LU-Net: a multi-task network to improve the robustness of segmentation of left ventriclular structures by deep learning in 2D echocardiography
arxiv(2020)
摘要
Segmentation of cardiac structures is one of the fundamental steps to estimate volumetric indices of the heart. This step is still performed semi-automatically in clinical routine, and is thus prone to inter- and intra-observer variability. Recent studies have shown that deep learning has the potential to perform fully automatic segmentation. However, the current best solutions still suffer from a lack of robustness. In this work, we introduce an end-to-end multi-task network designed to improve the overall accuracy of cardiac segmentation while enhancing the estimation of clinical indices and reducing the number of outliers. Results obtained on a large open access dataset show that our method outperforms the current best performing deep learning solution and achieved an overall segmentation accuracy lower than the intra-observer variability for the epicardial border (i.e. on average a mean absolute error of 1.5mm and a Hausdorff distance of 5.1mm) with 11% of outliers. Moreover, we demonstrate that our method can closely reproduce the expert analysis for the end-diastolic and end-systolic left ventricular volumes, with a mean correlation of 0.96 and a mean absolute error of 7.6ml. Concerning the ejection fraction of the left ventricle, results are more contrasted with a mean correlation coefficient of 0.83 and an absolute mean error of 5.0%, producing scores that are slightly below the intra-observer margin. Based on this observation, areas for improvement are suggested.
更多查看译文
关键词
ventriclular structures,2d echocardiography,deep learning,segmentation,lu-net,multi-task
AI 理解论文
溯源树
样例
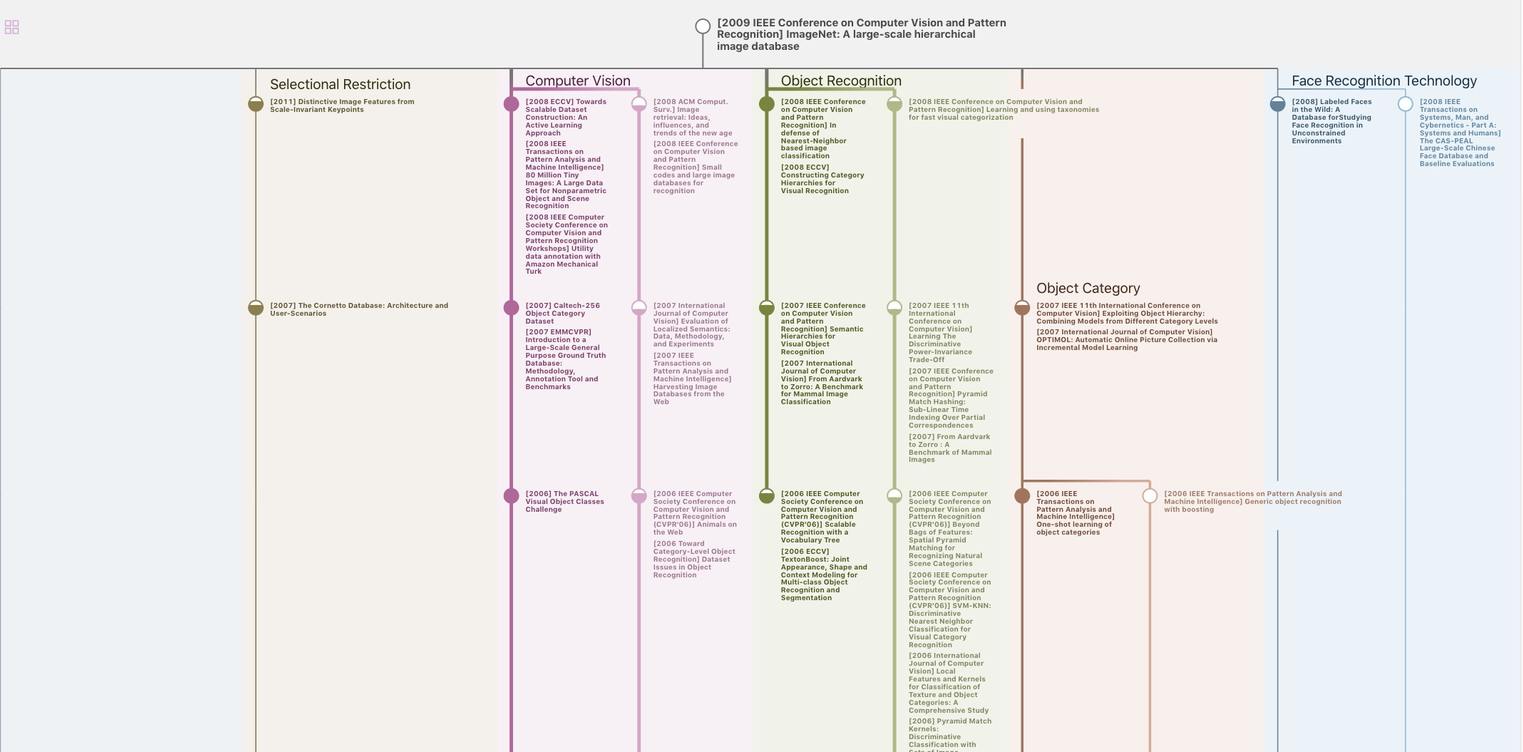
生成溯源树,研究论文发展脉络
Chat Paper
正在生成论文摘要