The equivalence between Stein variational gradient descent and black-box variational inference
international conference on learning representations(2020)
摘要
We formalize an equivalence between two popular methods for Bayesian inference: Stein variational gradient descent (SVGD) and black-box variational inference (BBVI). In particular, we show that BBVI corresponds precisely to SVGD when the kernel is the neural tangent kernel. Furthermore, we interpret SVGD and BBVI as kernel gradient flows; we do this by leveraging the recent perspective that views SVGD as a gradient flow in the space of probability distributions and showing that BBVI naturally motivates a Riemannian structure on that space. We observe that kernel gradient flow also describes dynamics found in the training of generative adversarial networks (GANs). This work thereby unifies several existing techniques in variational inference and generative modeling and identifies the kernel as a fundamental object governing the behavior of these algorithms, motivating deeper analysis of its properties.
更多查看译文
关键词
variational gradient descent,stein,black-box
black-box
AI 理解论文
溯源树
样例
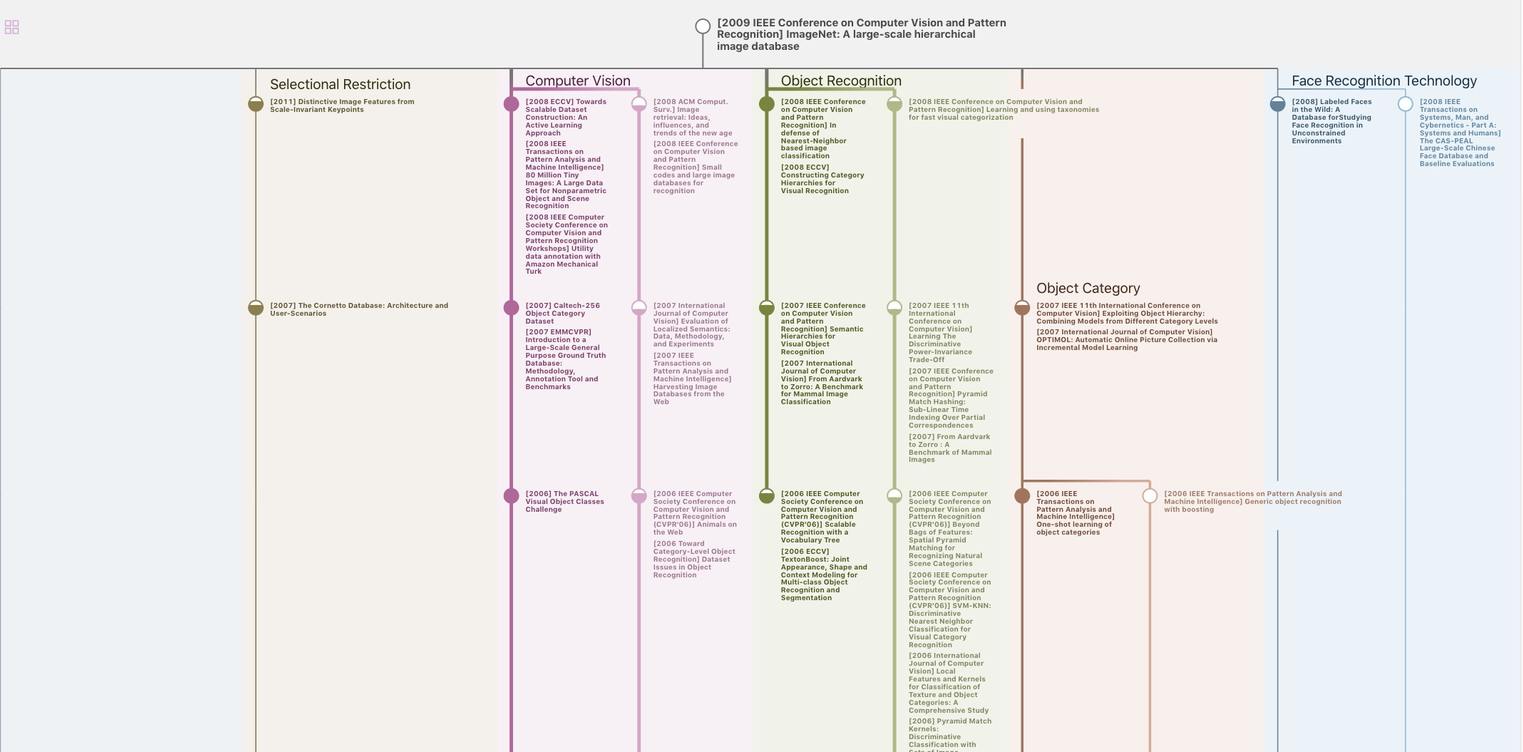
生成溯源树,研究论文发展脉络
Chat Paper
正在生成论文摘要