Adaptive Fractional Dilated Convolution Network for Image Aesthetics Assessment
CVPR(2020)
摘要
To leverage deep learning for image aesthetics assessment, one critical but unsolved issue is how to seamlessly incorporate the information of image aspect ratios to learn more robust models. In this paper, an adaptive fractional dilated convolution (AFDC), which is aspect-ratio-embedded, composition-preserving and parameter-free, is developed to tackle this issue natively in convolutional kernel level. Specifically, the fractional dilated kernel is adaptively constructed according to the image aspect ratios, where the interpolation of nearest two integer dilated kernels are used to cope with the misalignment of fractional sampling. Moreover, we provide a concise formulation for mini-batch training and utilize a grouping strategy to reduce computational overhead. As a result, it can be easily implemented by common deep learning libraries and plugged into popular CNN architectures in a computation-efficient manner. Our experimental results demonstrate that our proposed method achieves state-of-the-art performance on image aesthetics assessment over the AVA dataset.
更多查看译文
关键词
adaptive fractional dilated convolution network,image aesthetics assessment,image aspect ratios,convolutional kernel level,deep learning libraries,nearest two integer dilated kernels interpolation,CNN architectures
AI 理解论文
溯源树
样例
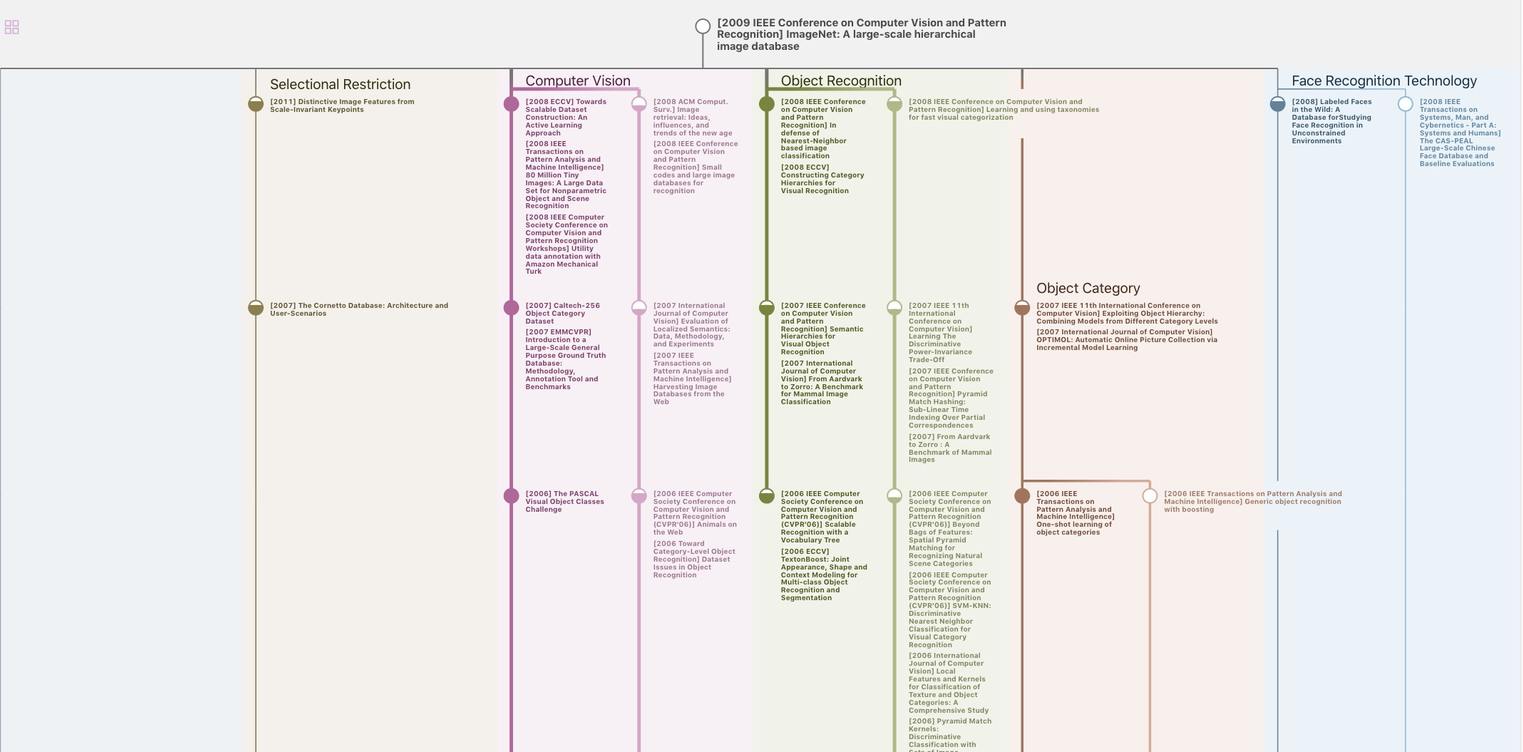
生成溯源树,研究论文发展脉络
Chat Paper
正在生成论文摘要