MAGSAC++, a fast, reliable and accurate robust estimator.
CoRR(2019)
摘要
A new method for robust estimation, MAGSAC++
1
, is proposed. It introduces a new model quality (scoring) function that does not require the inlier-outlier decision, and a novel marginalization procedure formulated as an M-estimation with a novel class of M-estimators (a robust kernel) solved by an iteratively re-weighted least squares procedure. We also propose a new sampler, Progressive NAPSAC, for RANSAC-like robust estimators. Exploiting the fact that nearby points often originate from the same model in real-world data, it finds local structures earlier than global samplers. The progressive transition from local to global sampling does not suffer from the weaknesses of purely localized samplers. On six publicly available realworld datasets for homography and fundamental matrix fitting, MAGSAC++ produces results superior to the state-of-the-art robust methods. It is faster, more geometrically accurate and fails less often.
更多查看译文
关键词
homography applications,fundamental matrix fitting,iteratively reweighted least squares procedure,M-estimation,scoring function,purely localized samplers,global samplers,RANSAC-like robust estimators,progressive NAPSAC,robust kernel,marginalization procedure,model quality function,accurate robust estimator,MAGSAC++
AI 理解论文
溯源树
样例
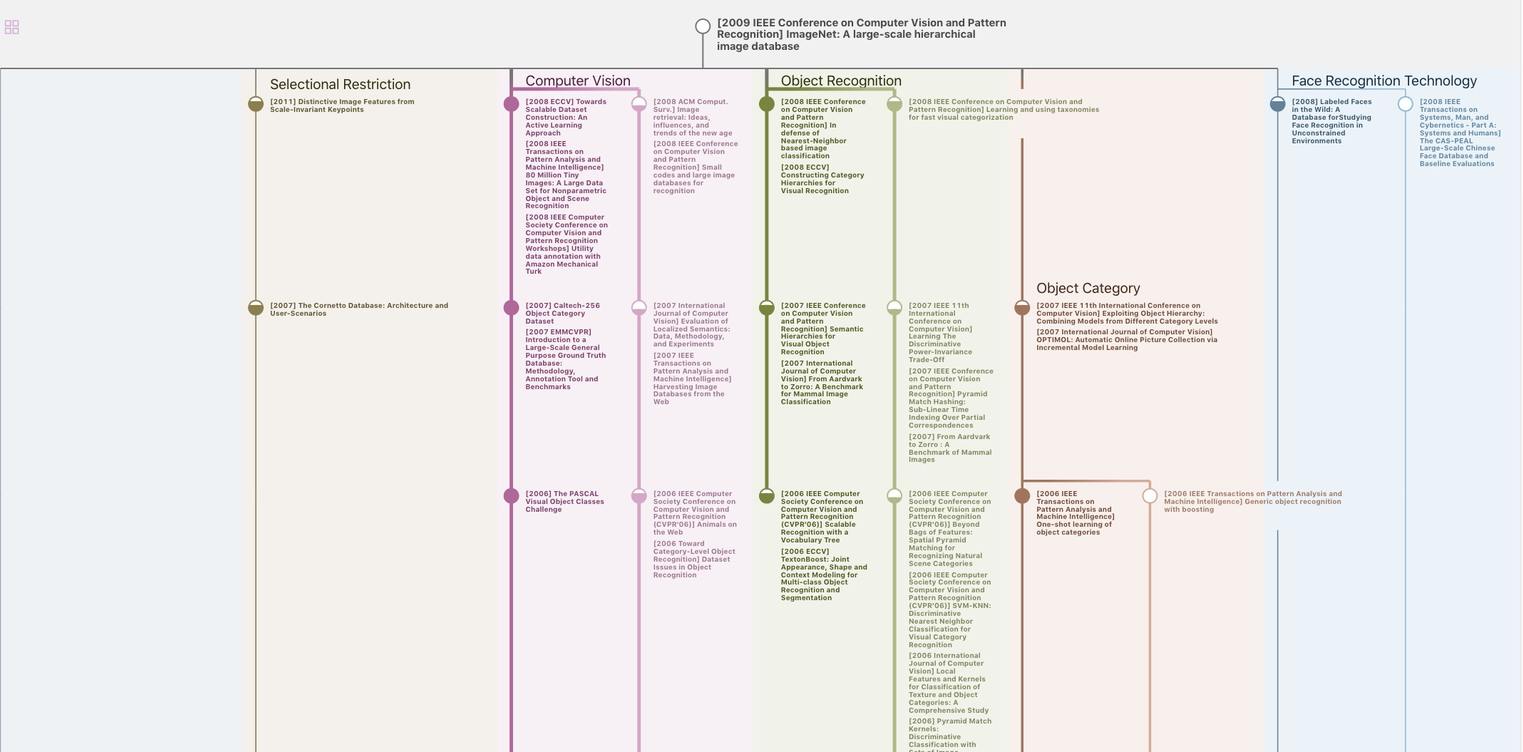
生成溯源树,研究论文发展脉络
Chat Paper
正在生成论文摘要