TypeNet: Scaling up Keystroke Biometrics
arxiv(2020)
摘要
We study the suitability of keystroke dynamics to authenticate 100K users typing free-text. For this, we first analyze to what extent our method based on a Siamese Recurrent Neural Network (RNN) is able to authenticate users when the amount of data per user is scarce, a common scenario in free-text keystroke authentication. With 1K users for testing the network, a population size comparable to previous works, TypeNet obtains an equal error rate of 4.8% using only 5 enrollment sequences and 1 test sequence per user with 50 keystrokes per sequence. Using the same amount of data per user, as the number of test users is scaled up to 100K, the performance in comparison to 1K decays relatively by less than 5%, demonstrating the potential of TypeNet to scale well at large scale number of users. Our experiments are conducted with the Aalto University keystroke database. To the best of our knowledge, this is the largest free-text keystroke database captured with more than 136M keystrokes from 168K users.
更多查看译文
关键词
keystroke biometrics,keystroke dynamics,Siamese recurrent neural network,free-text keystroke authentication,TypeNet,enrollment sequences,test sequence,free-text keystroke database
AI 理解论文
溯源树
样例
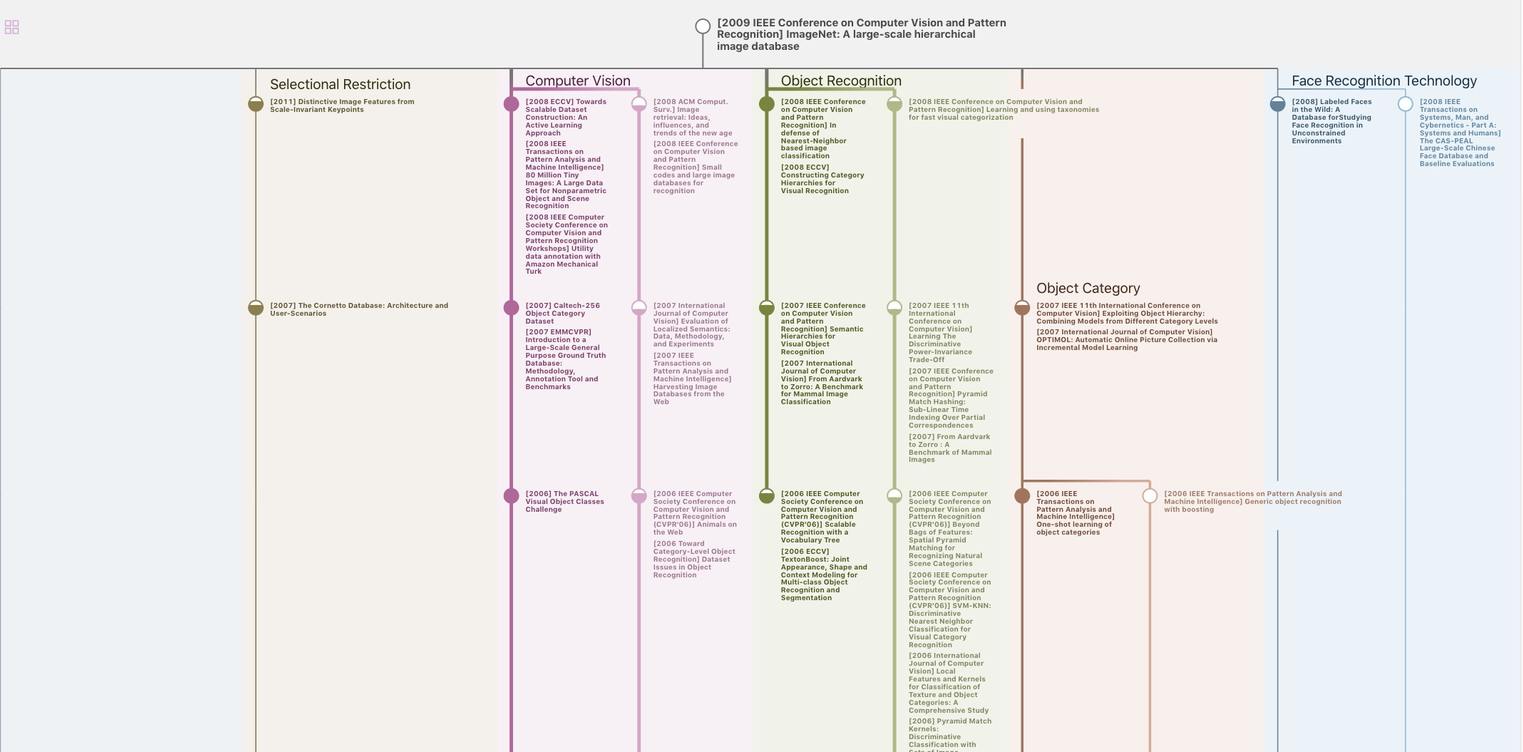
生成溯源树,研究论文发展脉络
Chat Paper
正在生成论文摘要