Fabric Defects Detection based on Multi-Sources Features Fusion.
ICCPR(2019)
摘要
For fabric object inspection, the traditional approaches (e.g., Low rank approximation and sparse representation) have achieved the excellent accuracy in some certain texture fabric, whereas some methods based on convolutional neural network have the advantage of higher efficiency and prime accuracy with various texture fabric. To furthermore improve the detection accuracy, in this paper, we propose a novel defect model based on transform learning. In the process of model training, both the multiple layer features of the image and the useful information of the source model are fused to meliorate the availability. Additionally, a novel training model called Multiple Sources Features Fusion (MSFF) is presented, which solve the situation of limited negative samples are available and demand to obtain fleet and precise quantification automatically for fabric image assessment. In this paper, we address this question quantitatively by comparing the performances of MSFF detection based on feature transfer network and Object Detection Network (ODN). And our proposed method improves Average Precision (AP) by more 5.9% relative to other result on TILDA-achieving an AP of 93.9%, and achieving an AP of 98.8% on ZYFD datasets, and false positive rate (FP) of 0.2%. Experimental results demonstrate the good performance in the defect detection for patterned fabric and more complex warp-knitted fabric.
更多查看译文
关键词
features,detection,multi-sources
AI 理解论文
溯源树
样例
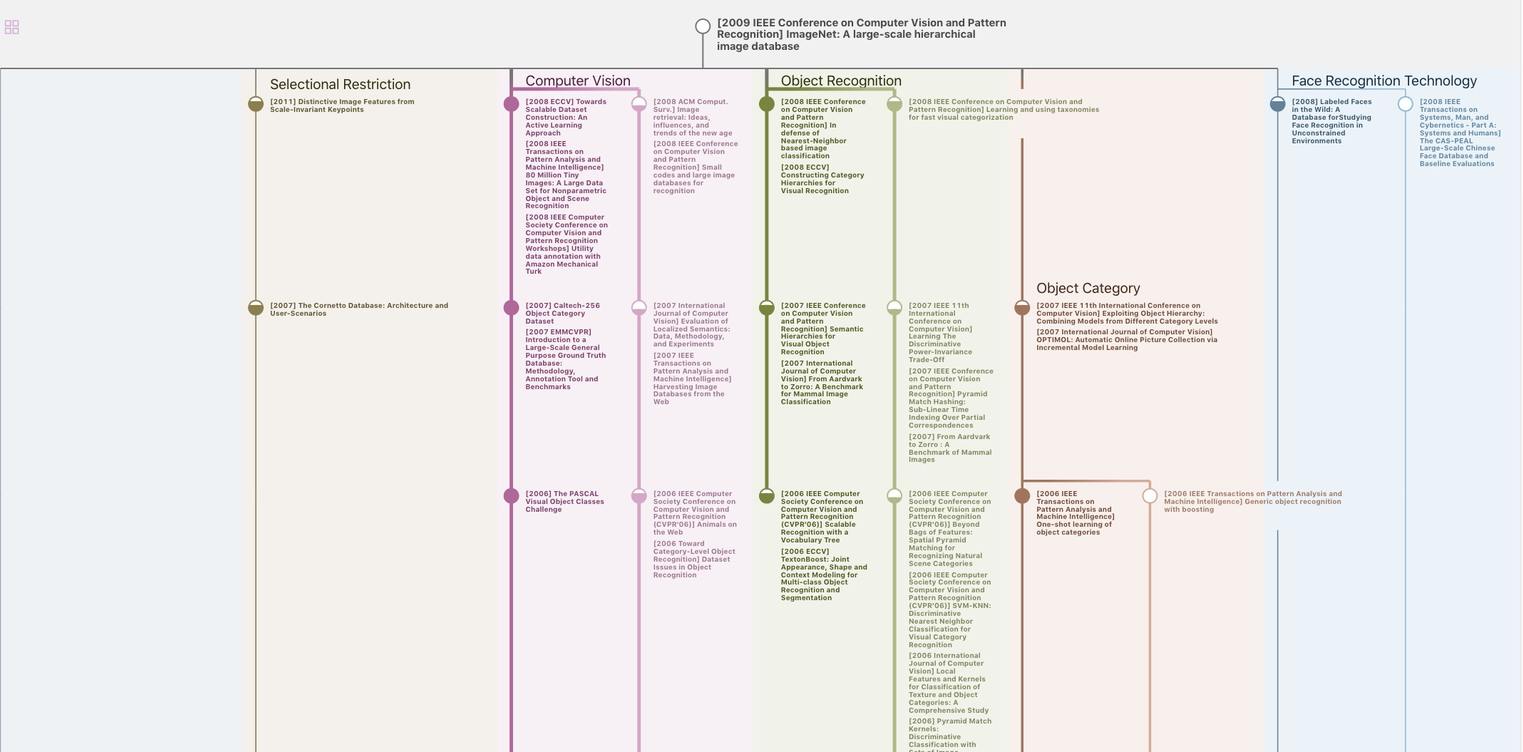
生成溯源树,研究论文发展脉络
Chat Paper
正在生成论文摘要