ML-EM algorithm with known continuous movement model
CoRR(2020)
摘要
In Positron Emission Tomography, movement leads to blurry reconstructions when not accounted for. Whether known a priori or estimated jointly to reconstruction, motion models are increasingly defined in continuum rather that in discrete, for example by means of diffeomorphisms. The present work provides both a statistical and functional analytic framework suitable for handling such models. It is based on time-space Poisson point processes as well as regarding images as measures, and allows to compute the maximum likelihood problem for line-of-response data with a known movement model. Solving the resulting optimisation problem, we derive an Maximum Likelihood Expectation Maximisation (ML-EM) type algorithm which recovers the classical ML-EM algorithm as a particular case for a static phantom. The algorithm is proved to be monotone and convergent in the low-noise regime. Simulations confirm that it correctly removes the blur that would have occurred if movement were neglected.
更多查看译文
关键词
continuous movement model,algorithm
AI 理解论文
溯源树
样例
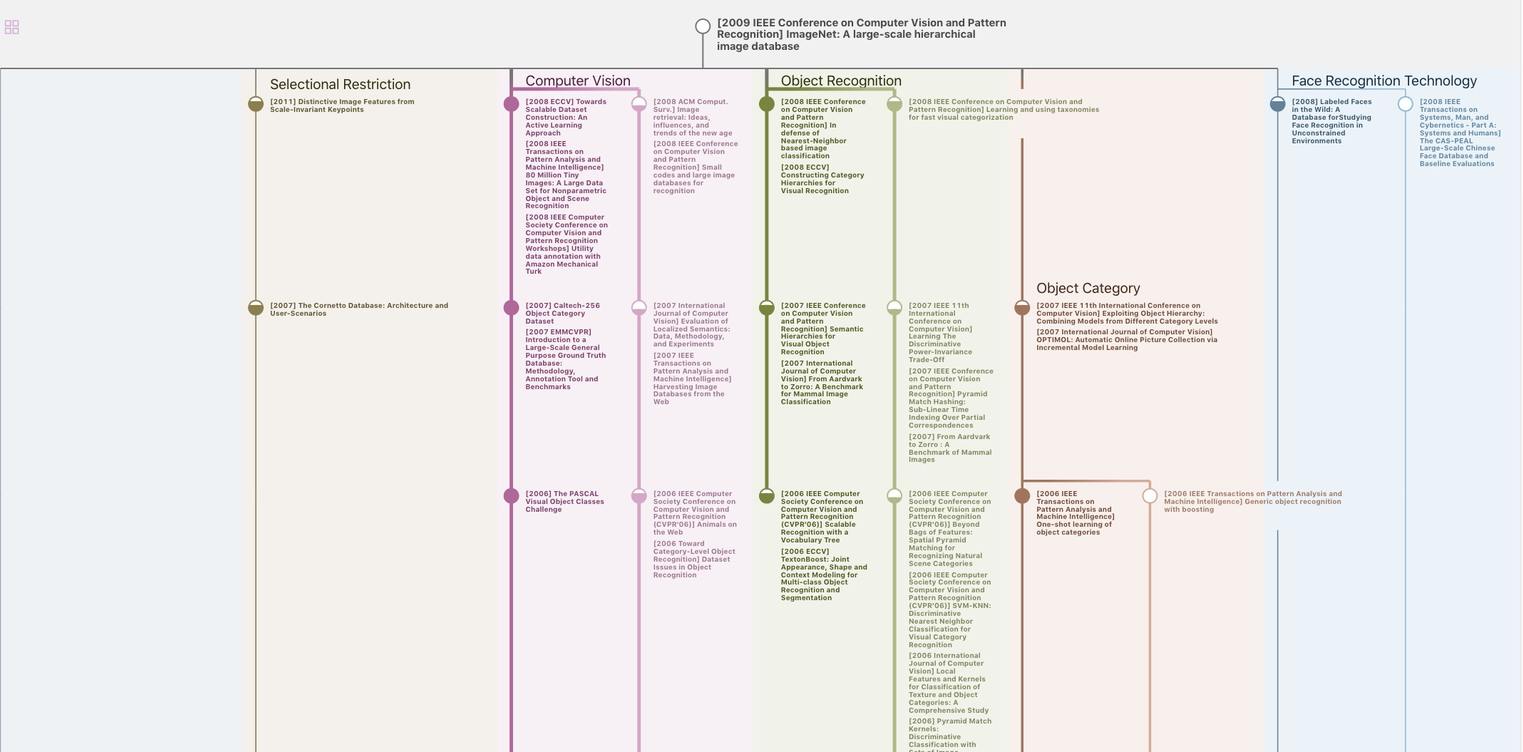
生成溯源树,研究论文发展脉络
Chat Paper
正在生成论文摘要