Segmentation of Cell Nuclei in Fluorescence Microscopy Images Using Deep Learning.
IbPRIA (1)(2019)
摘要
Cell nuclei segmentation is important for several applications, such as the detection of cancerous cells and cell cycle staging. The main challenges and difficulties, associated with this task, arise due to the presence of overlapping nuclei, image intensity inhomogeneities and image noise. Several classical methods have been proposed for cell nuclei segmentation. However, they depend strongly on manual setting of parameters and they are sensitive to noise. Recently, deep learning is becoming state-of-the-art, due to its enhanced performance in many tasks of computer vision, such as object detection, classification and segmentation. Deep learning models are robust to the presence of noise and are able to automatically extract meaningful features from the image. Although deep learning models perform significantly better than the traditional methods, they are computationally more expensive. In this paper we present a computationally efficient approach for high throughput nuclei segmentation based on deep learning. Our approach combines the object detection capability of Fast YOLO with the segmentation ability of U-Net. We applied our method to 2D fluorescence microscopy images with DAPI stained nuclei. Our results show that our method is competitive with Mask R-CNN, but significantly faster. In fact, with our method, an image of size 1388 x 1040 is segmented in approximately 1.6s which is about nine times faster than the Mask R-CNN (15.1 s). Additionally, our results show that the improvements in computational efficiency come at only a small cost in performance.
更多查看译文
关键词
Deep learning, Nuclei segmentation, Cell imaging
AI 理解论文
溯源树
样例
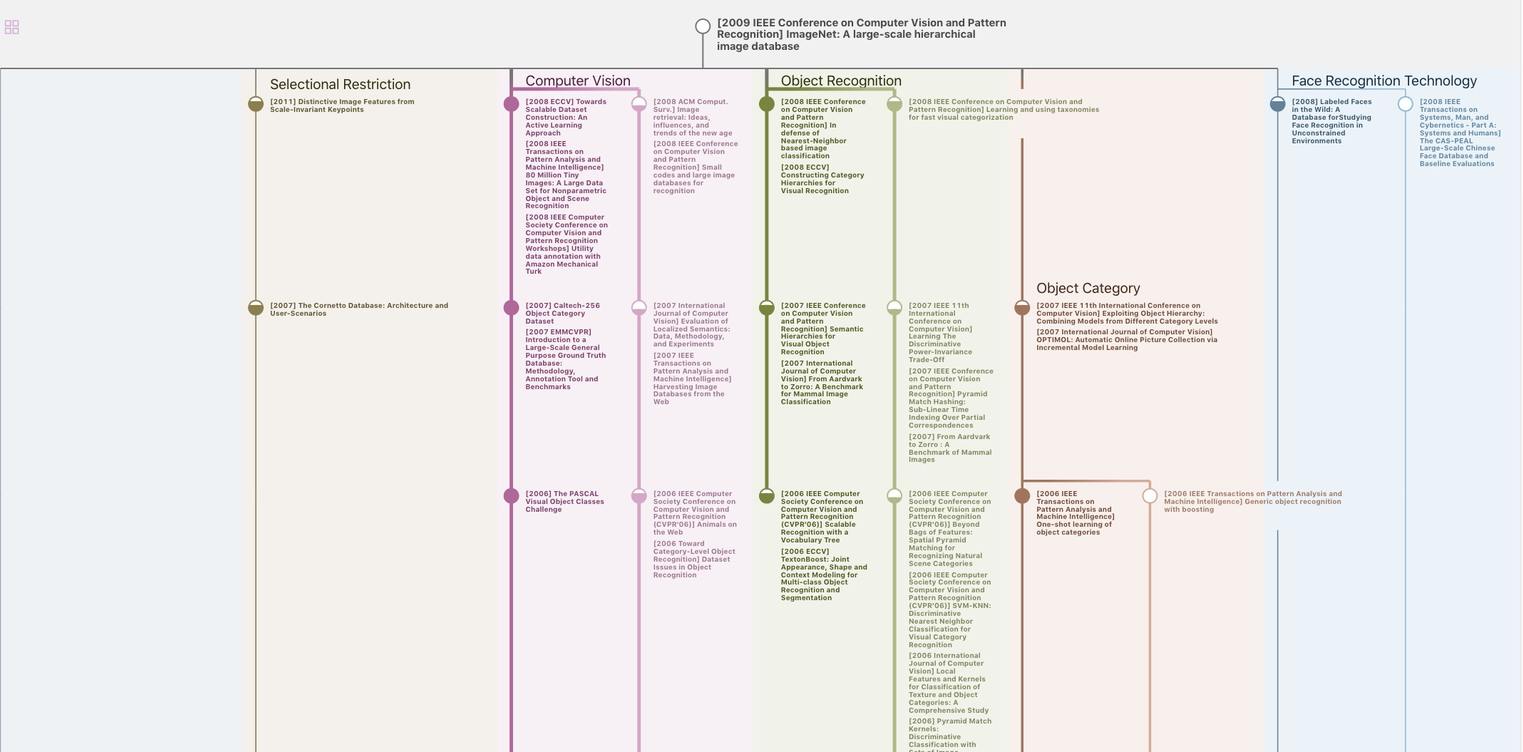
生成溯源树,研究论文发展脉络
Chat Paper
正在生成论文摘要