A Segment Level Approach to Speech Emotion Recognition Using Transfer Learning.
ACPR (2)(2019)
摘要
Speech emotion recognition (SER) is a non-trivial task considering that the very definition of emotion is ambiguous. In this paper, we propose a speech emotion recognition system that predicts emotions for multiple segments of a single audio clip unlike the conventional emotion recognition models that predict the emotion of an entire audio clip directly. The proposed system consists of a pre-trained deep convolutional neural network (CNN) followed by a single layered neural network which predicts the emotion classes of the audio segments. The predictions for the individual segments are finally combined to predict the emotion of a particular clip. We define several new types of accuracies while evaluating the performance of the proposed model. The proposed model attains an accuracy of 68.7% surpassing the current state-of-the-art models in classifying the data into one of the four emotional classes (angry, happy, sad and neutral) when trained and evaluated on IEMOCAP audio-only dataset.
更多查看译文
关键词
Emotion recognition, Affective computing, Deep learning, Mel spectrograms, Computational paralinguistics
AI 理解论文
溯源树
样例
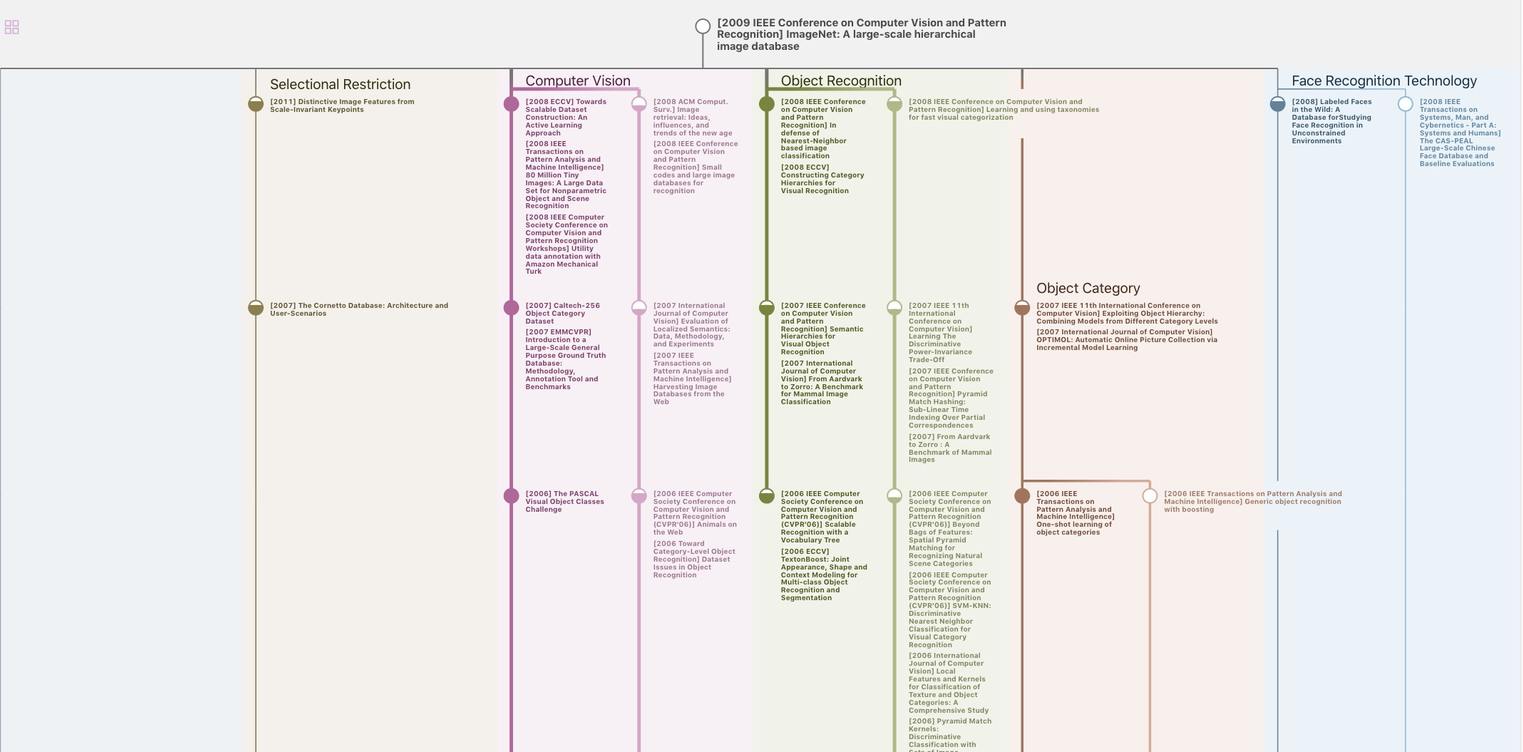
生成溯源树,研究论文发展脉络
Chat Paper
正在生成论文摘要