The Impact of Pan-Sharpening and Spectral Resolution on Vineyard Segmentation through Machine Learning.
REMOTE SENSING(2020)
摘要
Precision viticulture benefits from the accurate detection of vineyard vegetation from remote sensing, without a priori knowledge of vine locations. Vineyard detection enables efficient, and potentially automated, derivation of spatial measures such as length and area of crop, and hence required volumes of water, fertilizer, and other resources. Machine learning techniques have provided significant advancements in recent years in the areas of image segmentation, classification, and object detection, with neural networks shown to perform well in the detection of vineyards and other crops. However, what has not been extensively quantitatively examined is the extent to which the initial choice of input imagery impacts detection/segmentation accuracy. Here, we use a standard deep convolutional neural network (CNN) to detect and segment vineyards across Australia using DigitalGlobe Worldview-2 images at similar to 50 cm (panchromatic) and similar to 2 m (multispectral) spatial resolution. A quantitative assessment of the variation in model performance with input parameters during model training is presented from a remote sensing perspective, with combinations of panchromatic, multispectral, pan-sharpened multispectral, and the spectral Normalised Difference Vegetation Index (NDVI) considered. The impact of image acquisition parameters-namely, the off-nadir angle and solar elevation angle-on the quality of pan-sharpening is also assessed. The results are synthesised into a 'recipe' for optimising the accuracy of vineyard segmentation, which can provide a guide to others aiming to implement or improve automated crop detection and classification.
更多查看译文
关键词
precision viticulture,machine learning,pan-sharpening,image fusion,deep learning,semantic segmentation
AI 理解论文
溯源树
样例
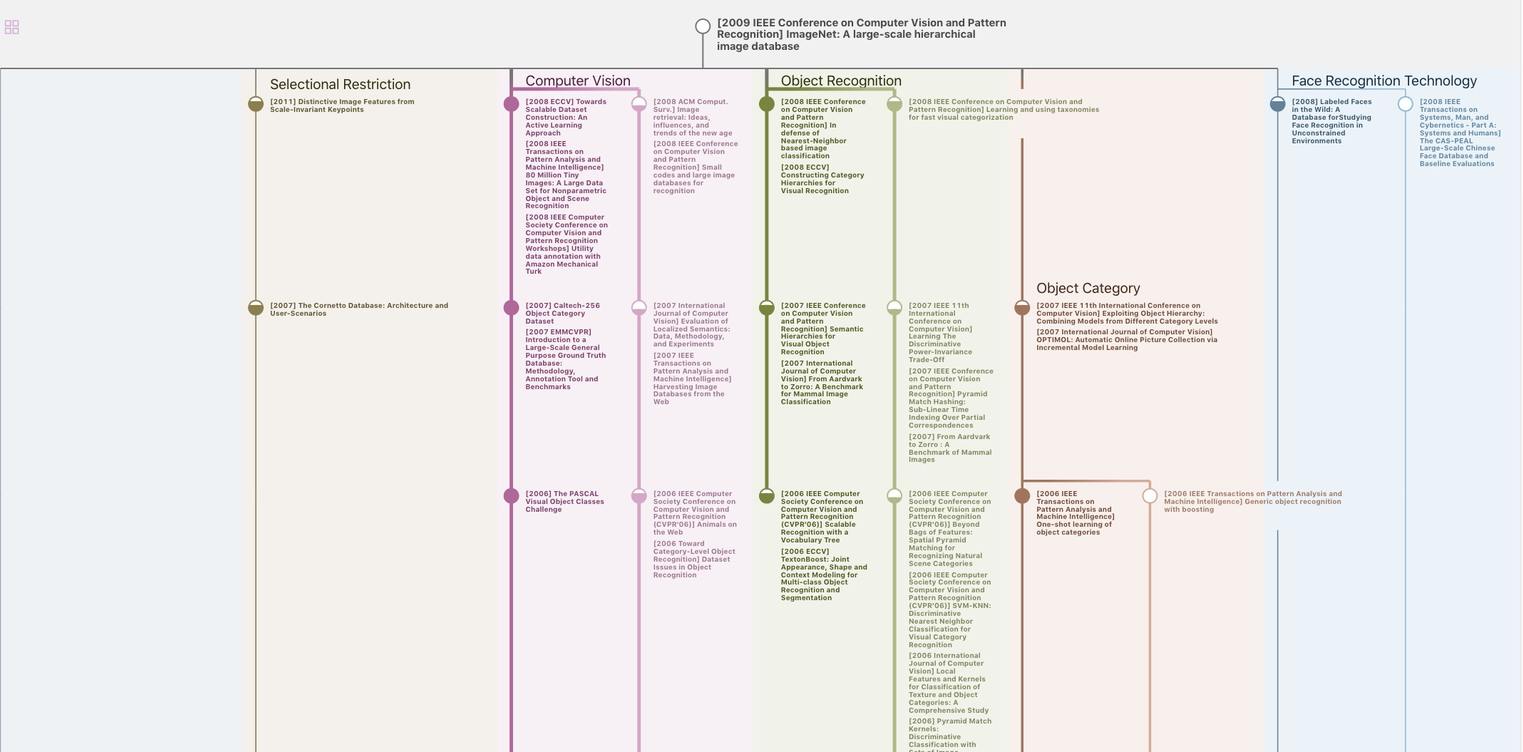
生成溯源树,研究论文发展脉络
Chat Paper
正在生成论文摘要