Activity Recognition For Indoor Fall Detection In 360-Degree Videos Using Deep Learning Techniques
PROCEEDINGS OF 3RD INTERNATIONAL CONFERENCE ON COMPUTER VISION AND IMAGE PROCESSING, CVIP 2018, VOL 2(2020)
摘要
Human activity recognition (HAR) targets the methodologies to recognize the different actions from a sequence of observations. Vision-based activity recognition is among the most popular unobtrusive technique for activity recognition. Caring for the elderly who are living alone from a remote location is one of the biggest challenges of modern human society and is an area of active research. The usage of smart homes with an increasing number of cameras in our daily environment provides the platform to use that technology for activity recognition also. The omnidirectional cameras can be utilized for fall detection activity which minimizes the requirement of multiple cameras for fall detection in an indoor living scenario. Consequently, two vision-based solutions have been proposed: one using convolutional neural networks in 3D-mode and another using a hybrid approach by combining convolutional neural networks and long short-term memory networks using 360-degree videos for human fall detection. An omnidirectional video dataset has been generated by recording a set of activities performed by different people as no such 360-degree video dataset is available in the public domain for human activity recognition. Both, the models provide fall detection accuracy of more than 90% for omnidirectional videos and can be used for developing a fall detection system for indoor health care.
更多查看译文
关键词
Activity recognition, Omnidirectional video, 3D convolutional networks, Long short-term memory networks, Fall activity, Daily activity
AI 理解论文
溯源树
样例
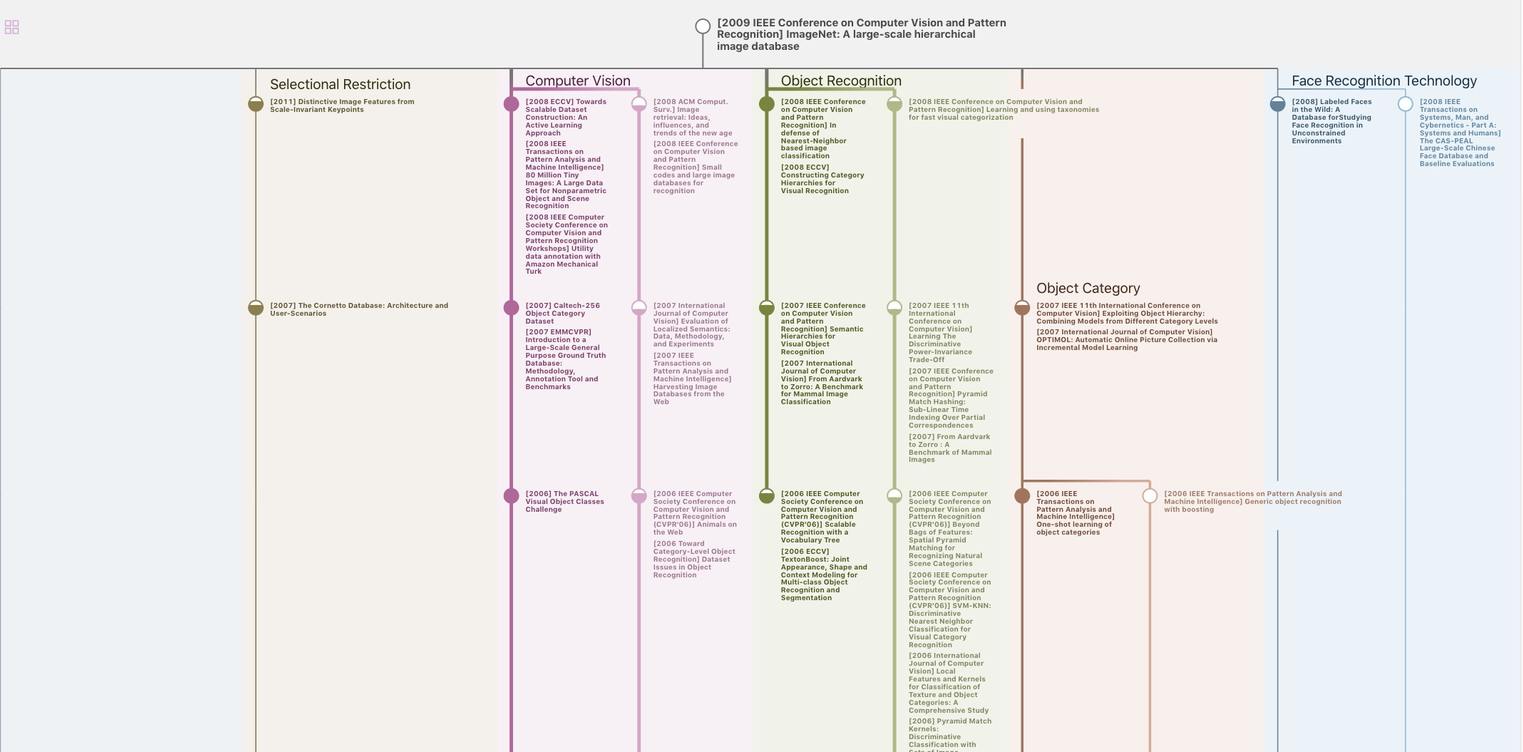
生成溯源树,研究论文发展脉络
Chat Paper
正在生成论文摘要