WRL: A Combined Model for Short-Term Load Forecasting.
APWeb/WAIM (1)(2019)
摘要
Load forecasting plays a vital role in economic construction and national security. The accuracy of short-term load forecasting will directly affect the quality of power supply and user experience, and will indirectly affect the stability and safety of the power system operation. In this paper, we present a novel short-term load forecasting model, which combines influencing factors analysis, Wavelet Decomposition feature extraction, Radial Basis Function (RBF) neural networks and Bidirectional Long Short-Term Memory (Bi-LSTM) networks (WRL below). The model uses wavelet decomposition to extract the main features of load data, analyzes its correlation with influencing factors, and then constructs corresponding adjustment factors. The RBF neural networks are used to forecast the feature subsequence related to external factors. Other subsequences are input into Bidirectional LSTM networks to forecast future values. Finally, the forecasting results are obtained by wavelet inverse transform. Experiments show that the proposed short-term load forecasting method is effective and feasible.
更多查看译文
关键词
Load forecasting, Wavelet Decomposition, RBF neural networks, Bi-LSTM networks, Adjustment factors
AI 理解论文
溯源树
样例
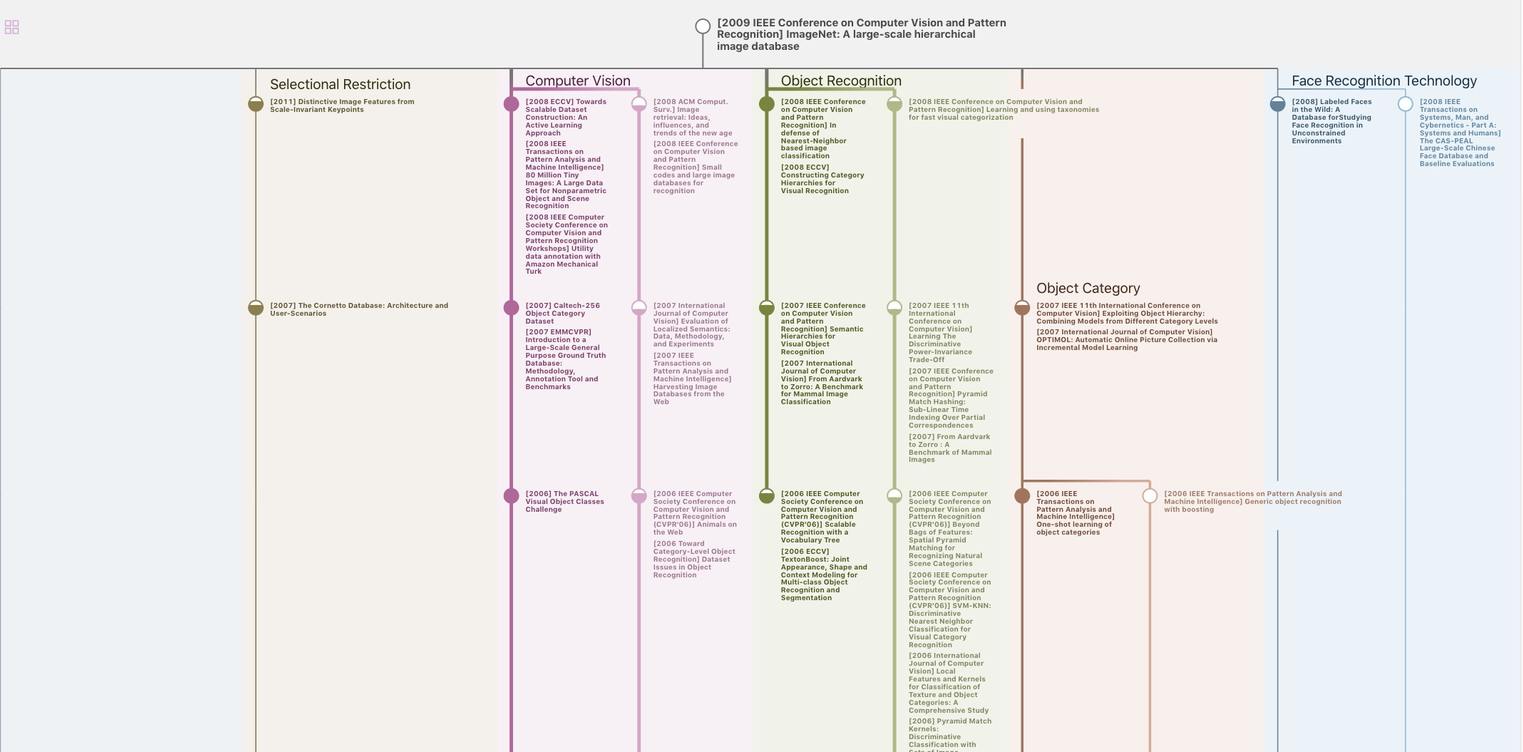
生成溯源树,研究论文发展脉络
Chat Paper
正在生成论文摘要