MFDC-net: Multifeature-based deep convolutional neural network for single image haze removal
CONCURRENCY AND COMPUTATION-PRACTICE & EXPERIENCE(2020)
摘要
When it comes to the dehazing method based on the ambient light scattering model, there are ill-conditioning problems of great concern. Therefore, the inaccurate estimation of the global atmospheric light and transmittance can bring about hue error phenomena, halo artifacts, and image noises. In this paper, we present an effective and efficient approach to solve above problems. Firstly, we adopt single-threshold segmentation method and quad-tree algorithms, which can break through the limitation that the dark channel prior theory (DCP) is not suitable for sky region, to quickly and accurately locate and estimate the global atmospheric light. Secondly, we design a new deep convolutional network architecture, which can effectively suppress the halo effect of image edges and restrain image noises, to optimize transmittance. Numerous of outdoor and synthesis haze image test results indicate that the optimized approach could shorten the processing time greatly. Besides, with more accurate global atmospheric light value and transmittance, the image noise and the halo artifact can be effectively suppressed and removed respectively, the hue error phenomenon improved, and the image contrast significantly enhanced in the dehazing results.
更多查看译文
关键词
convolutional network,ill-conditioning problem,quad-tree,single-threshold,transmittance
AI 理解论文
溯源树
样例
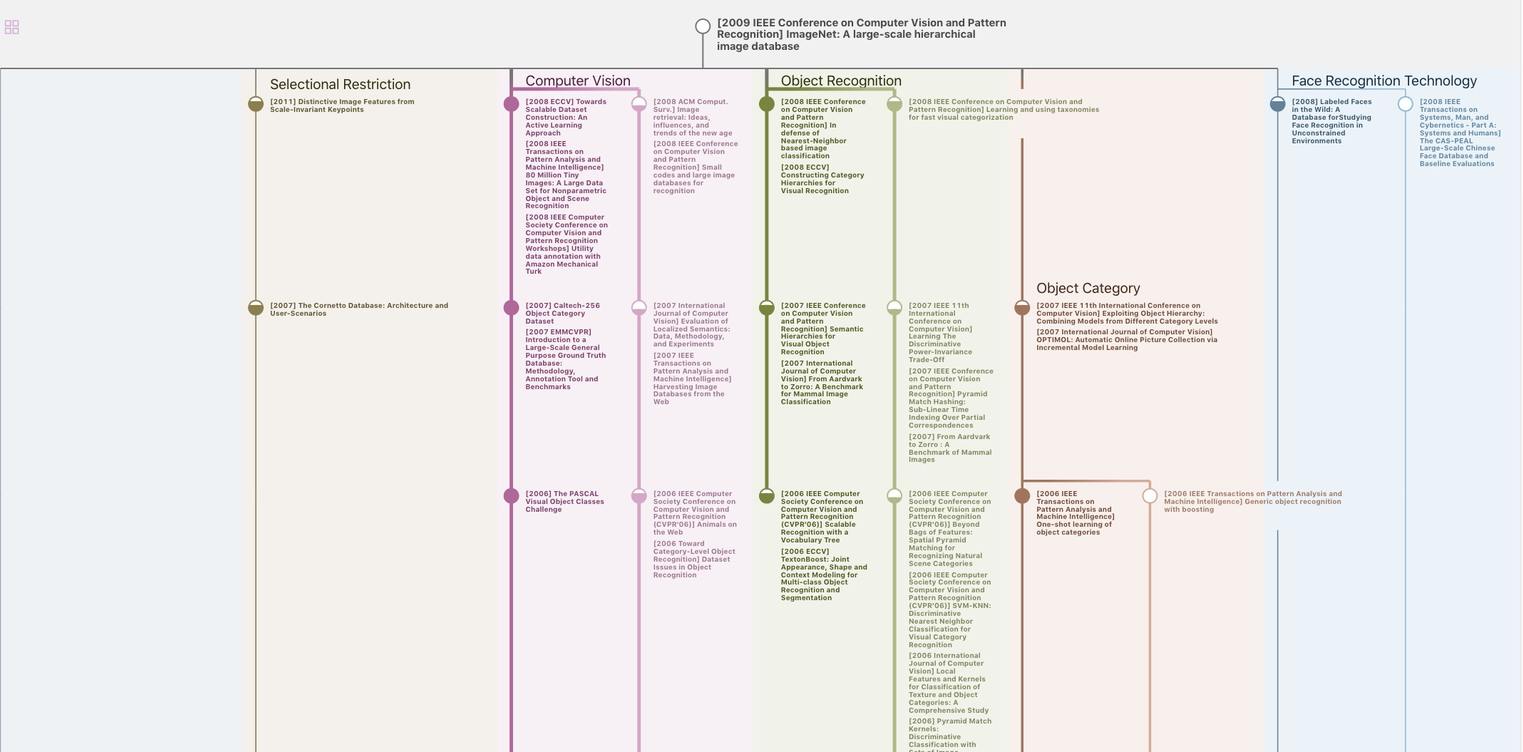
生成溯源树,研究论文发展脉络
Chat Paper
正在生成论文摘要