Hyperspectral Image Denoising via Combined Non-Local Self-Similarity and Local Low-Rank Regularization
IEEE ACCESS(2020)
Abstract
Hyperspectral images (HSIs) are usually corrupted by various noises during the image acquisition process, e.g., Gaussian noise, impulse noise, stripes, deadlines and many others. Such complex noise severely degrades the data quality, reduces the interpretation accuracy of HSIs, and restricts the subsequent HSI applications. In this paper, a spatial non-local and local rank-constrained low-rank regularized Plug-and-Play (NLRPnP) model is presented for mixed noise removal in HSIs. Specifically, we first divide HSIs into local overlapping patches. Local rank-constrained low-rank matrix recovery is adopted to effectively separate the low-rank clean HSI patches from the sparse noise and a part of Gaussian noise, and to significantly preserve local structure and detail information in HSIs. Then the spatial non-local based denoiser is introduced to promote the non-local self-similarity and obviously depress the Gaussian noise. Without increasing the difficulty of solving optimization problems, we combine the local and non-local based methods into the Plug-and-Play framework, and develop an efficient algorithm for solving the proposed NLRPnP model by using the alternating direction method of multipliers method. Finally, several experiments are conducted in both simulated and real data conditions to illustrate the better performance of the proposed NLRPnP model than the existing state-of-the-art denoising models.
MoreTranslated text
Key words
Noise reduction,Data models,Tensile stress,Hyperspectral imaging,Gaussian noise,Image denoising,Sparse matrices,Hyperspectral images,denoising,plug-and-play framework,local low-rank matrix recovery,non-local regularization
AI Read Science
Must-Reading Tree
Example
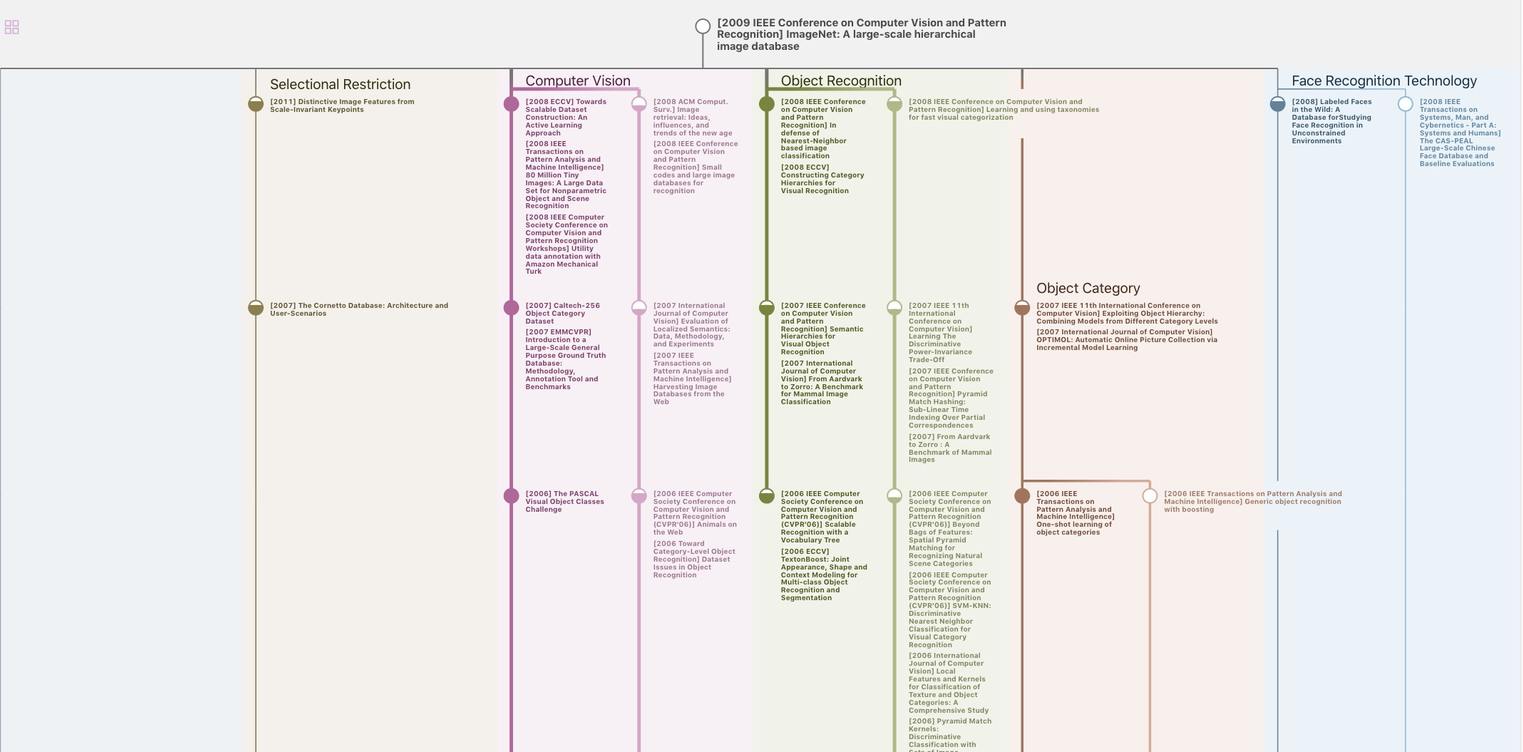
Generate MRT to find the research sequence of this paper
Chat Paper
Summary is being generated by the instructions you defined