An Enhanced Scale Robust Network for Crowd Counting.
IEEE ACCESS(2020)
摘要
The main challenge of crowd counting is the dramatic variations in scale and perspective. Most methods combine features of different receptive fields to deal with scale problems, but do not perform well on continuous scale variations in local areas. Moreover, they use only Euclidean loss which assumes independence of each pixel without considering local correlation in the density map. Therefore, we propose the enhanced scale robust network (ESRN) for accurate and efficient crowd counting. ESRN contains an embedded GAN module, which is followed by a well-designed enhancer. The discriminator guides the generator to strengthen the local correlation and create high-quality density maps, while the enhancer combines context information in different scales and varying among different sub-regions to further enhance the scale robustness of the network. The embedded GAN module is jointly trained with the enhancer. Extensive experimental results on three challenging datasets well demonstrate the effectiveness of our method.
更多查看译文
关键词
Generators,Gallium nitride,Robustness,Feature extraction,Convolution,Correlation,Generative adversarial networks,Crowd counting,local correlation,embedded GAN module,scale robustness,enhancer
AI 理解论文
溯源树
样例
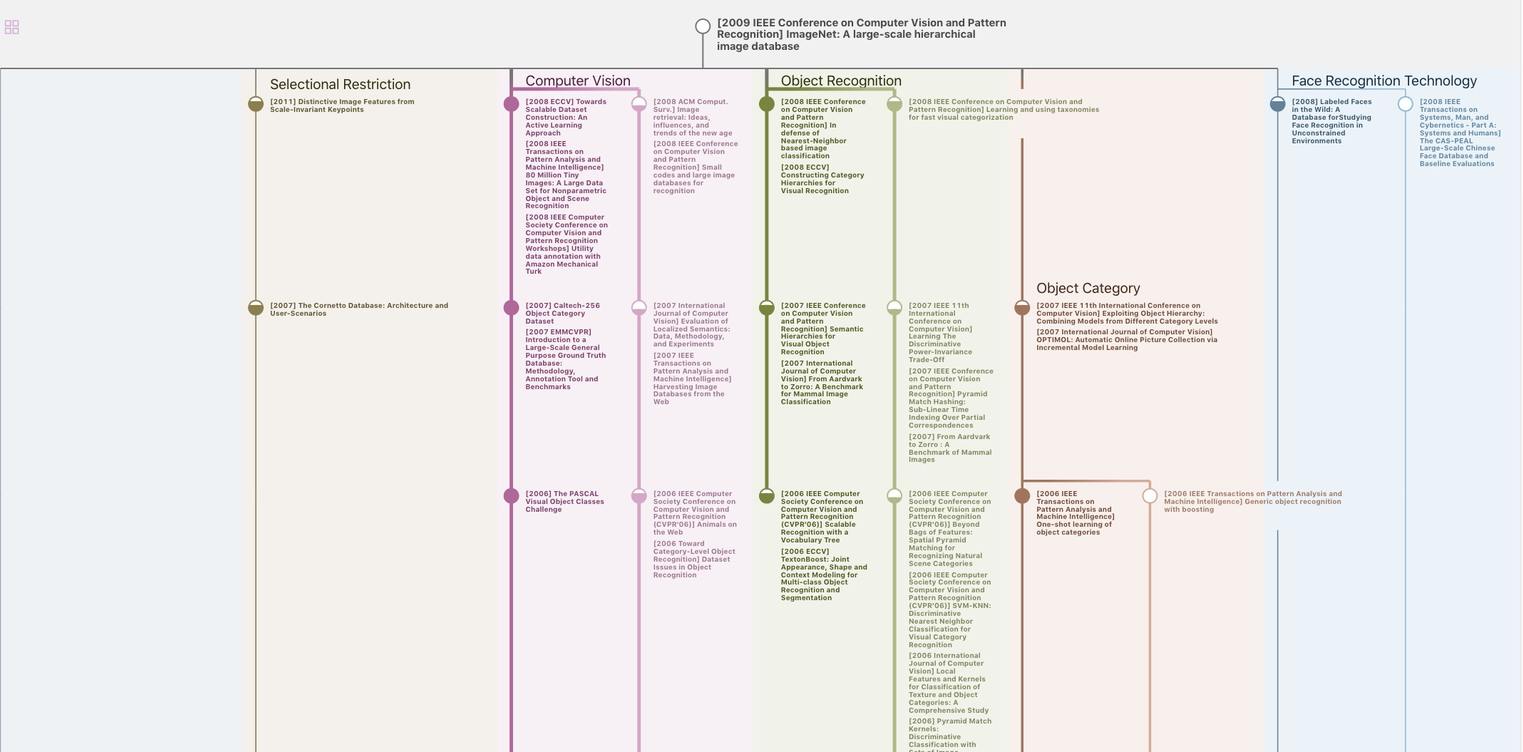
生成溯源树,研究论文发展脉络
Chat Paper
正在生成论文摘要