Development of Deep-learning Segmentation for Breast Cancer in MR Images based on Neural Network Convolution.
ICCPR(2019)
摘要
In this study, we proposed a deep-learning-based semantic segmentation of breast tumors in diagnostic breast MRI, which had been planned with chemotherapy treatment, for extracting imaging biomarkers in radiomics/radiogenomics studies. Deep-learning neural network convolution (NNC) that employs patched-based neural network regression in a convolutional manner were employed to output a map of the likelihood of being a tumor. We trained our NNC with three dynamic contrast-enhanced MR images as input: pre-contrast, early-phase, and a subtraction image between the two. Corresponding "teaching" images were obtained from "gold-standard" tumor regions that were manually segmented by an experienced radiologist. To evaluate the performance, we compared our method with non-deep-learning region-growing and watershed segmentation algorithms on 118 MRI studies in 4-fold cross-validation. Average Dice coefficients of our NNC, the region-growing, and watershed segmentation algorithms were 0.724, 0.643, and 0.540, respectively. The performance of our NNC was superior to that of the conventional methods with statistical significance. Thus, our NNC semantic segmentation would be a valuable tool for providing accurate tumor segmentation in extracting imaging biomarkers in radiomics/radiogenomics studies.
更多查看译文
AI 理解论文
溯源树
样例
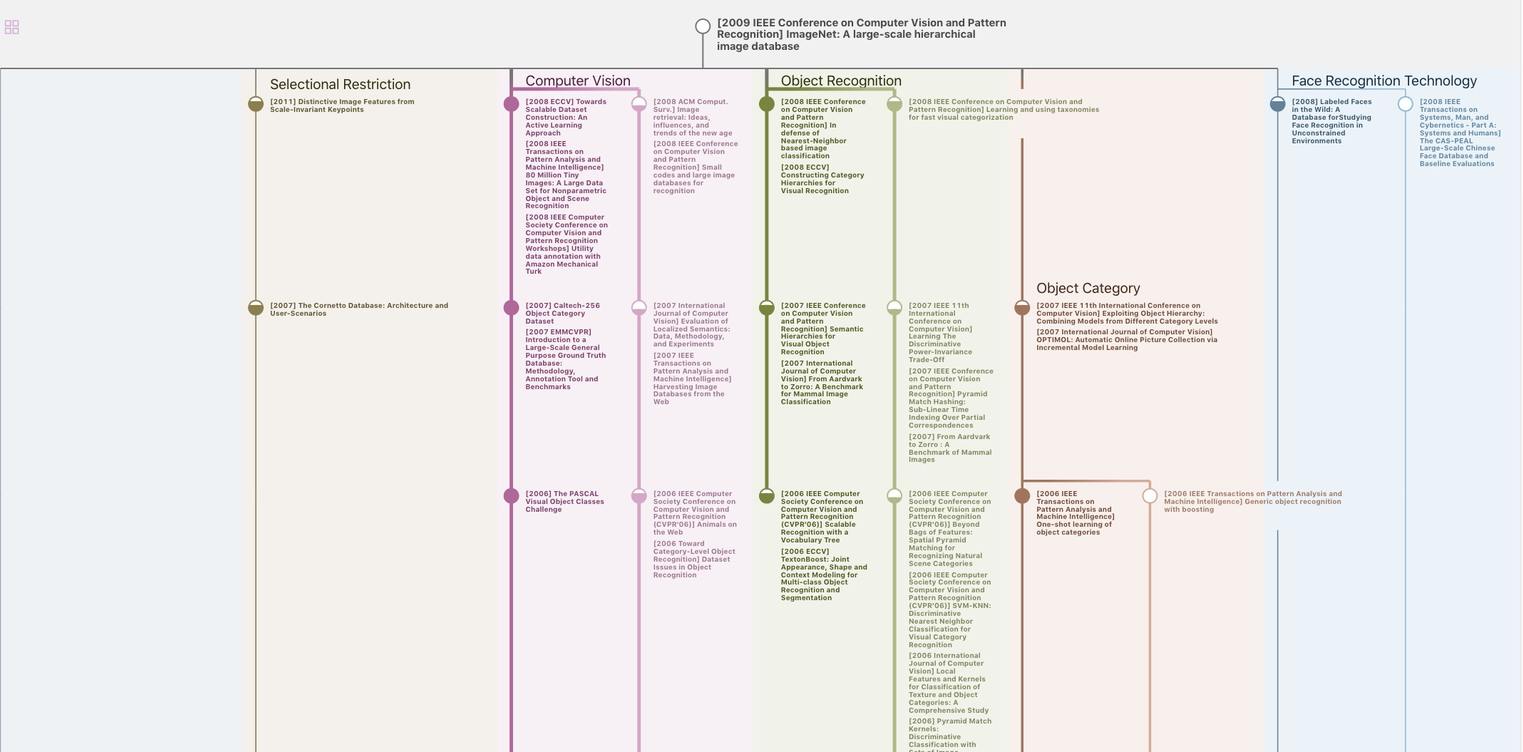
生成溯源树,研究论文发展脉络
Chat Paper
正在生成论文摘要