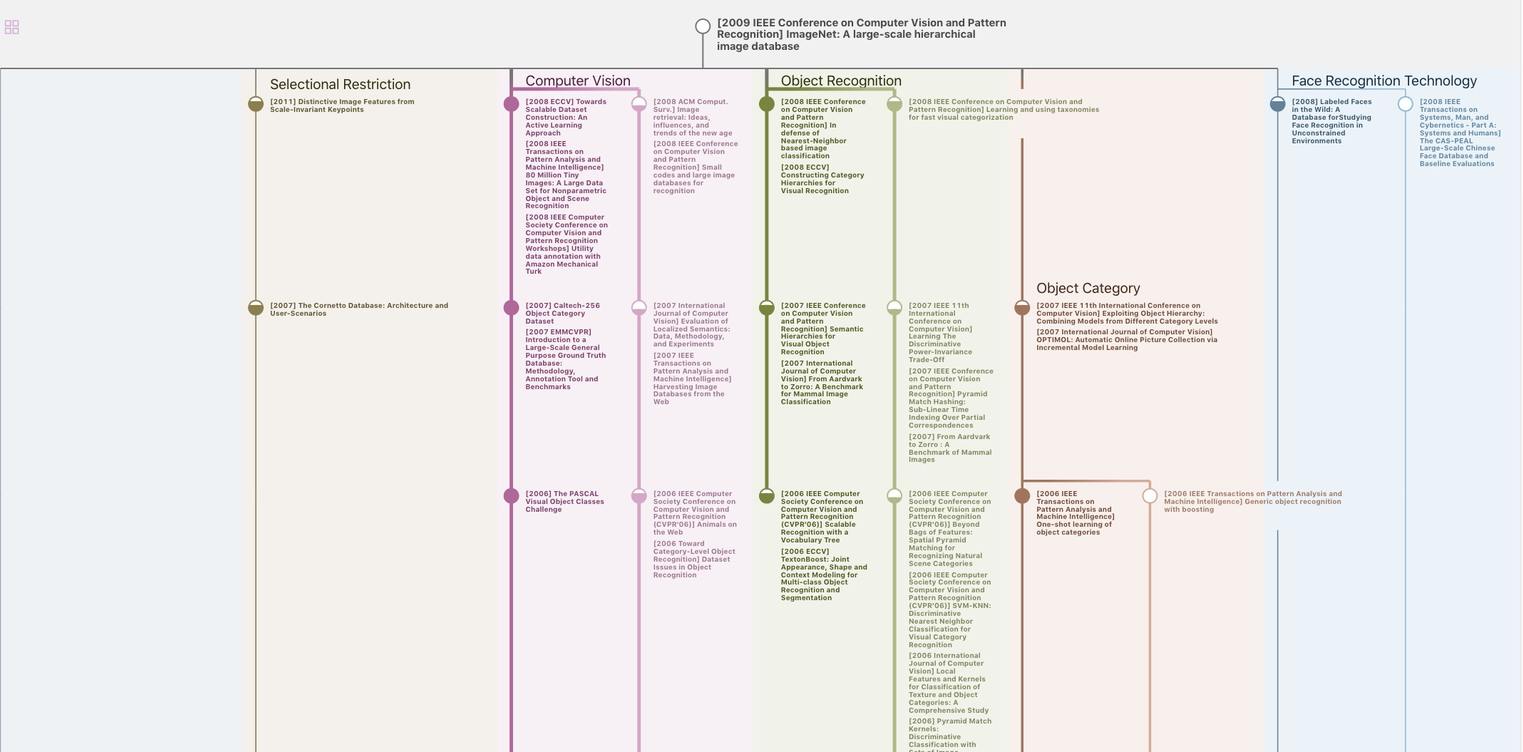
生成溯源树,研究论文发展脉络
Chat Paper
正在生成论文摘要