Intrusion Detection Using Convolutional Recurrent Neural Network.
ICCPR(2019)
摘要
There are two main problems in the current abnormal network traffic monitoring methods: feature dependence and low accuracy. To solve these problems, we propose a novel deep learning model OCL based on network traffic in this paper. Our OCL model consists of one-dimensional convolutional neural network and long short-term memory. Convolutional neural network is first used to extract features from the image representation of original network traffics. Secondly, LSTM is used to combine the extracted features over time from the obtained features via convolutional and pooling operation. Finally, the output of the LSTM model is fed to fully connection network for classification. End-to-end representation learning is adopted to automatically learn the key features of network traffics. This can describe the network traffic behavior better and improve the ability of anomaly detection effectively. To verify the effectiveness of OCL model, it is comprehensively evaluated on the NSL-KDD dataset and get the best results. Experimental results show that the OCL model achieves high accuracy, which has better performance than other detection methods.
更多查看译文
AI 理解论文
溯源树
样例
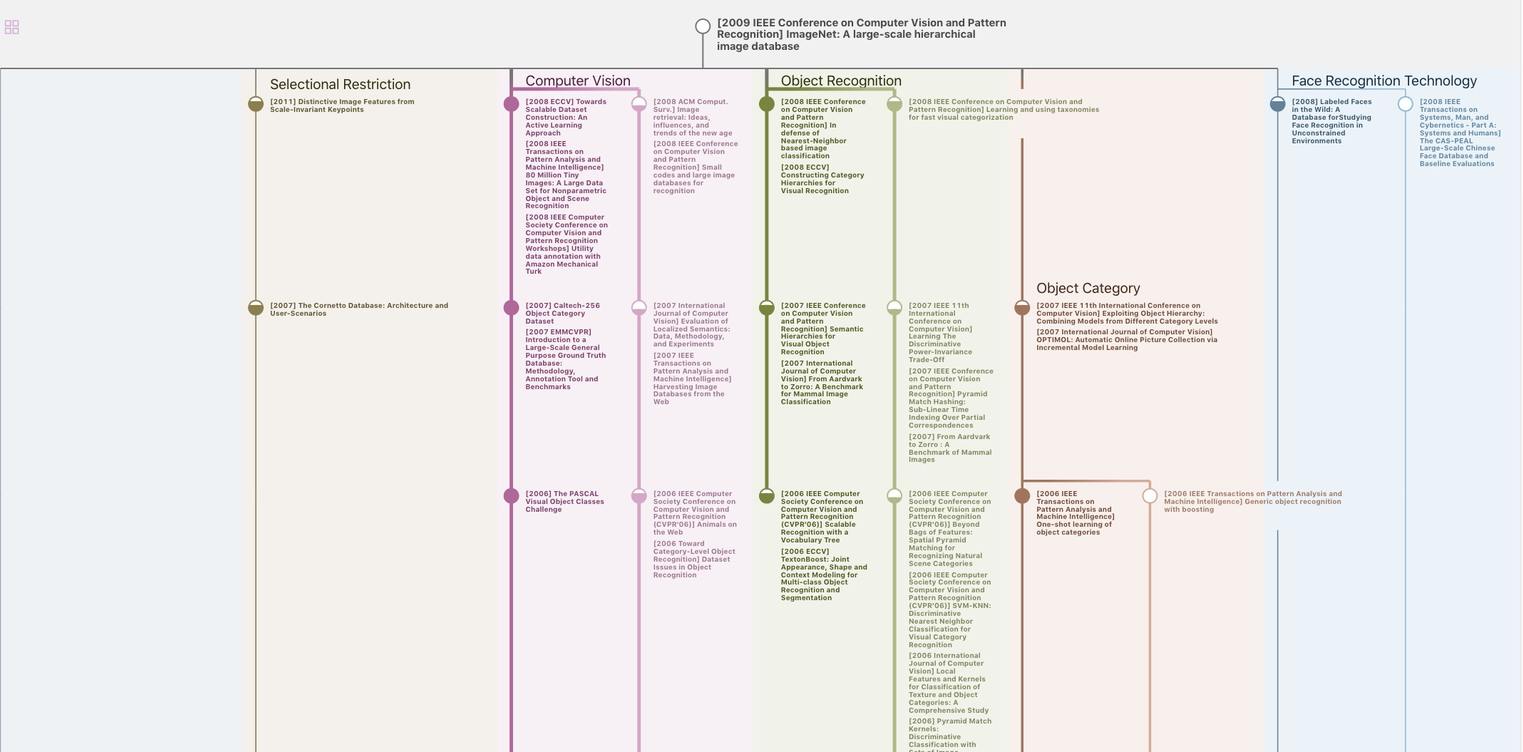
生成溯源树,研究论文发展脉络
Chat Paper
正在生成论文摘要